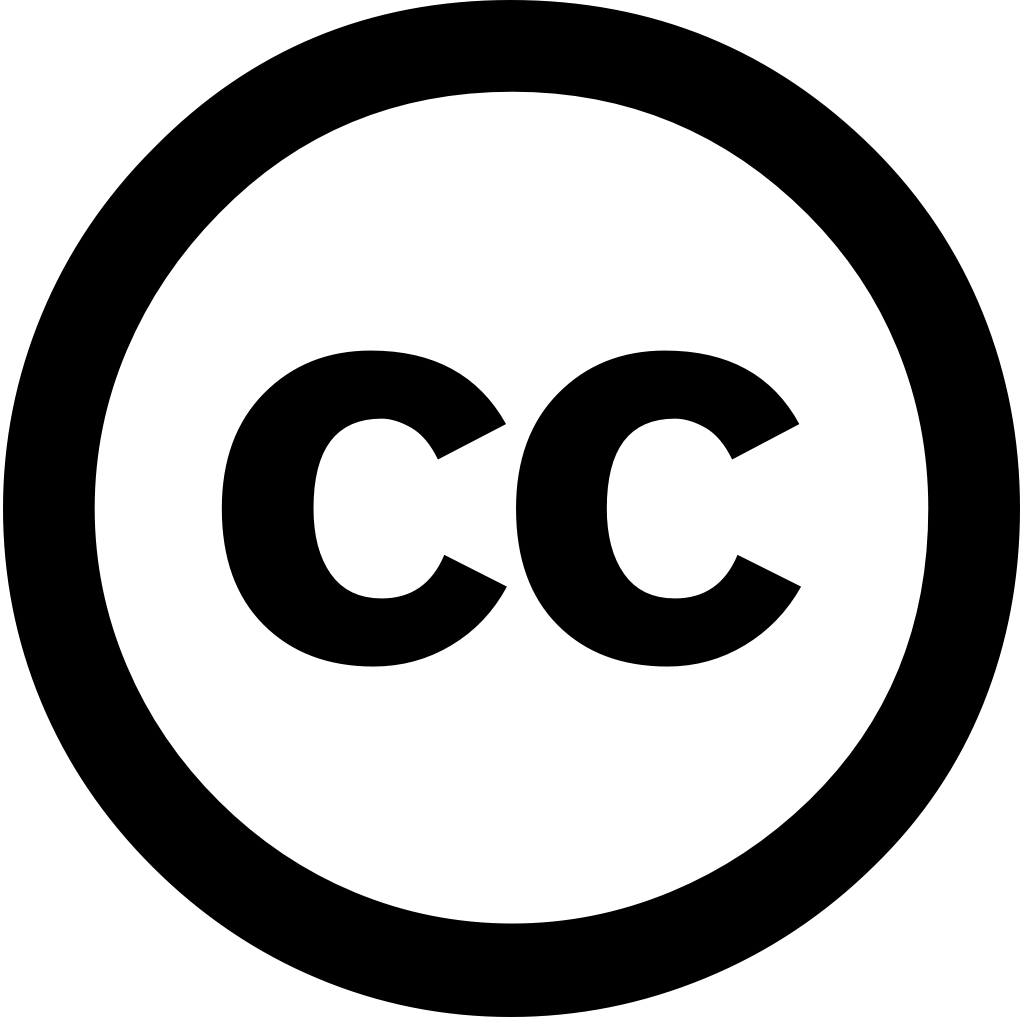
Computers, Journal Year: 2025, Volume and Issue: 14(4), P. 143 - 143
Published: April 10, 2025
Artificial Intelligence (AI) methodologies have profoundly influenced healthcare research, particularly in chronic disease management and public health. This paper provides a comprehensive state-of-the-art review of AI’s applications across diabetes, cancer, epidemiology, mortality prediction. The analysis highlights advancements machine learning (ML), deep (DL), natural language processing (NLP) that enable robust predictive models decision support systems, leading to significant clinical health outcomes. study examines modeling, pattern recognition, applications, addressing their respective challenges potential real-world settings. Emphasis is placed on the emerging role explainable AI (XAI), multimodal data fusion, privacy-preserving techniques such as federated learning, which aim enhance interpretability, robustness, ethical compliance. underscores vital interdisciplinary collaboration adaptive systems creating resilient, scalable, patient-centric solutions.
Language: Английский