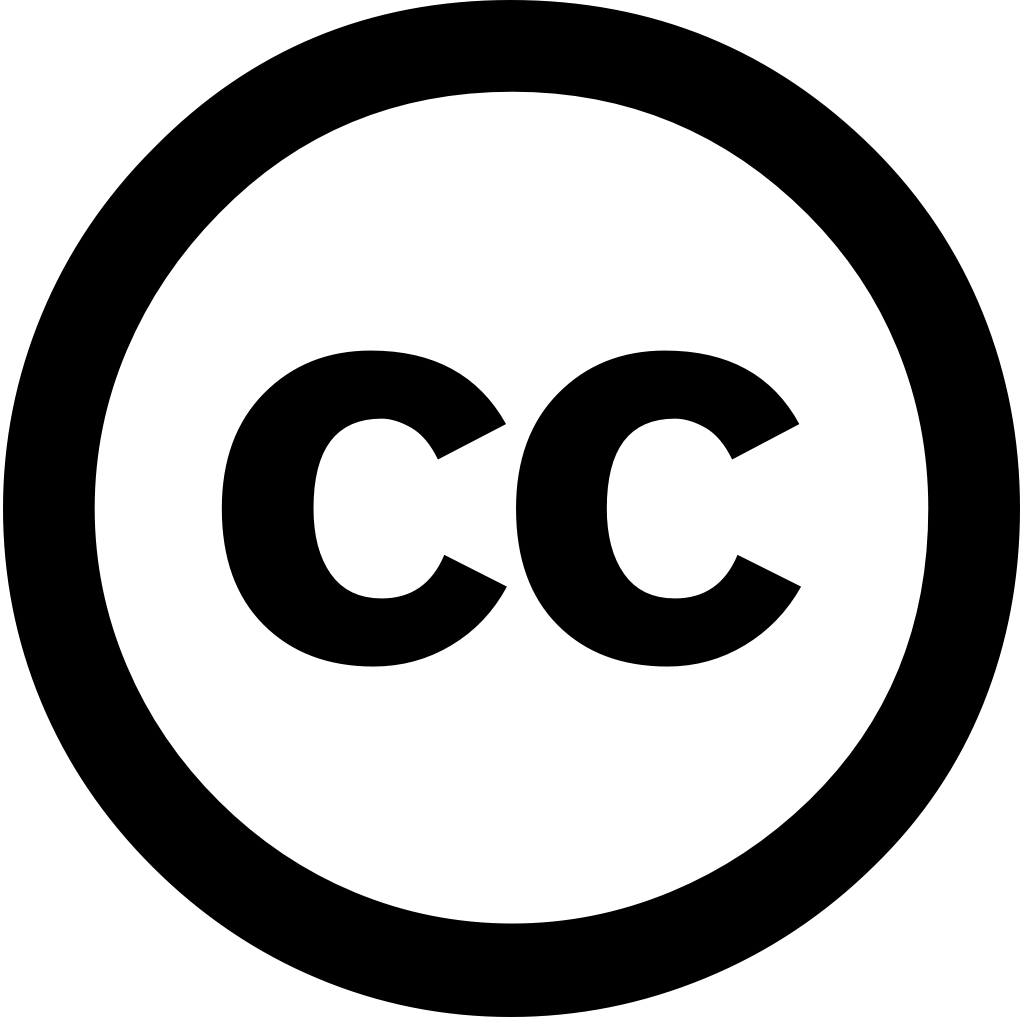
Applied Sciences, Journal Year: 2025, Volume and Issue: 15(9), P. 4756 - 4756
Published: April 25, 2025
The accurate evaluation of rock mass quality and competent roadway-support decision-making requires the rapid acquisition distribution structural planes in rocks. To address this need, a program was developed that uses deep learning to automatically recognize plane in-borehole images. First, borehole images from 30 mines China were collected during field tests, categorized into five types. Second, Coral architecture based on convolutional neural network (CNN) established extract features classify therein. experimental results indicate CNN model classifies with an overall accuracy 86%. Validation tests applications demonstrated recognition accuracies ranging 0.76 1.0 compared manual markings, meeting engineering requirements. Finally, proposed method, intelligent system surrounding fracture developed. Engineering application cases are presented discussed demonstrate method confirm approach. Compared traditional classification methods, rapidly recognizes at low cost, precision, non-destructive automated manner.
Language: Английский