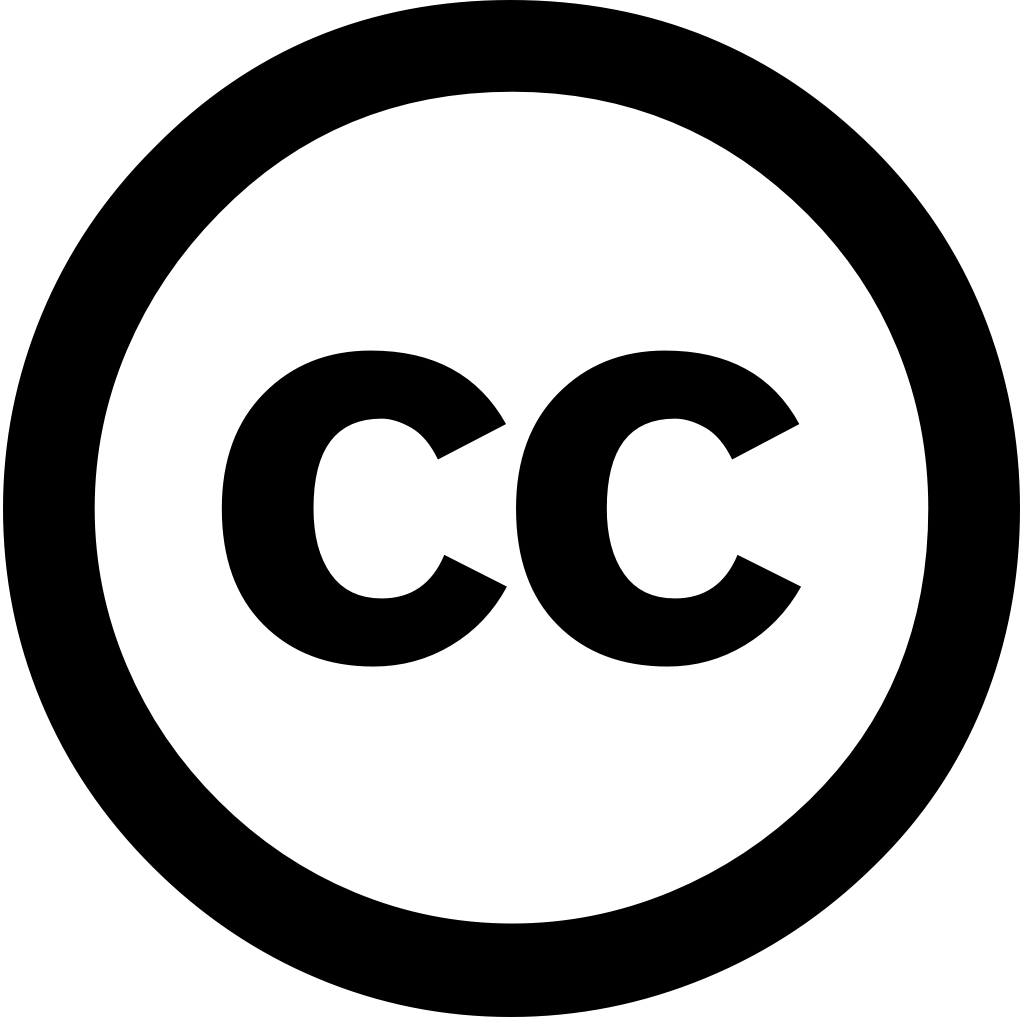
bioRxiv (Cold Spring Harbor Laboratory), Journal Year: 2024, Volume and Issue: unknown
Published: Oct. 22, 2024
Abstract Complex neural systems can display structured emergent dynamics. Capturing this structure remains a significant scientific challenge. Using information theory, we apply Dynamical Independence (DI) to uncover the dynamical in minimal 5-node biophysical model, shaped by interplay of two key aspects brain organisation: integration and segregation. In our study, functional within model is modulated global coupling parameter, while segregation influenced adding noise, which counteracts coupling. DI defines dimensionally-reduced macroscopic variable (e.g., coarse-graining) as extent that it behaves an independent process, distinct from micro-level We measure dependence (a departure independence) for variables across spatial scales. Our results indicate degree emergence relatively minimised at balanced points maximised extremes. Additionally, method identifies dynamics are localised microlevel nodes, thereby elucidating through relationship between microscopic processes. find deviation point less localised, more distributed identified DI. This finding suggests balance associated with lower levels (higher dependence), may be crucial sustaining coherent, structures. work also provides complete computational implementation identification could applied both silico vivo. Author summary Understanding how complex give rise patterns central challenge neuroscience. Emergence, where structures appear underlying interactions, plays role function, yet identifying specific involved elusive. Traditionally, methods have quantified but struggled pinpoint itself. develop method, based on quantity called (DI), simultaneously captures reveals neurophysiological data. explore segregation—two organisational principles brain—affects show finely system produces highly coherent structures, extreme deviations lead distributed, framework theoretical models potentially empirical data, advancing understanding brain’s organisation higher-order
Language: Английский