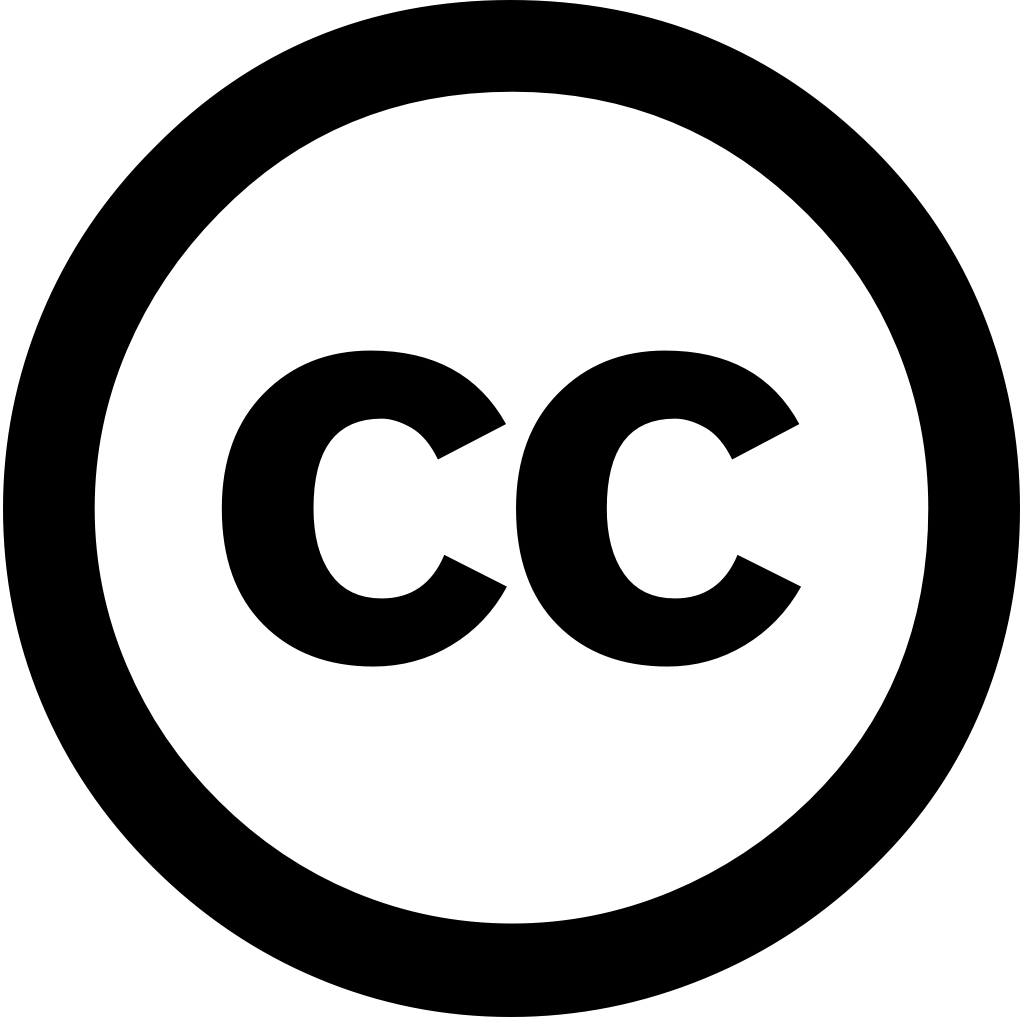
Frontiers in Big Data, Journal Year: 2025, Volume and Issue: 7
Published: Jan. 17, 2025
Leukemia is the 11th most prevalent type of cancer worldwide, with acute myeloid leukemia (AML) being frequent malignant blood malignancy in adults. Microscopic tests are common methods for identifying subtypes. An automated optical image-processing system using artificial intelligence (AI) has recently been applied to facilitate clinical decision-making. To evaluate performance all AI-based approaches detection and diagnosis (AML). Medical databases including PubMed, Web Science, Scopus were searched until December 2023. We used "metafor" "metagen" libraries R analyze different models studies. Accuracy sensitivity primary outcome measures. Ten studies included our review meta-analysis, conducted between 2016 Most deep-learning have utilized, convolutional neural networks (CNNs). The common- random-effects had accuracies 1.0000 [0.9999; 1.0001] 0.9557 [0.9312, 0.9802], respectively. random effects high values 0.8581, respectively, indicating that machine learning this study can accurately detect true-positive cases. Studies shown substantial variations accuracy sensitivity, as by Q I2 statistics. Our systematic meta-analysis found an overall AI correctly AML Future research should focus on unifying reporting assessment metrics diagnostics. https://www.crd.york.ac.uk/prospero/#recordDetails, CRD42024501980.
Language: Английский