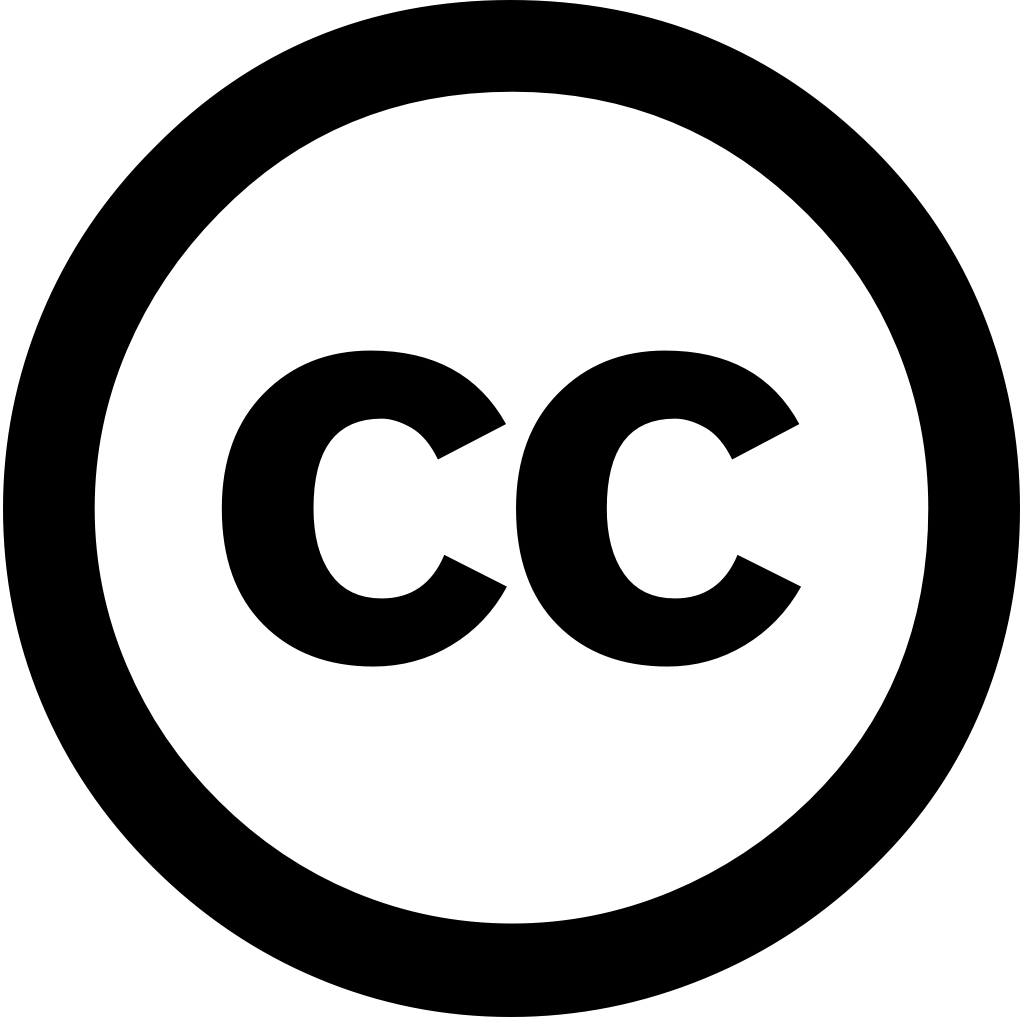
Agricultural Water Management, Journal Year: 2024, Volume and Issue: 307, P. 109253 - 109253
Published: Dec. 19, 2024
Language: Английский
Agricultural Water Management, Journal Year: 2024, Volume and Issue: 307, P. 109253 - 109253
Published: Dec. 19, 2024
Language: Английский
Agronomy, Journal Year: 2025, Volume and Issue: 15(4), P. 928 - 928
Published: April 10, 2025
Precision agriculture is necessary for dealing with problems like pest outbreaks, a lack of water, and declining crop health. Manual inspections broad-spectrum pesticide application are inefficient, time-consuming, dangerous. New drone photography IoT sensors offer quick, high-resolution, multimodal agricultural data collecting. Regional diversity, heterogeneity, privacy make it hard to conclude these data. This study proposes lightweight, hybrid deep learning architecture called federated LeViT-ResUNet that combines the spatial efficiency LeViT transformers ResUNet’s exact pixel-level segmentation address issues. The system uses multispectral footage sensor identify real-time insect hotspots, health, yield prediction. dynamic relevance sparsity-based feature selector (DRS-FS) improves ranking reduces redundancy. Spectral normalization, spatial–temporal alignment, dimensionality reduction provide reliable input representation. Unlike centralized models, our platform trains over-dispersed client datasets using preserve capture regional trends. A huge, open-access dataset from varied environmental circumstances was used simulation experiments. suggested approach on conventional models ResNet, DenseNet, vision transformer 98.9% classification accuracy 99.3% AUC. scalable sustainable privacy-preserving precision because its high generalization, low latency, communication efficiency. lays groundwork real-time, intelligent monitoring systems in diverse, resource-constrained farming situations.
Language: Английский
Citations
0Sensors, Journal Year: 2024, Volume and Issue: 24(24), P. 8172 - 8172
Published: Dec. 21, 2024
Grapevines (Vitis vinifera L.) are one of the most economically relevant crops worldwide, yet they highly vulnerable to various diseases, causing substantial economic losses for winegrowers. This systematic review evaluates application remote sensing and proximal tools vineyard disease detection, addressing current capabilities, gaps, future directions in sensor-based field monitoring grapevine diseases. The covers 104 studies published between 2008 October 2024, identified through searches Scopus Web Science, conducted on 25 January updated 10 2024. included focused exclusively detection while excluded were not related did use or sensing, conditions. studied diseases include downy mildew, powdery Flavescence dorée, esca complex, rots, viral main sensors RGB, multispectral, hyperspectral sensors, spectroscopy. A trend recent research is integration artificial intelligence techniques, such as machine learning deep learning, improve accuracy. results demonstrate progress monitoring, with concentrating specific sensor platforms, methodological improvements. Future should focus standardizing methodologies, integrating multi-sensor data, validating approaches across diverse contexts commercial applicability sustainability, both environmental challenges.
Language: Английский
Citations
3Advances in Space Research, Journal Year: 2025, Volume and Issue: unknown
Published: Feb. 1, 2025
Language: Английский
Citations
0Agricultural Water Management, Journal Year: 2025, Volume and Issue: 312, P. 109407 - 109407
Published: March 9, 2025
Language: Английский
Citations
0Computers and Electronics in Agriculture, Journal Year: 2025, Volume and Issue: 235, P. 110368 - 110368
Published: April 4, 2025
Language: Английский
Citations
0Sensors, Journal Year: 2025, Volume and Issue: 25(8), P. 2345 - 2345
Published: April 8, 2025
Chlorophyll absorbs light energy and converts it into chemical energy, making a crucial biochemical parameter for monitoring vegetation health, detecting environmental stress, predicting physiological states. Accurate rapid estimation of canopy chlorophyll content is assessing dynamics, ecological changes, growth patterns. Remote sensing technology has become an indispensable tool since 2015, with more than 50 research papers published annually, contributing to substantial body case studies. This review discusses remote technologies currently used estimating content, focusing on four key aspects: the acquisition reference datasets, identification optimal spectral variables, selection models, analysis application scenarios. The results indicate that bands in visible red-edge regions (e.g., 530 nm, 670 705 nm) provide high prediction accuracy. Machine learning methods, such as random forest support vector regression, exhibit excellent performance, determination coefficients (R2) typically exceeding 0.9, although overfitting remains issue. Although radiative transfer models are slightly less accurate (R2 = 0.6–0.8), they greater interpretability. Hybrid integrating machine show strong potential balance accuracy generalizability. Future should improve model generalizability different types conditions integrate multi-source data spatial temporal resolution. Combining physical processing artificial intelligence, can scalability, cost-effectiveness, real-time capabilities.
Language: Английский
Citations
0Agricultural Water Management, Journal Year: 2024, Volume and Issue: 307, P. 109253 - 109253
Published: Dec. 19, 2024
Language: Английский
Citations
0