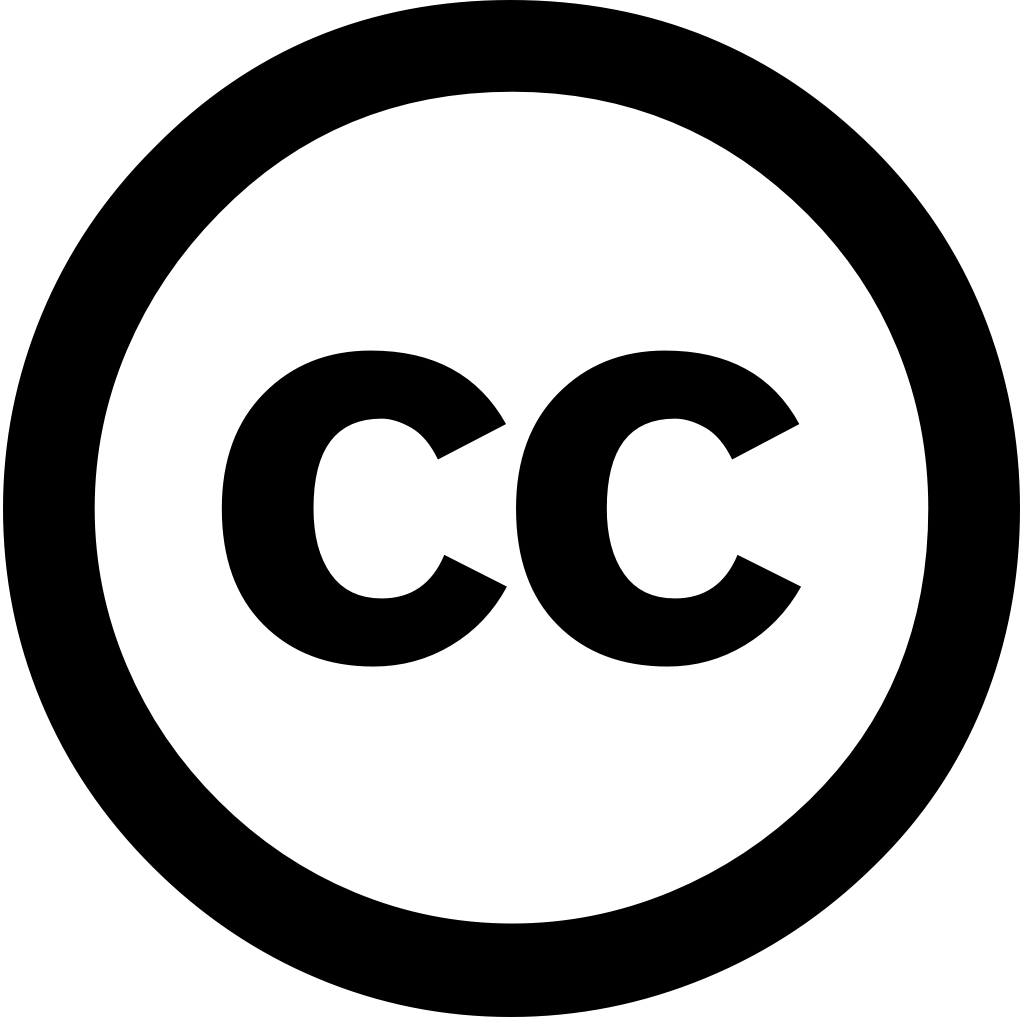
Computation, Journal Year: 2025, Volume and Issue: 13(3), P. 70 - 70
Published: March 8, 2025
The global COVID-19 pandemic has generated extensive datasets, providing opportunities to apply machine learning for diagnostic purposes. This study evaluates the performance of five supervised models—Random Forests (RFs), Artificial Neural Networks (ANNs), Support Vector Machines (SVMs), Logistic Regression (LR), and Decision Trees (DTs)—on a hospital-based dataset from Concepción Department in Paraguay. To address missing data, four imputation methods (Predictive Mean Matching via MICE, RF-based imputation, K-Nearest Neighbor, XGBoost-based imputation) were tested. Model was compared using metrics such as accuracy, AUC, F1-score, MCC across levels missingness. Overall, RF consistently achieved high accuracy AUC at highest missingness level, underscoring its robustness. In contrast, SVM often exhibited trade-off between specificity sensitivity. ANN DT showed moderate resilience, yet more prone shifts under certain approaches. These findings highlight RF’s adaptability different strategies, well importance selecting that minimize sensitivity–specificity trade-offs. By comparing multiple techniques models, this provides practical insights handling medical data resource-constrained settings underscores value robust ensemble reliable diagnostics.
Language: Английский