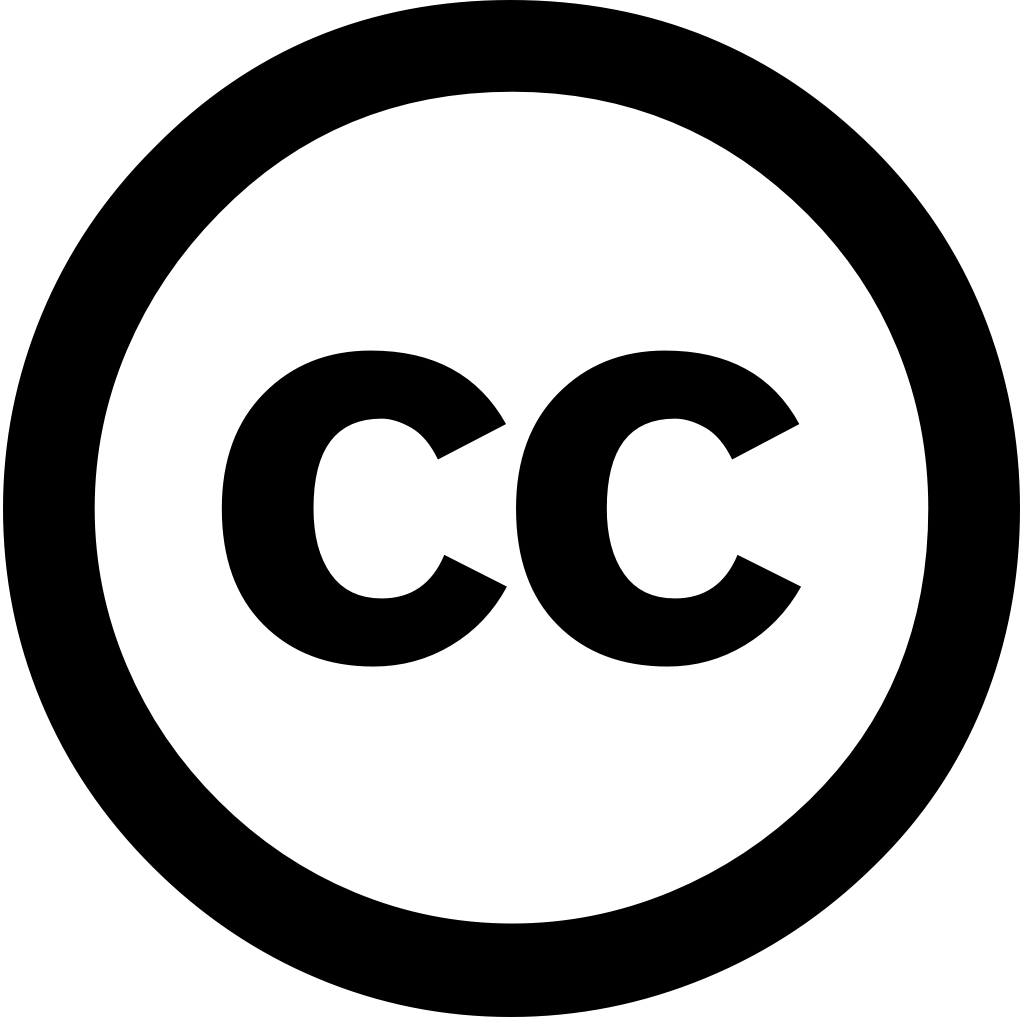
Life, Journal Year: 2025, Volume and Issue: 15(5), P. 745 - 745
Published: May 6, 2025
The integration of artificial intelligence and personalized medicine is transforming HIV management by enhancing diagnostics, treatment optimization, disease monitoring. Advances in machine learning, deep neural networks, multi-omics data analysis enable precise prognostication, tailored antiretroviral therapy, early detection drug resistance. AI-driven models analyze vast genomic, proteomic, clinical datasets to refine strategies, predict progression, pre-empt therapy failures. Additionally, AI-powered diagnostic tools, including learning imaging natural language processing, improve screening accuracy, particularly resource-limited settings. Despite these innovations, challenges such as privacy, algorithmic bias, the need for validation remain. Successful AI into care requires robust regulatory frameworks, interdisciplinary collaboration, equitable technology access. This review explores both potential limitations management, emphasizing ethical implementation expanded research maximize its impact. approaches hold great promise a more personalized, efficient, effective future care.
Language: Английский