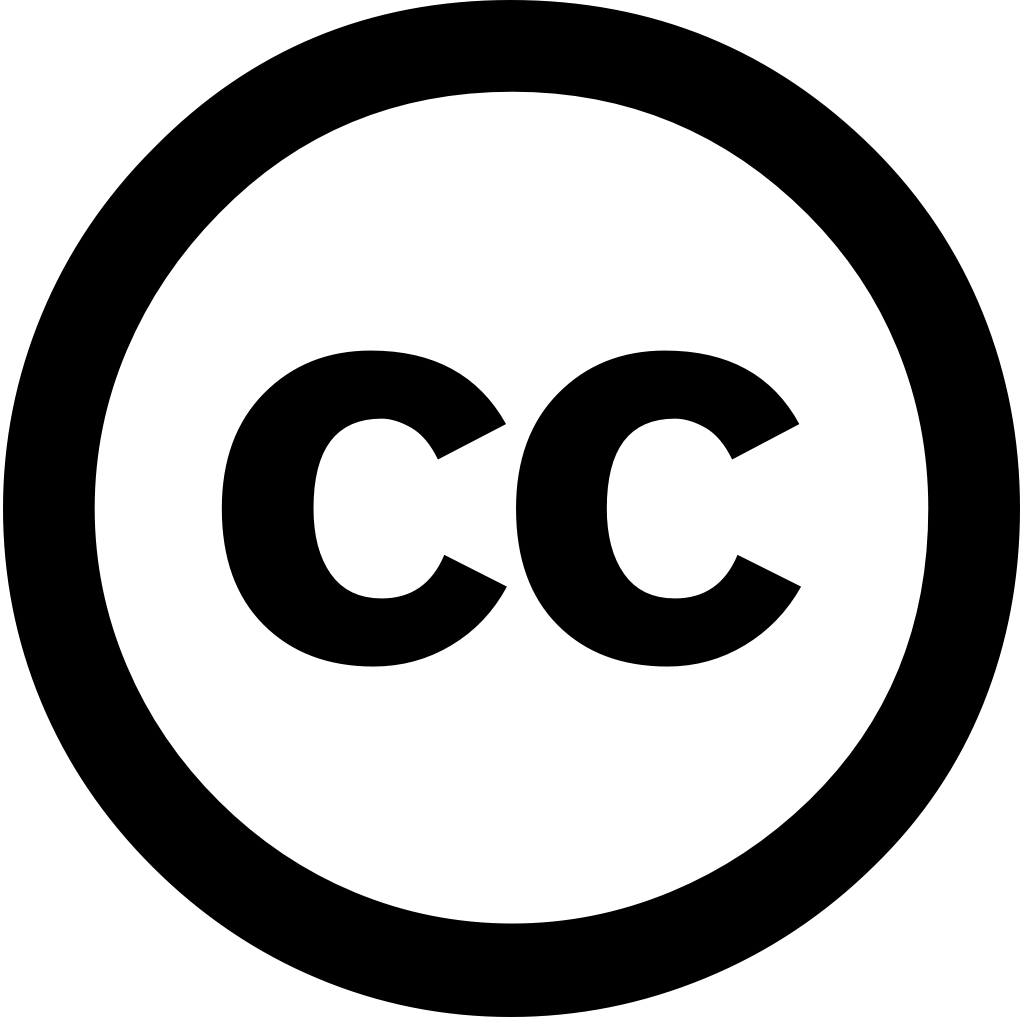
Frontiers in Computer Science, Journal Year: 2025, Volume and Issue: 7
Published: April 10, 2025
Introduction The accurate and timely diagnosis of skin diseases is a critical concern, as many exhibit similar symptoms in the early stages. Most existing automated detection/classification approaches that utilize machine learning or deep poses privacy issues, they involve centralized computing require local storage for data training. Methods Keeping sensitive patient primary objective, addition to ensuring accuracy efficiency, this paper presents an algorithm integrates Federated techniques into IoT-based edge-computing environment. purpose proposed technique protect by training model locally on edge device transferring only weights central server where aggregation takes place. This process ensures security at level eliminates need storage. Furthermore, framework enhances network’s real-time processing capabilities using IoT-integrated sensors, which turn facilitates swift diagnoses. In addition, also focuses design execution federated framework, includes power, memory, number nodes present network. Results effectiveness are demonstrated precise parameters, such accuracy, precision, f 1-score, recall, along with all intricacies secure approach. achieved 98.6%. As was trained locally, bandwidth utilization almost negligible. Discussion can assist specialists diagnosing conditions. Additionally, learning, continuously improves new input accumulates, enhancing subsequent rounds.
Language: Английский