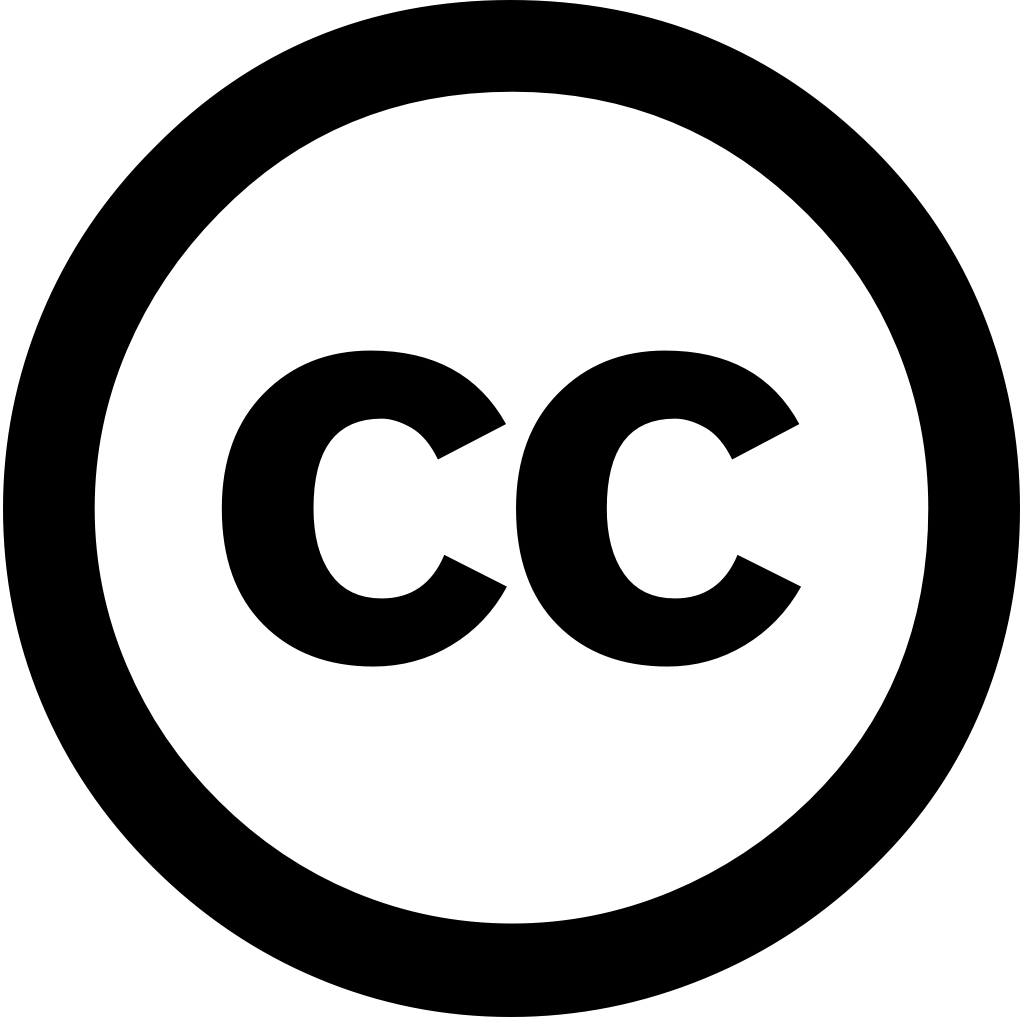
medRxiv (Cold Spring Harbor Laboratory), Journal Year: 2024, Volume and Issue: unknown
Published: Aug. 6, 2024
ABSTRACT Background Circulating immunometabolic biomarkers show promise for the diagnosis and treatment monitoring of tuberculosis (TB). However, that can distinguish TB from nontuberculous mycobacteria (NTM) infections, latent infection (LTBI), other lung diseases (ODx) have not been elucidated. This study utilized a multi-cohort, multi-omics approach combined with predictive modeling to identify, validate, prioritize active TB. Methods Functional omics data were collected two discovery cohorts (76 patients in TB-NTM cohort 72 TB-LTBI-ODx cohort) one validation (68 30 LTBI patients). An integrative analysis was performed identify plasma multi-ome biosignatures. Machine learning-based then applied assess performance these biosignatures most promising candidates. Results Conventional statistical analyses immune profiling metabolomics indicated minor differences between non-TB groups, whereas lipidome showed significant alteration. Muti-omics identified three could performance, achieving area under ROC curve (AUC) values 0.7–0.9 across groups both cohorts. The lipid PC(14:0_22:6) emerged as important predictor differentiating controls, consistently presenting at lower levels group compared counterparts. Further using independent external datasets demonstrated AUCs 0.77–1.00, confirming biomarkers’ efficacy distinguishing groups. Conclusion Our reveals alteration Predictive suggests lipids differential diagnosis. External further indicates potential diagnostic marker candidate Summary integration reliable highlighted importance circulating (TB) complex conditions similar clinical manifestations, healthy individuals.
Language: Английский