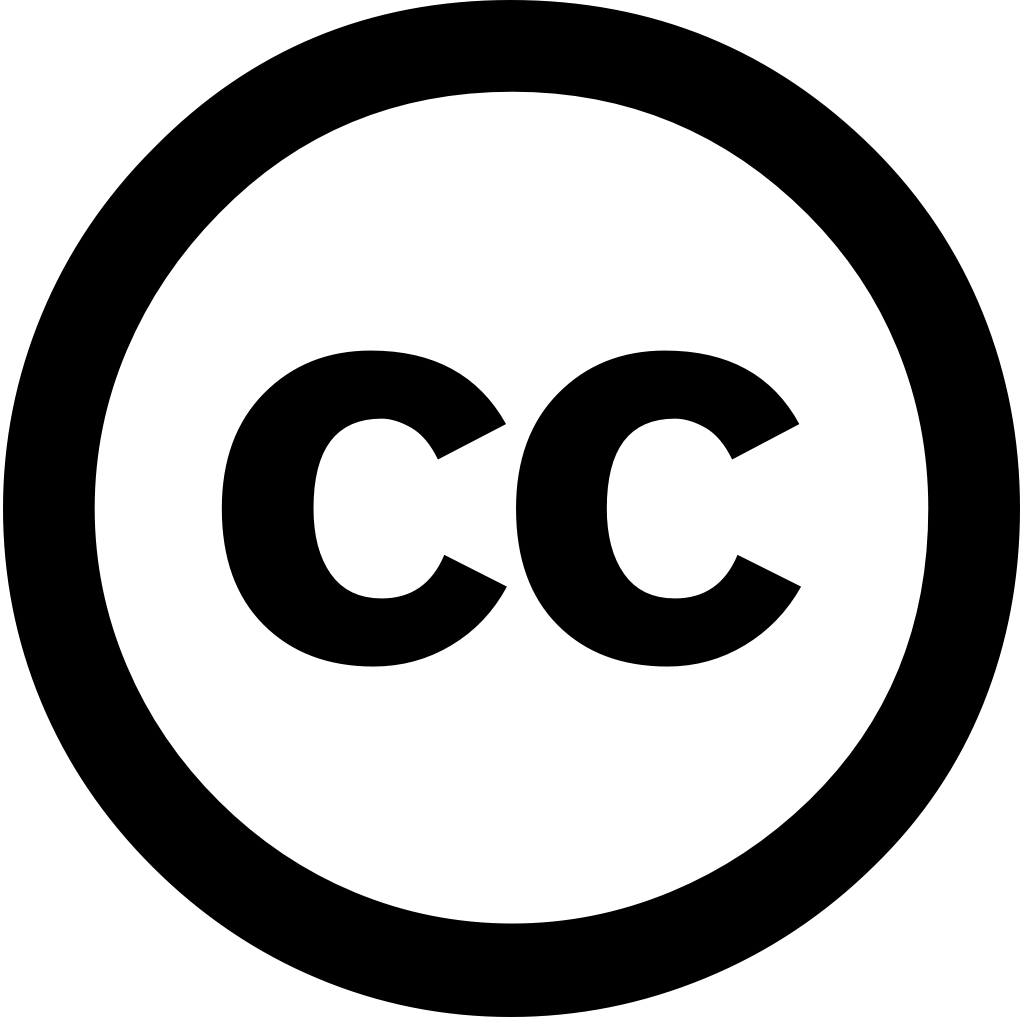
Scientific Reports, Journal Year: 2025, Volume and Issue: 15(1)
Published: April 17, 2025
With the enlarged utilization of computer networks, security has become one critical issues. A network intrusion by malicious or unauthorized consumers may cause severe interruption to networks. So, progress a strong and dependable detection system (IDS) is gradually significant. Intrusion relates suite models employed recognize attacks against infrastructures computers. There are dual main models, such as misuse anomaly detection. Anomaly central part in which disruptions normal behaviour propose presence unintentionally intentionally induced attacks, defects, faults, etc. arrival anomaly-based IDS, many have progressed tracking new threats systems. Machine learning (ML) deep (DL) currently leveraged for cybersecurity. This manuscript proposes an Enhanced Detection using Optimization Algorithm with Dimensionality Reduction Hybrid Model (EAID-OADRHM) technique. The proposed EAID-OADRHM technique presents approach perceiving migrating Min-max scaling normalization primarily at data pre-processing level clean transform input into consistent range. Furthermore, utilizes equilibrium optimizer (EO) model dimensionality reduction process. Additionally, classification performed employing long short-term memory autoencoder (LSTM-AE) model. Finally, improved Snow Ablation Optimizer (ISAO) optimally tunes hyperparameters LSTM-AE model, leading enhanced performance. simulation validation examined under CIC-IDS2017 dataset, outcomes computed numerous measures. experimental assessment portrayed superior accuracy value 99.46% over existing methods
Language: Английский