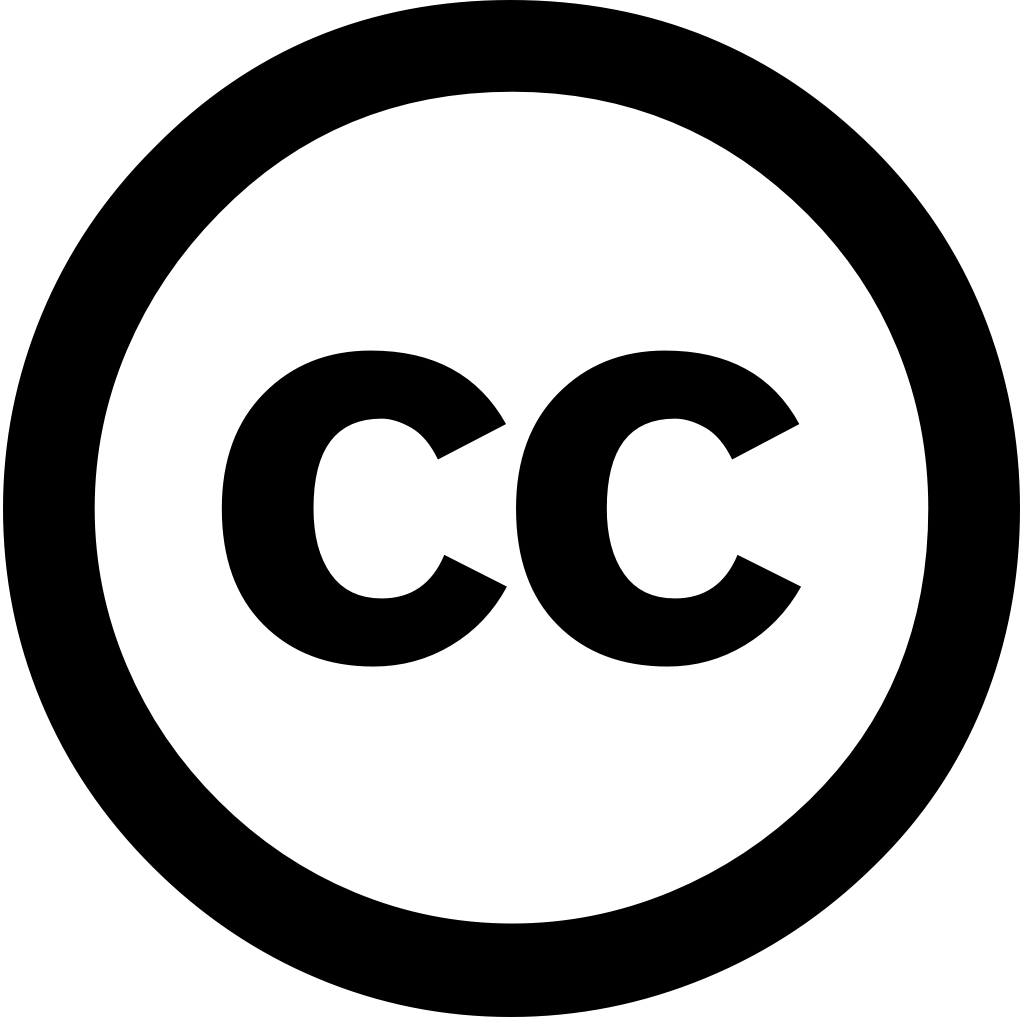
Complex & Intelligent Systems, Journal Year: 2024, Volume and Issue: 11(1)
Published: Dec. 30, 2024
Language: Английский
Complex & Intelligent Systems, Journal Year: 2024, Volume and Issue: 11(1)
Published: Dec. 30, 2024
Language: Английский
Radiotherapy and Oncology, Journal Year: 2024, Volume and Issue: 201, P. 110542 - 110542
Published: Sept. 17, 2024
Language: Английский
Citations
6Physics and Imaging in Radiation Oncology, Journal Year: 2025, Volume and Issue: unknown, P. 100704 - 100704
Published: Jan. 1, 2025
Language: Английский
Citations
0Physics in Medicine and Biology, Journal Year: 2025, Volume and Issue: 70(7), P. 070301 - 070301
Published: March 25, 2025
Language: Английский
Citations
0Physics and Imaging in Radiation Oncology, Journal Year: 2025, Volume and Issue: 33, P. 100743 - 100743
Published: Jan. 1, 2025
Language: Английский
Citations
0Physics in Medicine and Biology, Journal Year: 2025, Volume and Issue: 70(9), P. 09TR01 - 09TR01
Published: April 24, 2025
Abstract The fundamental goal in radiation therapy (RT) is to simultaneously maximize tumor cell killing and healthy tissue sparing. Reducing uncertainty margins improves normal sparing, but generally requires advanced techniques. Adaptive RT (ART) a compelling technique that leverages daily imaging anatomical information support reduced optimize plan quality for each treatment fraction. An especially exciting avenue ART proton (PT), which aims combine re-optimization with the unique advantages provided by protons, including integral dose near-zero deposition distal target along beam direction. A core component onboard image guidance, currently two options are available on systems, cone-beam computed tomography (CBCT) CT-on-rail (CToR) imaging. While CBCT suffers from poorer compared CToR imaging, platforms can be more easily integrated PT systems thus may streamlined adaptive (APT). In this review, we present current status of application evaluation adaptation, progress, challenges future directions.
Language: Английский
Citations
0medRxiv (Cold Spring Harbor Laboratory), Journal Year: 2024, Volume and Issue: unknown
Published: May 13, 2024
Abstract Background/purpose The use of artificial intelligence (AI) in radiotherapy (RT) is expanding rapidly. However, there exists a notable lack clinician trust AI models, underscoring the need for effective uncertainty quantification (UQ) methods. purpose this study was to scope existing literature related UQ RT, identify areas improvement, and determine future directions. Methods We followed PRISMA-ScR scoping review reporting guidelines. utilized population (human cancer patients), concept (utilization UQ), context (radiotherapy applications) framework structure our search screening process. conducted systematic spanning seven databases, supplemented by manual curation, up January 2024. Our yielded total 8980 articles initial review. Manuscript data extraction performed Covidence. Data categories included general characteristics, RT characteristics. Results identified 56 published from 2015-2024. 10 domains applications were represented; most studies evaluated auto-contouring (50%), image-synthesis (13%), multiple simultaneously (11%). 12 disease sites represented, with head neck being common site independent application space (32%). Imaging used 91% studies, while only 13% incorporated dose information. Most focused on failure detection as main (60%), Monte Carlo dropout commonly implemented method (32%) ensembling (16%). 55% did not share code or datasets. Conclusion revealed diversity beyond auto-contouring. Moreover, clear additional methods, such conformal prediction. results may incentivize development guidelines implementation RT.
Language: Английский
Citations
1Physics and Imaging in Radiation Oncology, Journal Year: 2024, Volume and Issue: 32, P. 100658 - 100658
Published: Oct. 1, 2024
Language: Английский
Citations
1Deleted Journal, Journal Year: 2024, Volume and Issue: unknown
Published: Nov. 7, 2024
Cone-beam computed tomography (CBCT) is widely utilized in image-guided radiation therapy; however, its image quality poor compared to planning CT (pCT), thus restricting utility for adaptive radiotherapy (ART). Our objective was enhance CBCT utilizing a transformer-based deep learning model, SwinUNETR, which we with conventional convolutional neural network (CNN) U-net. This retrospective study involved 260 patients undergoing prostate radiotherapy, 245 used training and 15 reserved as an independent hold-out test dataset. Employing CycleGAN framework, generated synthetic (sCT) images from images, employing SwinUNETR U-net generators. We evaluated sCT assessed dosimetric impact photon therapy through gamma analysis dose-volume histogram (DVH) comparisons. The mean absolute error values the numbers, calculated using all voxels within patient's body contour taking pCT reference, were 84.07, 73.49, 64.69 Hounsfield units CBCT, U-net, respectively. Gamma revealed superior agreement between dose on SwinUNETR-based plans those based DVH parameters deviated by < 1% plans. showed that, could proficiently generate more precise facilitating accurate calculations. demonstrates superiority of models over CNN-based approaches CBCT-to-CT translation, contributing advancement synthesis techniques ART.
Language: Английский
Citations
1The European Physical Journal Plus, Journal Year: 2024, Volume and Issue: 139(10)
Published: Oct. 9, 2024
Language: Английский
Citations
0Sensors, Journal Year: 2024, Volume and Issue: 24(23), P. 7460 - 7460
Published: Nov. 22, 2024
Image-guided treatment adaptation is a game changer in oncological particle therapy (PT), especially for younger patients. The purpose of this study to present cycle generative adversarial network (CycleGAN)-based method synthetic computed tomography (sCT) generation from cone beam CT (CBCT) towards adaptive PT (APT) paediatric Firstly, 44 CBCTs 15 young pelvic patients were pre-processed reduce ring artefacts and rigidly registered on same-day scans (i.e., verification scans, vCT scans) then inputted the CycleGAN (employing either Res-Net U-Net generators) synthesise sCT. In particular, 36 8 volumes used training testing, respectively. Image quality was evaluated qualitatively quantitatively using structural similarity index metric (SSIM) peak signal-to-noise ratio (PSNR) between CBCT (rCBCT) sCT evaluate improvements brought by CycleGAN. Despite limitations due sub-optimal input image small field view (FOV), found be overall satisfactory quantitative qualitative perspective. Our findings indicate that promising produce with acceptable CT-like texture settings, even when narrow fields (FOV) are employed.
Language: Английский
Citations
0