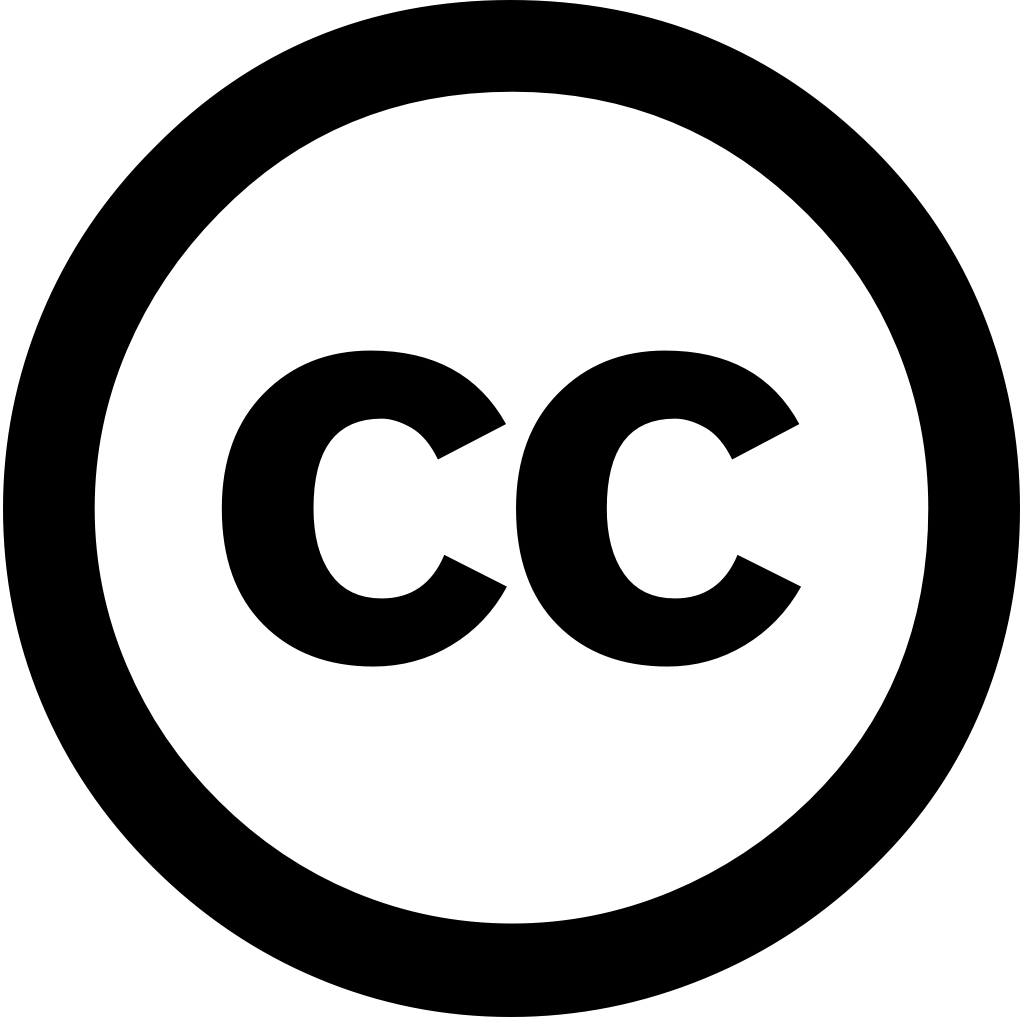
Journal of Agriculture and Food Research, Journal Year: 2025, Volume and Issue: 21, P. 101948 - 101948
Published: April 23, 2025
Language: Английский
Journal of Agriculture and Food Research, Journal Year: 2025, Volume and Issue: 21, P. 101948 - 101948
Published: April 23, 2025
Language: Английский
International Journal of Digital Earth, Journal Year: 2025, Volume and Issue: 18(1)
Published: Jan. 27, 2025
Language: Английский
Citations
1Global Food Security, Journal Year: 2025, Volume and Issue: 44, P. 100841 - 100841
Published: Feb. 24, 2025
Language: Английский
Citations
0Environmental Research Communications, Journal Year: 2025, Volume and Issue: 7(3), P. 035006 - 035006
Published: Feb. 24, 2025
Abstract Predicting crop yields remains a crucial challenge in agriculture, as these forecasts influence decision-making at global, regional, and individual levels. Historically, such predictions have utilized diverse data sources, including agricultural, land, climatic, atmospheric, other pertinent information. Over the past several years, application of machine learning techniques has emerged valuable analytical approach for estimating agricultural productivity, thereby informing decisions regarding selection management strategies throughout entire growing cycle. Various kinds models been research to forecast yields. Our work proposes stacked ensemble model designed purpose predicting yield. The proposed employs approach, with Decision Tree Regressor functioning meta-model amalgamate from six distinct base learner models: Linear Regression (LR), Elastic Net, XGBoost Regressor, K-Neighbors (KNR), AdaBoost Random Forest (RFR). achieves superior yield prediction performance, evidenced by notable enhancement accuracy significant decrease RMSE, surpassing predictive capabilities traditional models. model’s performance was assessed using metrics, Mean Absolute Error 7.20 tons/hectare, Square 15570.32 tons 2 /hectare , Root 124.78 Coefficient Determination (R Score) 0.98. results demonstrate that outperforms conventional approaches, achieving high R-squared score 98%.
Language: Английский
Citations
0Computers and Electronics in Agriculture, Journal Year: 2025, Volume and Issue: 234, P. 110317 - 110317
Published: March 23, 2025
Language: Английский
Citations
0Smart Agricultural Technology, Journal Year: 2025, Volume and Issue: unknown, P. 100909 - 100909
Published: March 1, 2025
Language: Английский
Citations
0Communications Earth & Environment, Journal Year: 2025, Volume and Issue: 6(1)
Published: April 16, 2025
Language: Английский
Citations
0Journal of Agriculture and Food Research, Journal Year: 2025, Volume and Issue: 21, P. 101948 - 101948
Published: April 23, 2025
Language: Английский
Citations
0