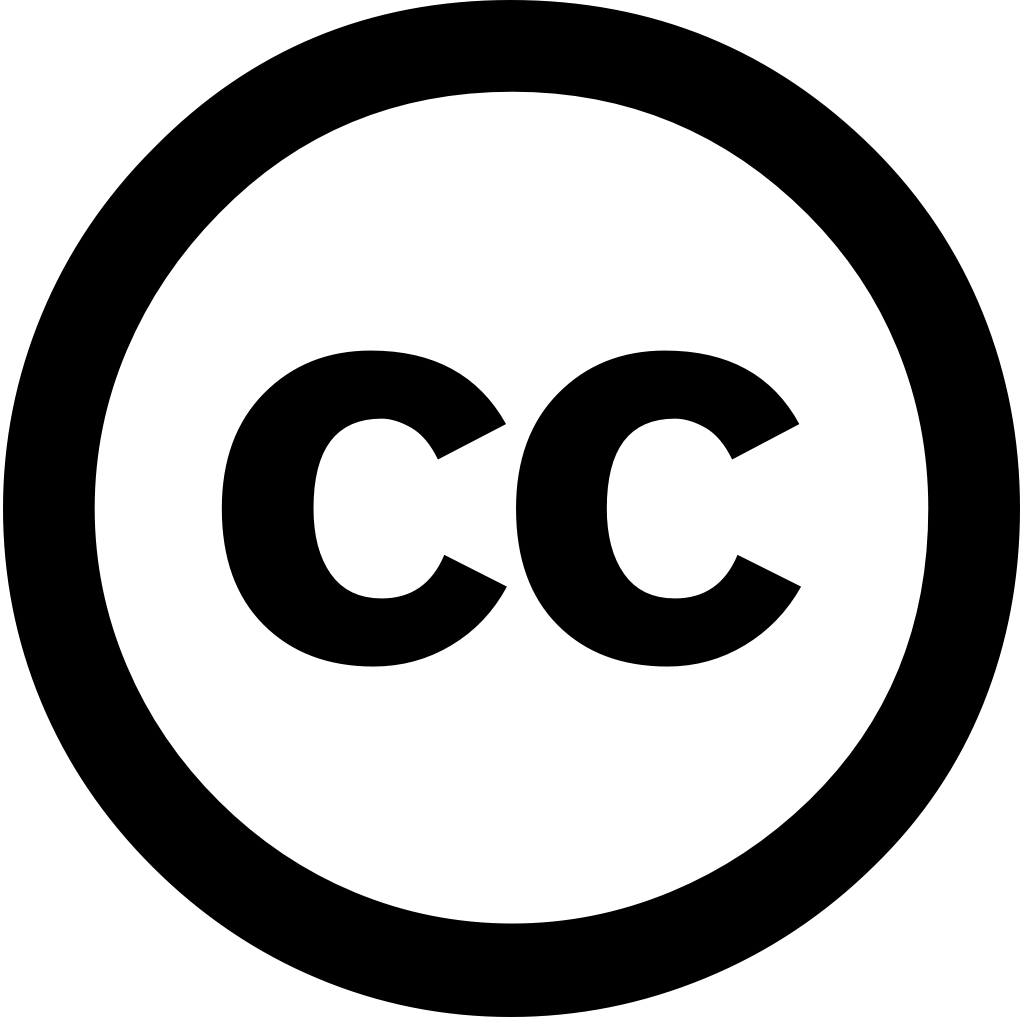
IEEE Journal of Selected Topics in Applied Earth Observations and Remote Sensing, Journal Year: 2024, Volume and Issue: 18, P. 1139 - 1152
Published: Nov. 19, 2024
Language: Английский
IEEE Journal of Selected Topics in Applied Earth Observations and Remote Sensing, Journal Year: 2024, Volume and Issue: 18, P. 1139 - 1152
Published: Nov. 19, 2024
Language: Английский
Aquatic Toxicology, Journal Year: 2025, Volume and Issue: 279, P. 107244 - 107244
Published: Jan. 8, 2025
Language: Английский
Citations
0Journal of Chemical Information and Modeling, Journal Year: 2025, Volume and Issue: unknown
Published: Feb. 17, 2025
Single-cell RNA sequencing (scRNA-seq) has emerged as a transformative technology for elucidating cellular heterogeneity at unprecedented resolution. However, technical limitations such limited depth and mRNA capture efficiency often result in zero counts, commonly referred to "dropout zeros" scRNA-seq data. These zeros pose significant challenges downstream analysis, they can distort the interpretation of transcriptomes. While numerous computational methods have been developed address this challenge, existing approaches frequently suffer from either insufficient imputation (under-imputation) or excessive modification (over-imputation). Here, we propose precisely balanced (PbImpute) method designed achieve optimal equilibrium between dropout recovery biological preservation PbImpute employs multistage approach: (1) Initial discrimination dropouts through parameter optimization new zero-inflated negative binomial (ZINB) distribution model, followed by initial imputation; (2) Application uniquely static repair algorithm enhance data fidelity; (3) Secondary identification based on gene expression frequency partition-specific coefficient variation; (4) Graph-embedding neural network-based (5) Implementation dynamic mechanism mitigate over-imputation effects. distinguishes itself integrating ZINB modeling with repair. This advantageous combined approach achieves balance over- under-imputation, while simultaneously preserving true reducing signal distortion. Comprehensive evaluation using both simulated real sets demonstrated that superior performance (F1 Score = 0.88 83% rate, ARI 0.78 PBMC) discriminating compared state-of-the-art methods. The significantly improves gene–gene cell–cell correlation structures, enhances differential analysis sensitivity, optimizes clustering resolution dimensional reduction visualization, facilitates more accurate trajectory inference. Ablation studies confirmed essential contribution modules method's performance. code is available https://github.com/WyBioTeam/PbImpute. By enhancing accuracy imputation, improve cell subpopulations detection differentially expressed genes, thereby facilitating precise analyses advancing disease research.
Language: Английский
Citations
0Advanced Science, Journal Year: 2025, Volume and Issue: unknown
Published: March 5, 2025
Single-cell RNA sequencing (scRNA-seq) has unveiled extensive cellular heterogeneity, yet precise cell type annotation and the identification of novel populations remain significant challenges. scHeteroNet, a graph neural network framework specifically designed to leverage heterophily in scRNA-seq data, is presented. Unlike traditional methods that assume homophily, scHeteroNet captures complex cell-cell interactions by integrating information from both immediate extended neighborhoods, resulting highly accurate representations. Additionally, incorporates an innovative novelty propagation mechanism robustly detects previously uncharacterized types. Comprehensive evaluations across diverse datasets demonstrate consistently outperforms state-of-the-art approaches classification detection. This heterophily-aware approach enhances ability uncover diversity, providing deeper insights into biological systems advancing field single-cell analysis.
Language: Английский
Citations
0IEEE Journal of Selected Topics in Applied Earth Observations and Remote Sensing, Journal Year: 2024, Volume and Issue: 18, P. 1139 - 1152
Published: Nov. 19, 2024
Language: Английский
Citations
1