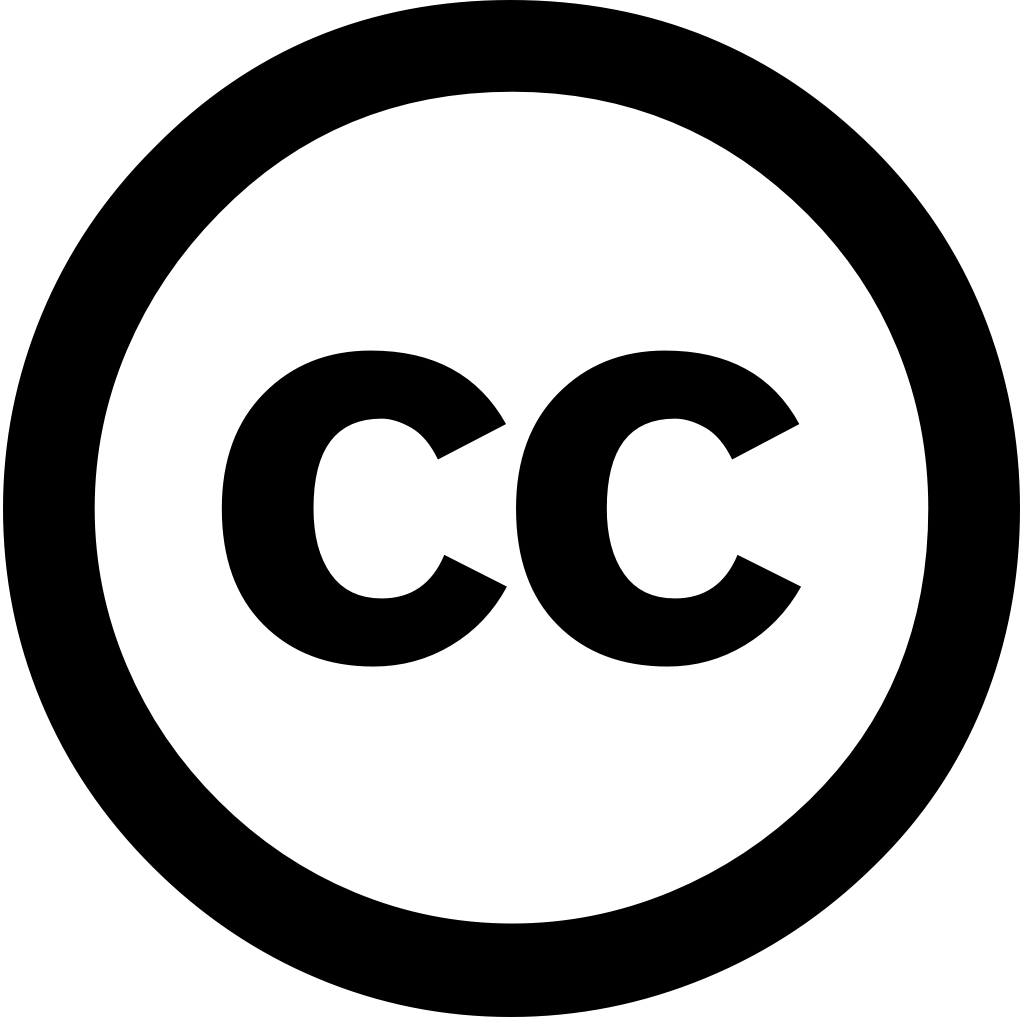
Agriculture, Journal Year: 2025, Volume and Issue: 15(5), P. 445 - 445
Published: Feb. 20, 2025
Nonparametric models have recently been receiving increased attention due to their effectiveness in genomic prediction for complex traits. However, regular nonparametric cannot effectively differentiate the relative importance of various SNPs, which significantly impedes further application these methods prediction. To enhance fitting ability and improve accuracy, a weighted kernel ridge regression model (WKRR) was proposed this study. For new method, different weights were assigned SNPs according p-values from GWAS, then KRR based on constructed Cross-validation adopted choose appropriate hyper-parameters during weighting process generalization. We compared predictive accuracy WKRR with best linear unbiased (GBLUP), BayesR, unweighted using both simulated real datasets. The results showed that outperformed all scenarios. Additionally, achieved an average improvement 1.70% accuracies across traits mice dataset 2.17% three lactation-related cattle GBLUP, yielded competitive BayesR. These findings demonstrated great potential
Language: Английский