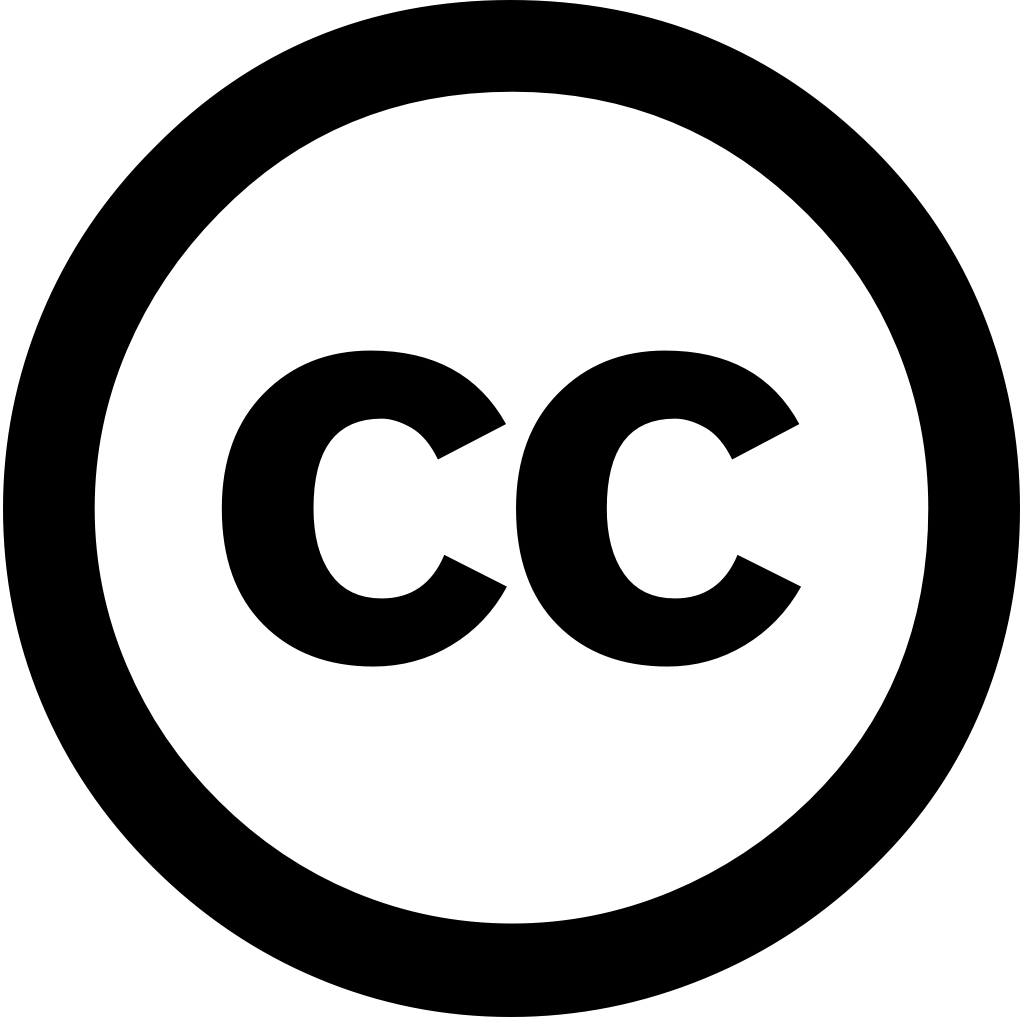
GigaScience, Journal Year: 2024, Volume and Issue: 13
Published: Jan. 1, 2024
Identification of protein-protein and protein-nucleic acid binding sites provides insights into biological processes related to protein functions technical guidance for disease diagnosis drug design. However, accurate predictions by computational approaches remain highly challenging due the limited knowledge residue patterns. The pattern a should be characterized spatial distribution its neighboring residues combined with their physicochemical information interaction, which yet cannot achieved previous methods. Here, we design GraphRBF, hierarchical geometric deep learning model learn patterns from big data. To achieve it, GraphRBF describes interactions designing an enhanced graph neural network characterizes distributions introducing prioritized radial basis function network. After training testing, shows great improvements over existing state-of-the-art methods strong interpretability learned representations. Applying SARS-CoV-2 omicron spike protein, it successfully identifies known epitopes protein. Moreover, predicts multiple potential regions new nanobodies or even drugs evidence. A user-friendly online server is freely available at http://liulab.top/GraphRBF/server.
Language: Английский