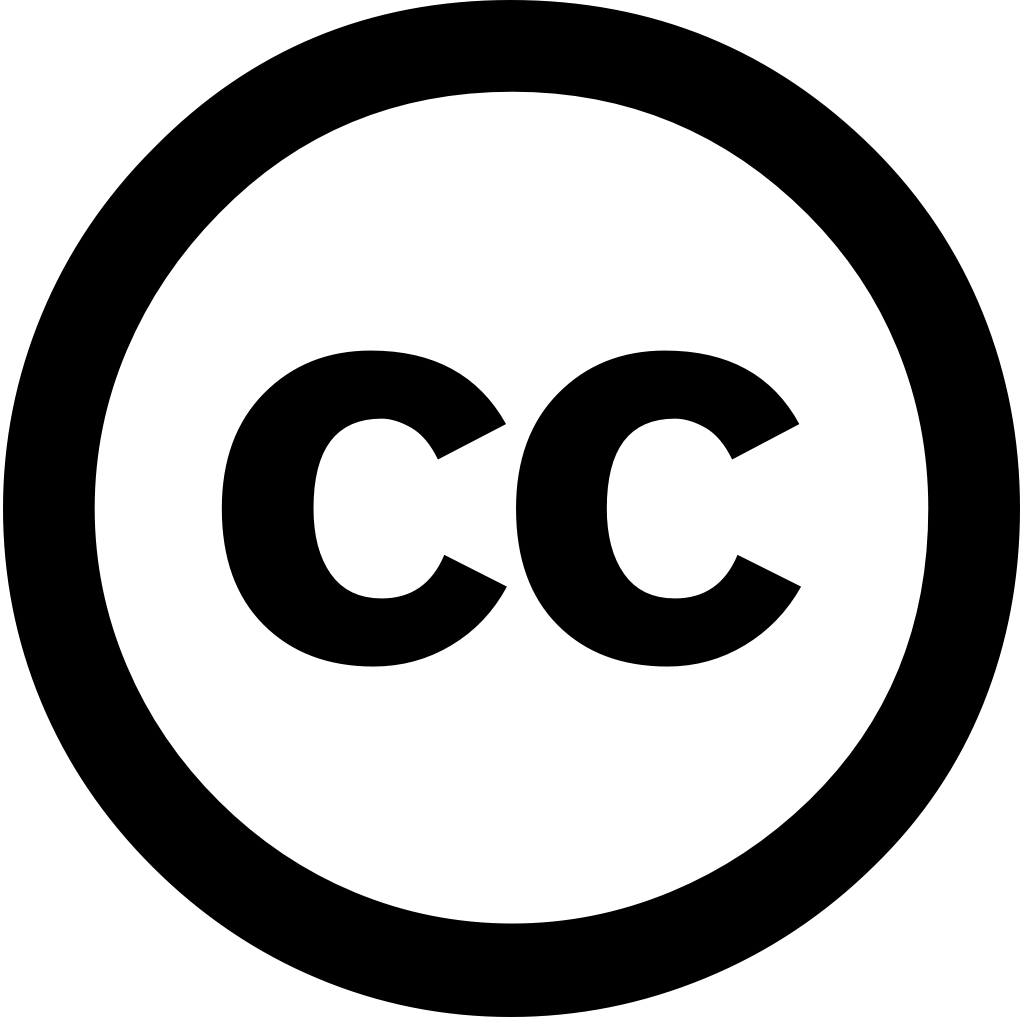
Journal of Computational Design and Engineering, Journal Year: 2024, Volume and Issue: 11(4), P. 249 - 305
Published: July 3, 2024
Abstract Crayfish optimization algorithm (COA) is a novel bionic metaheuristic with high convergence speed and solution accuracy. However, in some complex problems real application scenarios, the performance of COA not satisfactory. In order to overcome challenges encountered by COA, such as being stuck local optimal insufficient search range, this paper proposes four improvement strategies: search-hide, adaptive spiral elite greedy opposition-based learning, competition-elimination, chaos mutation. To evaluate accuracy, speed, robustness modified crayfish (MCOA), simulation comparison experiments 10 algorithms are conducted. Experimental results show that MCOA achieved minor Friedman test value 23 functions, CEC2014 CEC2020, average superiority rates 80.97%, 72.59%, 71.11% WT, respectively. addition, shows applicability progressiveness five engineering actual industrial field. Moreover, 80% 100% rate against on CEC2020 fixed-dimension function benchmark functions. Finally, owns better population diversity.
Language: Английский