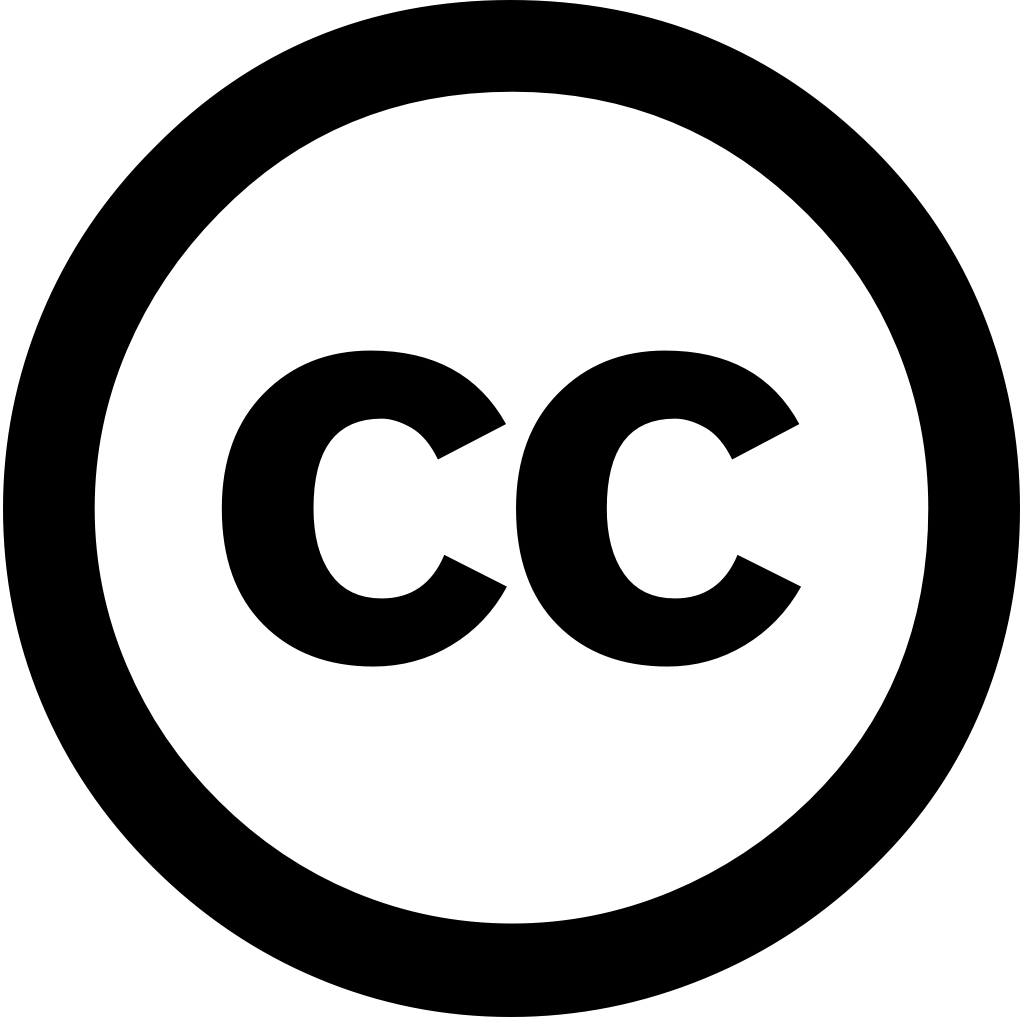
Briefings in Bioinformatics, Journal Year: 2025, Volume and Issue: 26(2)
Published: March 1, 2025
Abstract Synthetic lethality (SL) is a promising gene interaction for cancer therapy. Recent SL prediction methods integrate knowledge graphs (KGs) into graph neural networks (GNNs) and employ attention mechanisms to extract local subgraphs as explanations target pairs. However, often lack fidelity, typically generate single explanation per pair, fail ensure trustworthy high-order structures in their explanations. To overcome these limitations, we propose Diverse Graph Information Bottleneck Lethality (DGIB4SL), KG-based GNN that generates multiple faithful the same pair effectively encodes structures. Specifically, introduce novel DGIB objective, integrating determinant point process constraint standard information bottleneck 13 motif-based adjacency matrices capture representations. Experimental results show DGIB4SL outperforms state-of-the-art baselines provides prediction, revealing diverse biological underlying inference.
Language: Английский