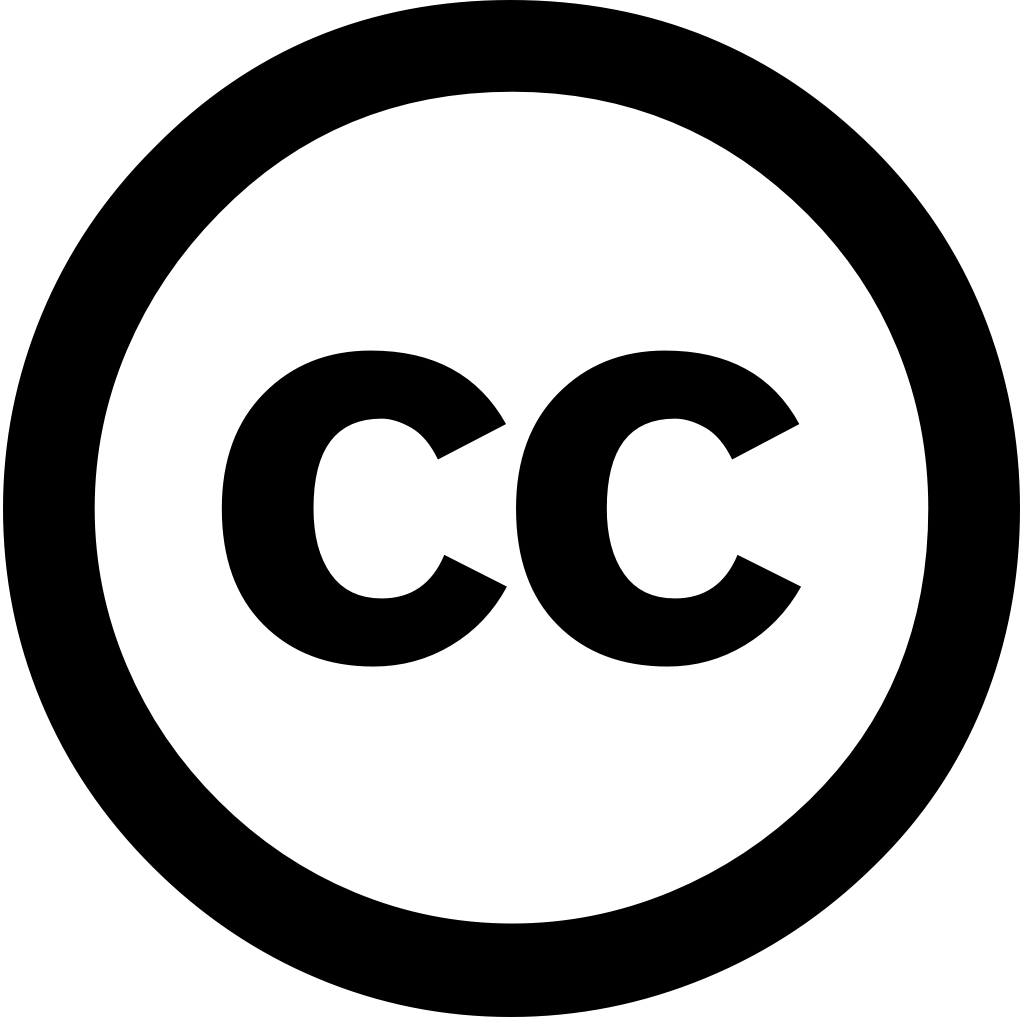
Plant and Cell Physiology, Journal Year: 2023, Volume and Issue: 64(8), P. 841 - 843
Published: June 18, 2023
Plant and Cell Physiology, Journal Year: 2023, Volume and Issue: 64(8), P. 841 - 843
Published: June 18, 2023
Briefings in Bioinformatics, Journal Year: 2024, Volume and Issue: 25(4)
Published: May 23, 2024
Abstract Unlike animals, variability in transcription factors (TFs) and their binding regions (TFBRs) across the plants species is a major problem that most of existing TFBR finding software fail to tackle, rendering them hardly any use. This limitation has resulted into underdevelopment plant regulatory research rampant use Arabidopsis-like model species, generating misleading results. Here, we report revolutionary transformers-based deep-learning approach, PTFSpot, which learns from TF structures regions’ co-variability bring universal TF-DNA interaction detect with complete freedom species-specific models’ limitations. During series extensive benchmarking studies over multiple experimentally validated data, it not only outperformed by >30% lead but also delivered consistently >90% accuracy even for those families were never encountered during model-building process. PTFSpot makes possible now accurately annotate TFBRs genome total lack information, completely free bottlenecks TF-specific models.
Language: Английский
Citations
5Plant Direct, Journal Year: 2023, Volume and Issue: 7(11)
Published: Nov. 1, 2023
Pineapple (
Language: Английский
Citations
4Heliyon, Journal Year: 2024, Volume and Issue: 10(20), P. e39140 - e39140
Published: Oct. 1, 2024
Language: Английский
Citations
1Agronomy Journal, Journal Year: 2023, Volume and Issue: 116(3), P. 1112 - 1125
Published: June 20, 2023
Abstract Increasing the production of three major food crops (MFCs), maize ( Zea mays ), rice Oryza sativa and wheat Triticum aestivum is essential to fulfilling demand for growing human population. may require integration machine learning (ML) into plant breeding programs. However, developing ML tools improve MFCs a daunting task due lack quality data computation resources needed process this information. Hence, review discusses recent applications improving production, including phenotyping, yield forecasting, candidate gene prediction. Based on challenges reported in experiments MFCs, prescribes solutions produce scalable models. This provides valuable insights future studies promotes collective efforts among researchers implementing enhance productivity.
Language: Английский
Citations
3Plant and Cell Physiology, Journal Year: 2022, Volume and Issue: 63(10), P. 1324 - 1325
Published: Sept. 8, 2022
Language: Английский
Citations
4bioRxiv (Cold Spring Harbor Laboratory), Journal Year: 2023, Volume and Issue: unknown
Published: Nov. 11, 2023
ABSTRACT Transcription factors (TFs) are regulatory proteins which bind to a specific DNA region known as the transcription factor binding regions (TFBRs) regulate rate of process. The identification TFBRs has been made possible by number experimental and computational techniques established during past few years. process TFBR involves peak in data, followed motif characteristics. Using same data attempts have raise models identify such could save time resources spent for experiments. These approaches depend lot on what way they learn how. existing skewed heavily around human discovery, while plants drastically different genomic setup regulation these grossly ignored. Here, we provide comprehensive study current state matters plant TF discovery algorithms. While doing so, encountered several software tools’ issues rendering tools not usable researches. We fixed them also provided corrected scripts tools. expect this serve guide better understanding care be taken applying them, especially cross-species applications. available at https://github.com/SCBB-LAB/Comparative-analysis-of-plant-TFBS-software .
Language: Английский
Citations
2IEEE/ACM Transactions on Computational Biology and Bioinformatics, Journal Year: 2024, Volume and Issue: 21(5), P. 1542 - 1551
Published: May 27, 2024
DNA
motif
is
the
pattern
shared
by
similar
fragments
in
sequences,
which
plays
a
key
role
regulating
gene
expression,
and
discovery
has
become
research
topic.
Exact
planted
(
Language: Английский
Citations
0bioRxiv (Cold Spring Harbor Laboratory), Journal Year: 2023, Volume and Issue: unknown
Published: Nov. 20, 2023
Abstract Unlike animals, variability in transcription factors (TF) and their binding regions (TFBR) across the plants species is a major problem which most of existing TFBR finding software fail to tackle, rendering them hardly any use. This limitation has resulted into underdevelopment plant regulatory research rampant use Arabidopsis like model species, generating misleading results. Here we report revolutionary transformers based deep-learning approach, PTFSpot, learns from TF structures co-variability bring universal TF-DNA interaction detect with complete freedom specific models’ limitations. During series extensive benchmarking studies over multiple experimentally validated data, it not only outperformed by >30% lead, but also delivered consistently >90% accuracy even for those families were never encountered during building process. PTFSpot makes possible now accurately annotate TFBRs genome total lack information, completely free bottlenecks models.
Language: Английский
Citations
1bioRxiv (Cold Spring Harbor Laboratory), Journal Year: 2024, Volume and Issue: unknown
Published: April 28, 2024
Abstract The evolution of gene expression responses are a critical component adaptation to variable environments. Predicting how DNA sequence influences is challenging because the genotype phenotype map not well resolved for cis regulatory elements, transcription factor binding, interactions, and epigenetic features, mention these factors respond environment. We tested if flexible machine learning models could learn some underlying cis- map. this approach using cold-responsive transcriptome profiles in 5 diverse Arabidopsis thaliana accessions. first evidence that regulation plays role environmental response, finding 14 15 motifs were significantly enriched within up- down-stream regions differentially regulated genes (DEGs). next applied convolutional neural networks (CNNs), which de novo sequences predict response found CNNs predicted differential with moderate accuracy, predictions hindered by biological complexity large potential code. Overall, DEGs between specific environments can be based on variation sequences, although more information needs incorporated better may required.
Language: Английский
Citations
0Plants, Journal Year: 2022, Volume and Issue: 12(1), P. 71 - 71
Published: Dec. 23, 2022
Quinoa constitutes among the tolerant plants to challenging and harmful abiotic environmental factors. was selected as model crops destined for bio-saline agriculture that could contribute staple food security an ever-growing worldwide population under various climate change scenarios. The auxin response factors (ARFs) constitute main contributors in plant adaptation severe conditions. Thus, determination of ARF-binding sites represents major step provide promising insights helping breeding programs improving agronomic traits. Hence, determining is a task, particularly species with large genome sizes. In this report, we present data fusion approach based on Dempster–Shafer evidence theory fuzzy set predict sites. We then performed “In-silico” identification Chenopodium quinoa. characterization some known pathways implicated signaling other higher confirms our prediction reliability. Furthermore, several no or little available information about their functions were identified play important roles quinoa predictive genes associated detected may certainly help explore biological unknown newly
Language: Английский
Citations
2