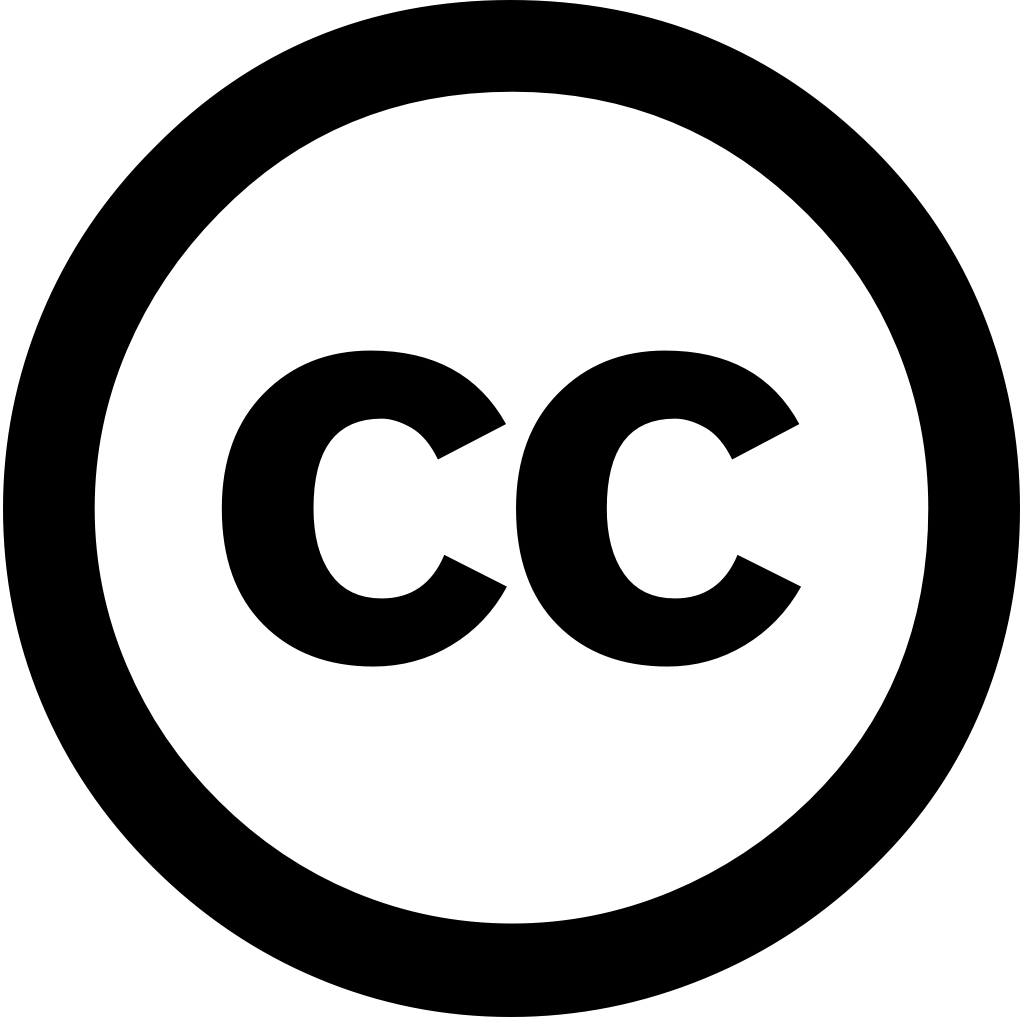
bioRxiv (Cold Spring Harbor Laboratory), Journal Year: 2024, Volume and Issue: unknown
Published: Aug. 20, 2024
Abnormal DNA ploidy, found in numerous cancers, is increasingly being recognized as a contributor driving chromosomal instability, genome evolution, and the heterogeneity that fuels cancer cell progression. Furthermore, it has been linked with poor prognosis of patients. While next-generation sequencing can be used to approximate tumor high error rate for near-euploid states, cost time consuming, motivating alternative rapid quantification methods. We introduce PloiViT, transformer-based model ploidy outperforms traditional machine learning models, enabling cost-effective directly from pathology slides. trained PloiViT on dataset fifteen types The Cancer Genome Atlas validated its performance multiple independent cohorts. Additionally, we explored impact self-supervised feature extraction performance. using features, achieved lowest prediction cohorts, exhibiting better generalization capabilities. Our findings demonstrate predicts higher values aggressive groups patients specific mutations, validating potential complementary assessment data. To further promote use, release our models user-friendly inference application Python package easy adoption use.
Language: Английский