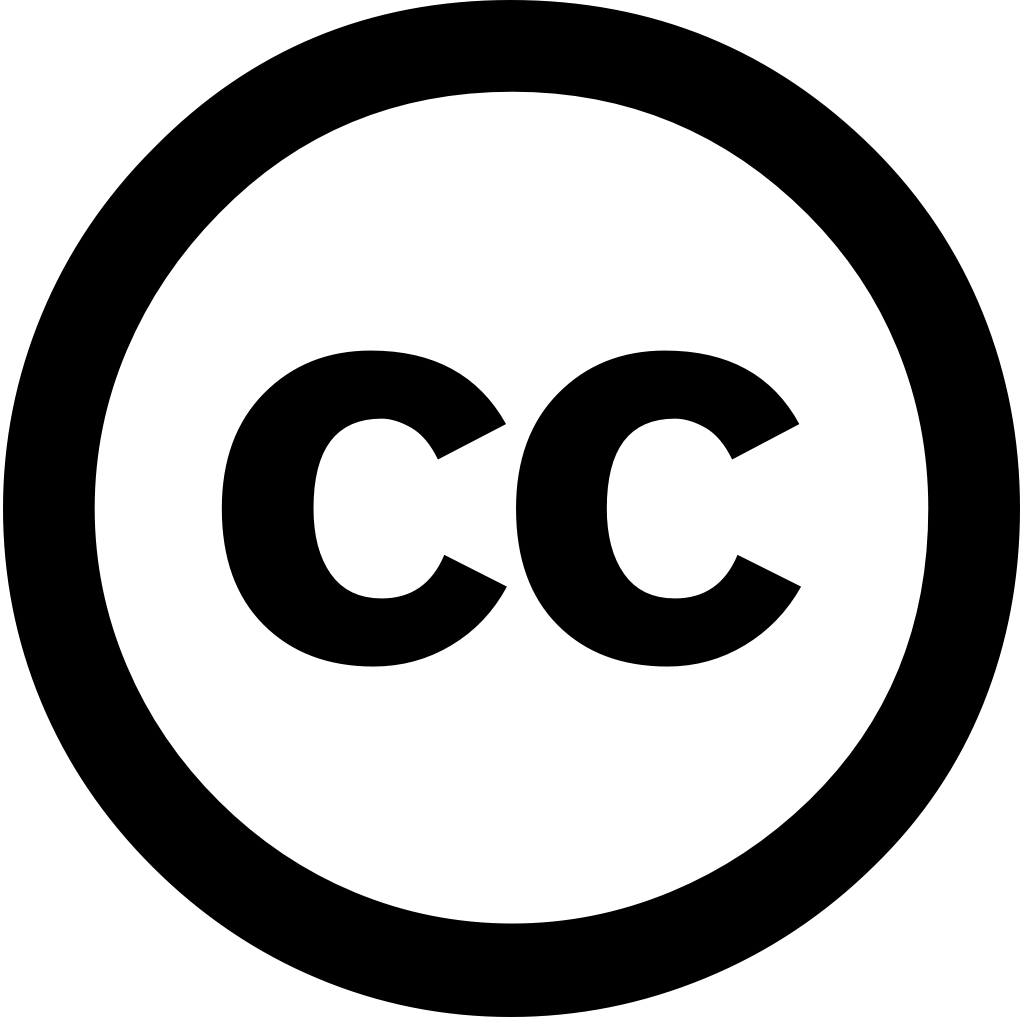
BMC Bioinformatics, Journal Year: 2025, Volume and Issue: 26(1)
Published: Feb. 11, 2025
Abstract Background Alterations of metabolism, including changes in mitochondrial metabolism as well glutathione (GSH) are a appreciated hallmark many cancers. Mitochondrial GSH (mGSH) transport is poorly characterized aspect which we investigate the context cancer. Existing functional annotation approaches from machine (ML) or deep learning (DL) models based only on protein sequences, were unable to annotate functions biological contexts. Results We develop flexible ML framework for diverse feature data. This hybrid leverages cancer cell line multi-omics data and other knowledge features, uncover potential genes involved mGSH membrane achieves strong performance across tasks several primary tumor samples. For our application, classification predict known transporter SLC25A39 but not SLC25A40 being highly probably related SLC25A10, SLC25A50, orphan SLC25A24, SLC25A43 predicted be associated with multiple contexts structural analysis these proteins reveal similarities substrate binding regions residues SLC25A39. Conclusion These findings have implications better understanding novel therapeutic targets respect through annotations genes. The proposed here can applied function classifications datasets generate hypotheses various Code tutorial generating predictions this available at: https://github.com/lkenn012/mGSH_cancerClassifiers .
Language: Английский