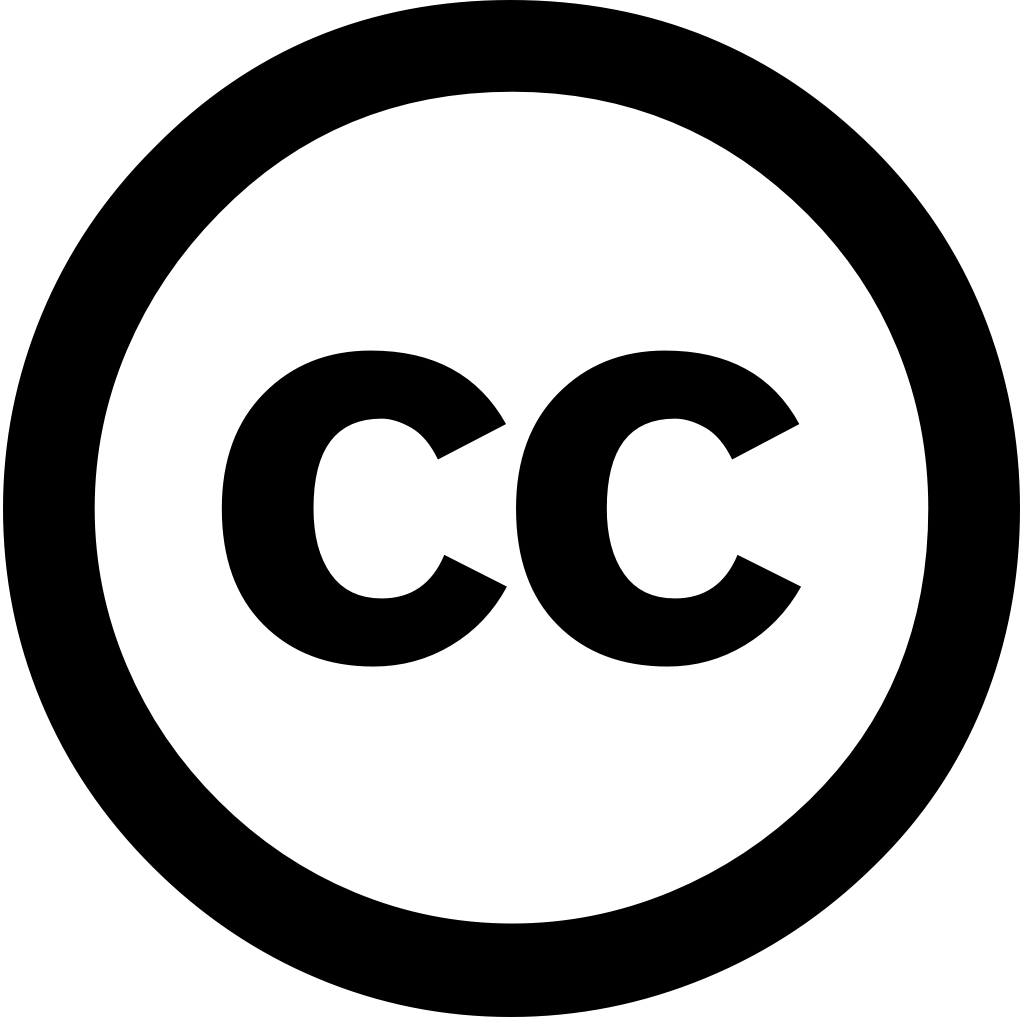
bioRxiv (Cold Spring Harbor Laboratory), Journal Year: 2024, Volume and Issue: unknown
Published: Aug. 7, 2024
Abstract Spatially resolved transcriptomics technologies enable the mapping of multiplexed gene expression profiles within tissue contexts. To explore spatial patterns in complex tissues, computational methods have been developed to identify spatially variable genes single slices. However, there is a lack designed with differential (DSEPs) across multiple slices or conditions, which becomes increasingly common experimental designs. The challenges include complexity cross-slice and information modeling, scalability issues constructing large-scale cell graphs, mixed factors inter-slice heterogeneity. We propose DSEP identification as new task develop River, an interpretable deep learning-based method, solve this task. River comprises two-branch prediction model architecture post-hoc attribution method prioritize that explain condition differences. River’s special design for modeling spatial-informed makes it scalable omics datasets. proposed strategies decouple non-spatial components outcomes. validated performance using simulated datasets applied genes/proteins diverse biological contexts, including embryo development, diabetes-induced alterations spermatogenesis, lupus-induced splenic changes. In human triple-negative breast cancer dataset, identified generalizable survival-related DSEPs, unseen patient groups. does not rely on specific data distribution assumptions compatible various types, making versatile analyzing architectures conditions.
Language: Английский