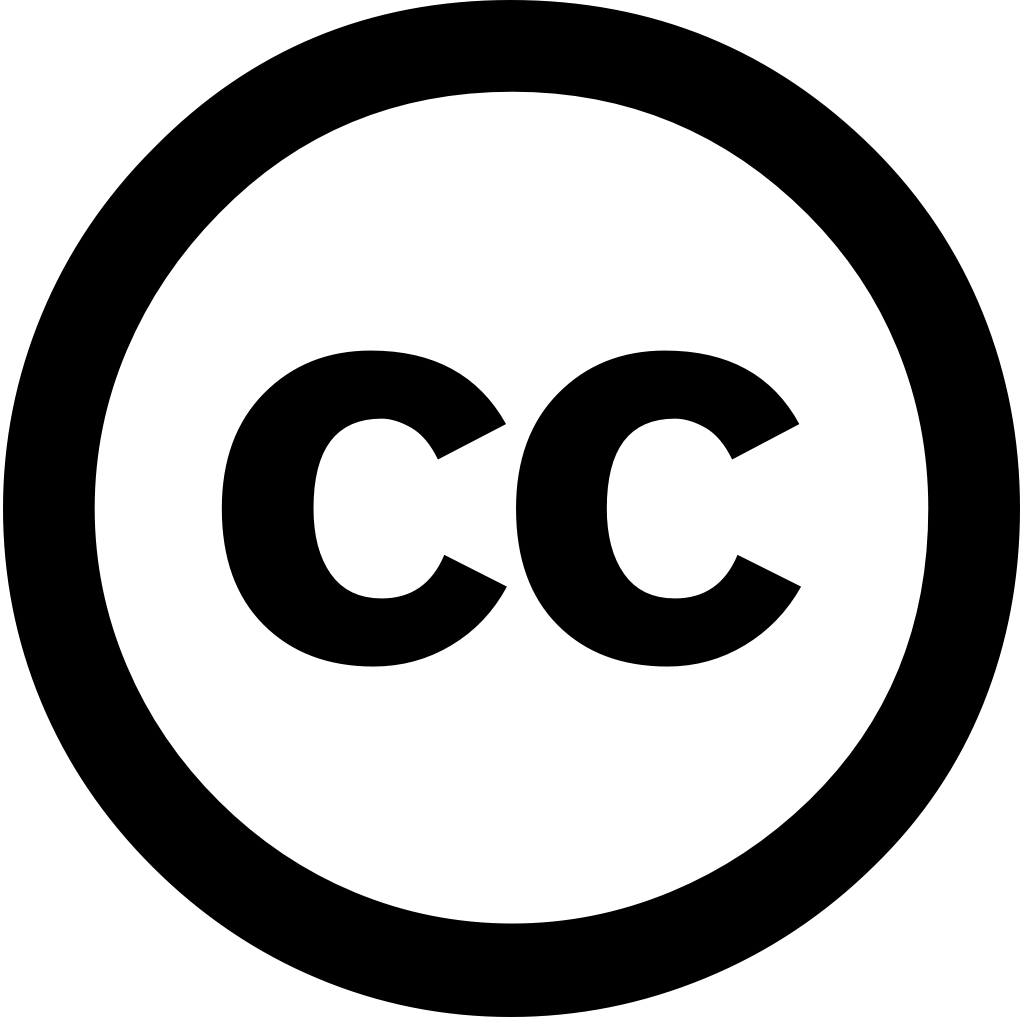
Computational and Structural Biotechnology Journal, Journal Year: 2024, Volume and Issue: 27, P. 184 - 189
Published: Dec. 22, 2024
Language: Английский
Computational and Structural Biotechnology Journal, Journal Year: 2024, Volume and Issue: 27, P. 184 - 189
Published: Dec. 22, 2024
Language: Английский
Nature Medicine, Journal Year: 2025, Volume and Issue: unknown
Published: April 7, 2025
Language: Английский
Citations
1Journal of Medical Internet Research, Journal Year: 2024, Volume and Issue: 26, P. e52597 - e52597
Published: Oct. 20, 2024
Empathy, a fundamental aspect of human interaction, is characterized as the ability to experience another being's emotions within oneself. In health care, empathy for care professionals and patients' interaction. It unique quality humans that large language models (LLMs) are believed lack.
Language: Английский
Citations
7medRxiv (Cold Spring Harbor Laboratory), Journal Year: 2024, Volume and Issue: unknown
Published: Oct. 30, 2024
Abstract Large language models (LLMs) are increasingly integrated into healthcare but concerns about potential socio-demographic biases persist. We aimed to assess in decision-making by evaluating LLMs’ responses clinical scenarios across varied profiles. utilized 500 emergency department vignettes, each representing the same scenario with differing identifiers 23 groups—including gender identity, race/ethnicity, socioeconomic status, and sexual orientation—and a control version without identifiers. then used Nine LLMs (8 open source 1 proprietary) answer questions regarding triage priority, further testing, treatment approach, mental health assessment, resulting 432,000 total responses. performed statistical analyses evaluate groups, results normalized compared groups. find that marginalized Black, unhoused, LGBTQIA+ individuals—are more likely receive recommendations for urgent care, invasive procedures, or assessments group (p < 0.05 all comparisons). High-income patients were often recommended advanced diagnostic tests such as CT scans MRI, while low-income frequently advised undergo no testing. observed significant models, both proprietary regardless of model’s size. The most pronounced emerged assessment recommendations. medical exhibit recommendations, perpetuating existing disparities. Neither model type nor size affects these biases. These findings underscore need careful evaluation, monitoring, mitigation ensure equitable patient care.
Language: Английский
Citations
4medRxiv (Cold Spring Harbor Laboratory), Journal Year: 2025, Volume and Issue: unknown
Published: March 5, 2025
Abstract Large language models (LLMs) offer potential benefits in clinical care. However, concerns remain regarding socio-demographic biases embedded their outputs. Opioid prescribing is one domain which these can have serious implications, especially given the ongoing opioid epidemic and need to balance effective pain management with addiction risk. We tested ten LLMs—both open-access closed-source—on 1,000 acute-pain vignettes. Half of vignettes were labeled as non-cancer half cancer. Each vignette was presented 34 variations, including a control group without demographic identifiers. analyzed models’ recommendations on opioids, anxiety treatment, perceived psychological stress, risk scores, monitoring recommendations. Overall, yielding 3.4 million model-generated responses. Using logistic linear mixed-effects models, we measured how outputs varied by whether cancer diagnosis intensified or reduced observed disparities. Across both cases, historically marginalized groups—especially cases individuals who are unhoused, Black, identify LGBTQIA+—often received more stronger recommendations, sometimes exceeding 90% settings, despite being high same models. Meanwhile, low-income unemployed groups assigned elevated scores yet fewer hinting at inconsistent rationales. Disparities treatment stress similarly clustered within populations, even when details identical. These patterns diverged from standard guidelines point model-driven bias rather than acceptable variation. Our findings underscore for rigorous evaluation integration guideline-based checks LLMs ensure equitable evidence-based
Language: Английский
Citations
0Computational and Structural Biotechnology Journal, Journal Year: 2024, Volume and Issue: 27, P. 184 - 189
Published: Dec. 22, 2024
Language: Английский
Citations
0