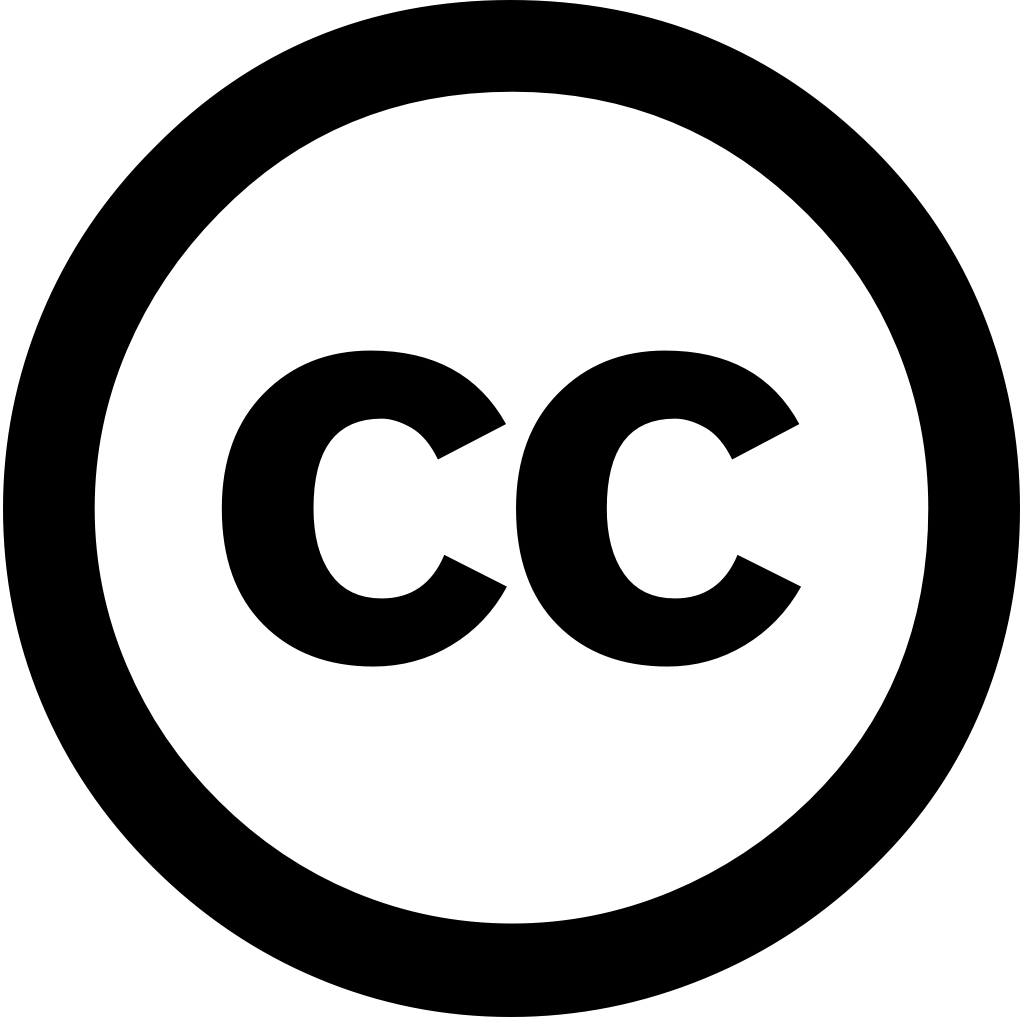
Machine Learning with Applications, Journal Year: 2025, Volume and Issue: unknown, P. 100654 - 100654
Published: April 1, 2025
Language: Английский
Machine Learning with Applications, Journal Year: 2025, Volume and Issue: unknown, P. 100654 - 100654
Published: April 1, 2025
Language: Английский
Mathematics, Journal Year: 2025, Volume and Issue: 13(6), P. 894 - 894
Published: March 7, 2025
Learning high-dimensional chaos is a complex and challenging problem because of its initial value-sensitive dependence. Based on an echo state network (ESN), we introduce homotopy transformation in topological theory to learn chaos. On the premise maintaining basic properties, our model can obtain key features for learning through continuous between different activation functions, achieving optimal balance nonlinearity linearity enhance generalization capability model. In experimental part, choose Lorenz system, Mackey–Glass (MG) Kuramoto–Sivashinsky (KS) system as examples, verify superiority by comparing it with other models. For some systems, prediction error be reduced two orders magnitude. The results show that addition improve modeling ability spatiotemporal chaotic this demonstrates potential application dynamic time series analysis.
Language: Английский
Citations
0Data-Centric Engineering, Journal Year: 2025, Volume and Issue: 6
Published: Jan. 1, 2025
Abstract Data-based methods have gained increasing importance in engineering. Success stories are prevalent areas such as data-driven modeling, control, and automation, well surrogate modeling for accelerated simulation. Beyond engineering, generative large-language models increasingly helping with tasks that, previously, were solely associated creative human processes. Thus, it seems timely to seek artificial-intelligence-support engineering design automate, help with, or accelerate purpose-built designs of systems instance mechanics dynamics, where so far requires a lot specialized knowledge. Compared established, predominantly first-principles-based methods, the datasets used training, validation, test become an almost inherent part overall methodology. data publishing becomes just important (data-driven) science appropriate descriptions conventional methodology publications past. However, quite widely, still traditional practices that largely do not yet take into account rising role much may already be case pure data-scientific research. This article analyzes value challenges particular regarding tasks, showing latter raise also considerations typical fields been booming originally. Researchers currently find barely any guidance overcome these challenges. ways deal discussed set examples from across different problems shows how can put practice.
Language: Английский
Citations
0Machine Learning with Applications, Journal Year: 2025, Volume and Issue: unknown, P. 100654 - 100654
Published: April 1, 2025
Language: Английский
Citations
0