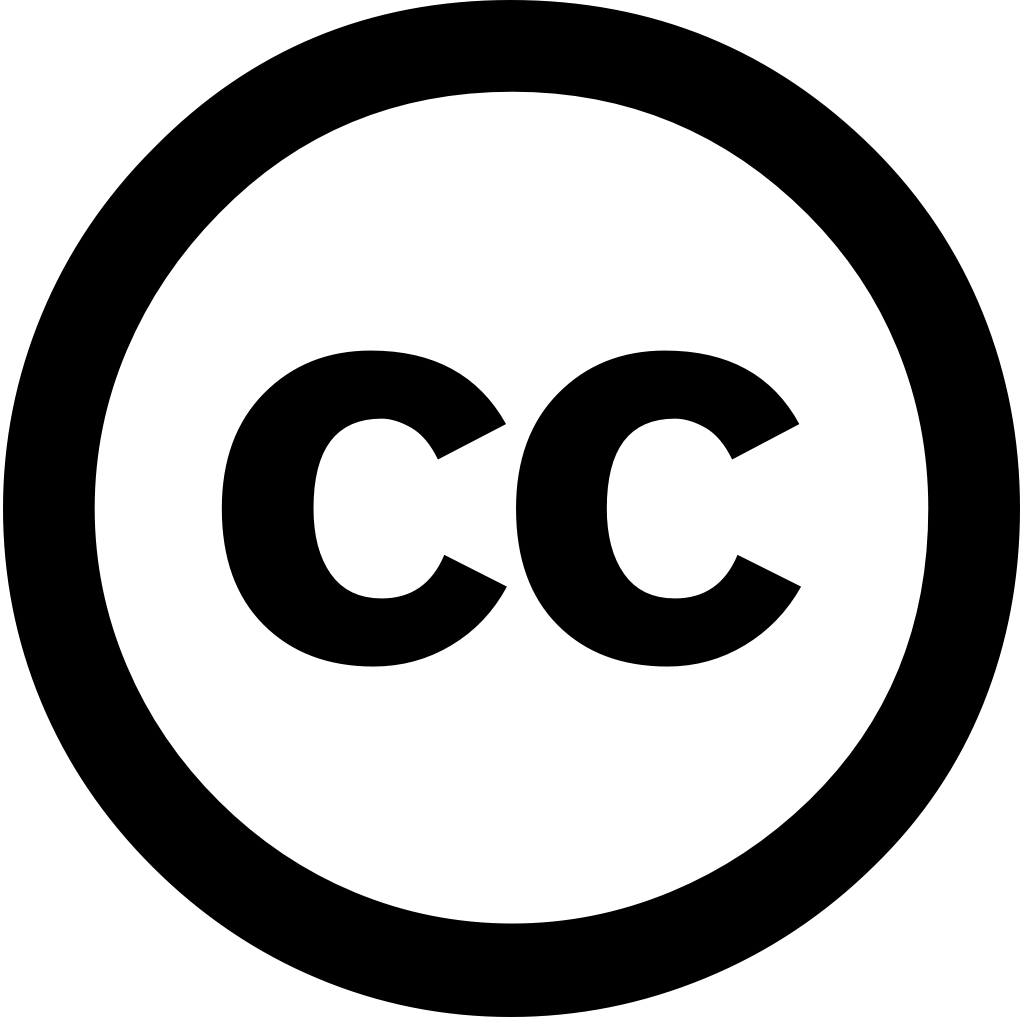
IEEE Access, Journal Year: 2024, Volume and Issue: 13, P. 20374 - 20412
Published: Dec. 23, 2024
Language: Английский
IEEE Access, Journal Year: 2024, Volume and Issue: 13, P. 20374 - 20412
Published: Dec. 23, 2024
Language: Английский
IEEE Access, Journal Year: 2024, Volume and Issue: 12, P. 115750 - 115774
Published: Jan. 1, 2024
Language: Английский
Citations
7International Journal of Intelligent Networks, Journal Year: 2024, Volume and Issue: 5, P. 30 - 37
Published: Jan. 1, 2024
The early detection of brain tumor is crucial for effective treatment and improved patient prognosis in Industrial Information Systems. This research introduces a novel computational model employing three-layer Convolutional Neural Network (CNN) the identification tumors Leveraging advanced techniques, this proposed can autonomously detect intricate patterns features from medical imaging data, resulting more accurate expedited diagnoses. With an impressive 90 % precision rate, our demonstrates potential to serve as valuable tool professionals working field neuroimaging. By presenting dependable precise model, study contributes advancement within domain imaging. We anticipate that methodology will aid healthcare providers making diagnoses, thereby leading enhanced outcomes. Potential avenues future encompass refining model's fundamental architecture exploring real-time therapeutic applications.
Language: Английский
Citations
6Heliyon, Journal Year: 2024, Volume and Issue: 10(2), P. e24188 - e24188
Published: Jan. 1, 2024
Bayesian deep learning (BDL) has emerged as a powerful technique for quantifying uncertainty in classification tasks, surpassing the effectiveness of traditional models by aligning with probabilistic nature real-world data. This alignment allows informed decision-making not only identifying most likely outcome but also surrounding uncertainty. Such capabilities hold great significance fields like medical diagnoses and autonomous driving, where consequences misclassification are substantial. To further improve quantification, research community introduced model ensembles, which combines multiple to enhance predictive accuracy quantification. These ensembles have exhibited superior performance compared individual even non-Bayesian counterparts. In this study, we propose novel approach that leverages power enhanced The proposed method exploits disparity between predicted positive negative classes employes it ranking metric selection. For each instance or sample, ensemble's output class is determined selecting top 'k' based on ranking. Experimental results different image classifications demonstrate consistently outperforms achieves comparable conventional ensemble. investigation highlights practical application ensemble techniques refining enhancing evaluation tasks.
Language: Английский
Citations
5Biomimetics, Journal Year: 2023, Volume and Issue: 8(4), P. 359 - 359
Published: Aug. 11, 2023
Digital twins are computer programs that use real-world data to create simulations predict the performance of processes, products, and systems. may integrate artificial intelligence improve their outputs. Models for dealing with uncertainties noise used accuracy digital twins. Most currently systems aim reduce Nevertheless, biological characterized by inherent variability, which is necessary proper function. The constrained-disorder principle defines living as having a disorder part existence operation while kept within dynamic boundaries. In present paper, we review role in complex its bioengineering. We describe medical applications current methods modeling. paper presents effectiveness twin continuously implementing variability signatures simultaneously reducing unwanted inputs Accounting noisy internal external environments future design improved, more accurate
Language: Английский
Citations
132021 IEEE/CVF International Conference on Computer Vision (ICCV), Journal Year: 2023, Volume and Issue: unknown, P. 21040 - 21049
Published: Oct. 1, 2023
Medical image segmentation is a challenging task, particularly due to inter- and intra-observer variability, even between medical experts. In this paper, we propose novel model, called Probabilistic Inter-Observer iNtra-Observer variation NetwOrk (Pionono). It captures the labeling behavior of each rater with multidimensional probability distribution integrates information feature maps produce probabilistic predictions. The model optimized by variational inference can be trained end-to-end. outperforms state-of-the-art models such as STAPLE, U-Net, based on confusion matrices. Additionally, Pionono predicts multiple coherent that mimic rater's expert opinion, which provides additional valuable for diagnostic process. Experiments real-world cancer datasets demonstrate high accuracy efficiency Pionono, making it powerful tool analysis.
Language: Английский
Citations
10Heliyon, Journal Year: 2025, Volume and Issue: 11(2), P. e41802 - e41802
Published: Jan. 1, 2025
Deep Learning (DL) has significantly contributed to the field of medical imaging in recent years, leading advancements disease diagnosis and treatment. In case Diabetic Retinopathy (DR), DL models have shown high efficacy tasks such as classification, segmentation, detection, prediction. However, model's opacity complexity lead errors decision-making, particularly complex cases, making it necessary estimate uncertainty predictions. Therefore, there is a need predictions, which cannot be estimated by classical alone. To address this issue, Bayesian methods been proposed, their use increasing field. paper, we developed straightforward architecture for classification DR using Convolutional Neural Network (CNN) model. We then applied CNN twice, once Variational Inference (VI) Monte Carlo dropout (MC-dropout) methods, same architecture. This allowed us gain posterior predictive distributions each them. The performance proposed was evaluated on two benchmark datasets, namely APTOS 2019 Messidor-2. Experimental findings demonstrated that surpassed other state-of-the-art models, achieving test accuracy 94.70 % 77.00 CNN, 94.00 86.00 BCNN-VI, 93.30 81.00 BCNN-MC-dropout dataset Messidor-2 dataset, respectively. Finally, computed entropy standard deviation obtained distribution quantify model uncertainty. research highlights potential benefits image analysis improve reliability diagnosing
Language: Английский
Citations
0ACM Transactions on Computing for Healthcare, Journal Year: 2025, Volume and Issue: unknown
Published: Feb. 4, 2025
This paper offers an extensive survey of one the fundamental aspects trustworthiness Artificial Intelligence (AI) in healthcare, namely uncertainty, focusing on large panoply recent studies addressing connection between AI, and healthcare. The concept uncertainty is a recurring theme across multiple disciplines, with varying focuses approaches. Here, we focus diverse nature medical applications, emphasizing importance quantifying model predictions its advantages specific clinical settings. Questions that emerge this context range from guidelines for AI integration healthcare domain to ethical deliberations their compatibility cutting-edge research. Together description main works context, also discuss that, as medicine evolves introduces novel sources there need more versatile quantification methods be developed collaboratively by researchers professionals. Finally, acknowledge limitations current different facets within domain. In particular, identify relative paucity approaches user’s perception accordingly trustworthiness.
Language: Английский
Citations
0Published: Feb. 26, 2025
Language: Английский
Citations
0Metabolites, Journal Year: 2025, Volume and Issue: 15(3), P. 174 - 174
Published: March 3, 2025
Background/Objectives: Metabolomics has recently emerged as a key tool in the biological sciences, offering insights into metabolic pathways and processes. Over last decade, network-based machine learning approaches have gained significant popularity application across various fields. While several studies utilized metabolomics profiles for sample classification, many remain unexplored metabolomic-based classification tasks. This study aims to compare performance of approaches, including developed methods, metabolomics-based classification. Methods: A standard data preprocessing procedure was applied 17 metabolomic datasets, Bayesian neural network (BNN), convolutional (CNN), feedforward (FNN), Kolmogorov-Arnold (KAN), spiking (SNN) were evaluated on each dataset. The datasets varied widely size, mass spectrometry method, response variable. Results: With respect AUC test data, BNN, CNN, FNN, KAN, SNN top-performing models 4, 1, 5, 3, 4 respectively. Regarding F1-score, BNN (3 datasets), CNN FNN (4 KAN datasets). For accuracy, performed best Conclusions: No modeling approach consistently outperformed others metrics AUC, or accuracy. Our results indicate that while no single is superior tasks, may be underappreciated underutilized relative more commonly used FNN.
Language: Английский
Citations
0Published: April 23, 2025
Language: Английский
Citations
0