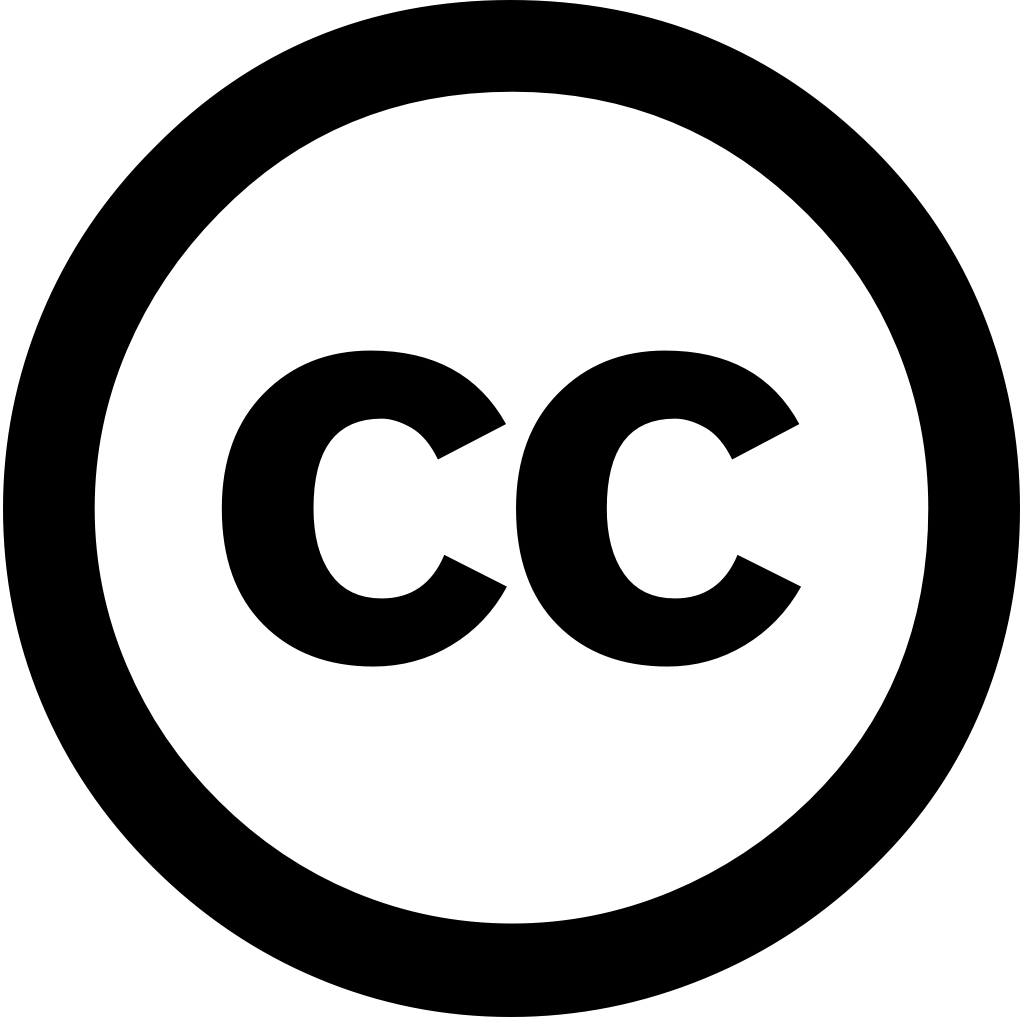
JMIR Formative Research, Journal Year: 2024, Volume and Issue: unknown
Published: June 14, 2024
Language: Английский
JMIR Formative Research, Journal Year: 2024, Volume and Issue: unknown
Published: June 14, 2024
Language: Английский
JMIR Mental Health, Journal Year: 2024, Volume and Issue: 11, P. e57400 - e57400
Published: Sept. 3, 2024
Background Large language models (LLMs) are advanced artificial neural networks trained on extensive datasets to accurately understand and generate natural language. While they have received much attention demonstrated potential in digital health, their application mental particularly clinical settings, has generated considerable debate. Objective This systematic review aims critically assess the use of LLMs specifically focusing applicability efficacy early screening, interventions, settings. By systematically collating assessing evidence from current studies, our work analyzes models, methodologies, data sources, outcomes, thereby highlighting challenges present, prospects for use. Methods Adhering PRISMA (Preferred Reporting Items Systematic Reviews Meta-Analyses) guidelines, this searched 5 open-access databases: MEDLINE (accessed by PubMed), IEEE Xplore, Scopus, JMIR, ACM Digital Library. Keywords used were (mental health OR illness disorder psychiatry) AND (large models). study included articles published between January 1, 2017, April 30, 2024, excluded languages other than English. Results In total, 40 evaluated, including 15 (38%) conditions suicidal ideation detection through text analysis, 7 (18%) as conversational agents, 18 (45%) applications evaluations health. show good effectiveness detecting issues providing accessible, destigmatized eHealth services. However, assessments also indicate that risks associated with might surpass benefits. These include inconsistencies text; production hallucinations; absence a comprehensive, benchmarked ethical framework. Conclusions examines inherent risks. The identifies several issues: lack multilingual annotated experts, concerns regarding accuracy reliability content, interpretability due “black box” nature LLMs, ongoing dilemmas. clear, framework; privacy issues; overreliance both physicians patients, which could compromise traditional medical practices. As result, should not be considered substitutes professional rapid development underscores valuable aids, emphasizing need continued research area. Trial Registration PROSPERO CRD42024508617; https://www.crd.york.ac.uk/prospero/display_record.php?RecordID=508617
Language: Английский
Citations
14Frontiers in Digital Health, Journal Year: 2024, Volume and Issue: 6
Published: June 12, 2024
Prompt engineering, the process of arranging input or prompts given to a large language model guide it in producing desired outputs, is an emerging field research that shapes how these models understand tasks, information, and generate responses wide range natural processing (NLP) applications. Digital mental health, on other hand, becoming increasingly important for several reasons including early detection intervention, mitigate limited availability highly skilled medical staff clinical diagnosis. This short review outlines latest advances prompt engineering NLP digital health. To our knowledge, this first attempt discuss types, methods, tasks are used health We three types tasks: classification, generation, question answering. conclude, we challenges, limitations, ethical considerations, future directions believe contributes useful point departure
Language: Английский
Citations
7Expert Systems with Applications, Journal Year: 2024, Volume and Issue: 255, P. 124855 - 124855
Published: July 24, 2024
Early suicidal ideation detection has long been regarded as an important task that can benefit both society and individuals. In this regard, it shown that, very frequently, the first symptoms of problem be identified by analyzing contents shared on social media. Machine learning classification models have proven promising in capturing behavioral textual features from posts This study proposes a novel machine-learning model to detect risk suicide media posts, employing natural language processing state-of-the-art deep techniques. We propose ensemble LSTM-TCN benefits self-attention mechanism among users two well-known networks, Twitter (X) Reddit. Furthermore, we present comprehensive analysis data, examining statistically semantically, which provide rich knowledge about ideation. Our proposed (AL-BTCN) outperforms compared models, resulting over 94% accuracy, recall, F1-score. Researchers, mental health specialists, service providers all findings study.
Language: Английский
Citations
6Current Opinion in Psychology, Journal Year: 2024, Volume and Issue: 59, P. 101872 - 101872
Published: Aug. 23, 2024
Language: Английский
Citations
5Published: Jan. 1, 2024
Language: Английский
Citations
4Published: March 21, 2025
Effective toxic content detection relies heavily on high-quality and diverse data, which serve as the foundation for robust moderation models. Synthetic data has become a common approach training models across various NLP tasks. However, its effectiveness remains uncertain highly subjective tasks like hate speech detection, with previous research yielding mixed results. This study explores potential of open-source LLMs harmful synthesis, utilizing controlled prompting supervised fine-tuning techniques to enhance quality diversity. We systematically evaluated six open source five datasets, assessing their ability generate diverse, while minimizing hallucination duplication. Our results show that Mistral consistently outperforms other models, significantly enhances reliability further analyze trade-offs between prompt-based vs. fine-tuned discuss real-world deployment challenges, highlight ethical considerations. findings demonstrate provide scalable cost-effective solutions augment paving way more accessible transparent tools.
Language: Английский
Citations
0Published: April 15, 2025
Language: Английский
Citations
0Published: April 22, 2025
Language: Английский
Citations
0Published: April 24, 2025
Language: Английский
Citations
0Big Data and Cognitive Computing, Journal Year: 2025, Volume and Issue: 9(5), P. 116 - 116
Published: April 28, 2025
The decision-making process to rule R&D relies on information related current trends in particular research areas. In this work, we investigated how one can use large language models (LLMs) transfer the dataset and its annotation from another. This is crucial since sharing knowledge between different languages could boost certain underresourced directions target language, saving lots of effort data or quick prototyping. We experiment with English Russian pairs, translating DEFT (Definition Extraction Texts) corpus. corpus contains three layers dedicated term-definition pair mining, which a rare type for Russian. presence such beneficial natural processing methods trend analysis science terms definitions are basic blocks any scientific field. provide pipeline using LLMs. end, train BERT-based translated establish baseline.
Language: Английский
Citations
0