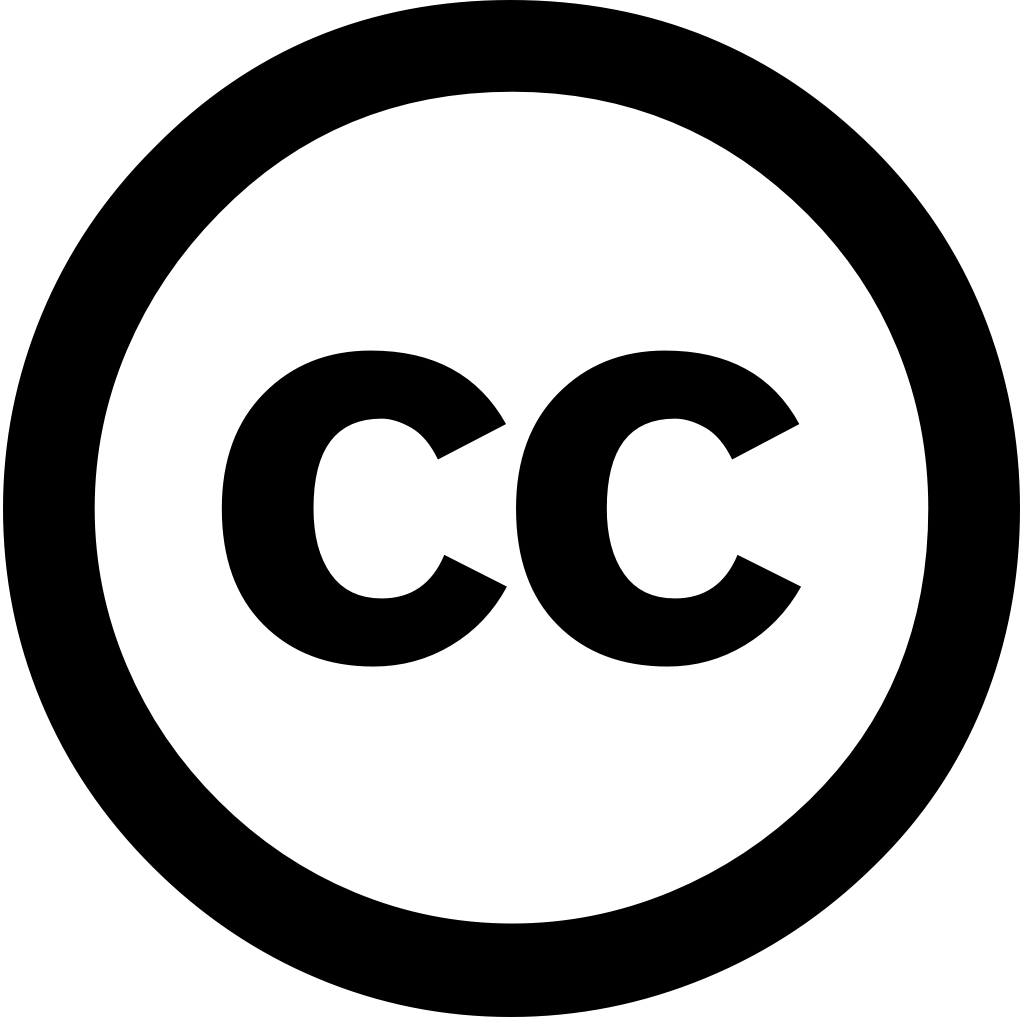
Internet of Things, Journal Year: 2024, Volume and Issue: unknown, P. 101469 - 101469
Published: Dec. 1, 2024
Language: Английский
Internet of Things, Journal Year: 2024, Volume and Issue: unknown, P. 101469 - 101469
Published: Dec. 1, 2024
Language: Английский
IEEE Access, Journal Year: 2024, Volume and Issue: 12, P. 91357 - 91382
Published: Jan. 1, 2024
Language: Английский
Citations
9Machine Learning and Knowledge Extraction, Journal Year: 2025, Volume and Issue: 7(1), P. 12 - 12
Published: Feb. 6, 2025
This study introduces the Pixel-Level Interpretability (PLI) model, a novel framework designed to address critical limitations in medical imaging diagnostics by enhancing model transparency and diagnostic accuracy. The primary objective is evaluate PLI’s performance against Gradient-Weighted Class Activation Mapping (Grad-CAM) achieve fine-grained interpretability improved localization precision. methodology leverages VGG19 convolutional neural network architecture utilizes three publicly available COVID-19 chest radiograph datasets, consisting of over 1000 labeled images, which were preprocessed through resizing, normalization, augmentation ensure robustness generalizability. experiments focused on key metrics, including interpretability, structural similarity (SSIM), precision, mean squared error (MSE), computational efficiency. results demonstrate that PLI significantly outperforms Grad-CAM all measured dimensions. produced detailed pixel-level heatmaps with higher SSIM scores, reduced MSE, faster inference times, showcasing its ability provide granular insights into localized features while maintaining In contrast, Grad-CAM’s explanations often lack granularity required for clinical reliability. By integrating fuzzy logic enhance visual numerical explanations, can deliver interpretable outputs align expectations, enabling practitioners make informed decisions confidence. work establishes as robust tool bridging gaps AI usability. addressing challenges accuracy simultaneously, contributes advancing integration healthcare sets foundation broader applications other high-stake domains.
Language: Английский
Citations
0Frontiers in Oncology, Journal Year: 2025, Volume and Issue: 15
Published: March 26, 2025
Introduction The early identification of brain tumors is essential for optimal treatment and patient prognosis. Advancements in MRI technology have markedly enhanced tumor detection yet necessitate accurate classification appropriate therapeutic approaches. This underscores the necessity sophisticated diagnostic instruments that are precise comprehensible to healthcare practitioners. Methods Our research presents CNN-TumorNet, a convolutional neural network categorizing images into non-tumor categories. Although deep learning models exhibit great accuracy, their complexity frequently restricts clinical application due inadequate interpretability. To address this, we employed LIME technique, augmenting model transparency offering explicit insights its decision-making process. Results CNN-TumorNet attained 99% accuracy rate differentiating from scans, underscoring reliability efficacy as instrument. Incorporating guarantees model’s judgments comprehensible, enhancing adoption. Discussion Despite overarching challenge interpretability persists. These may function ”black boxes,” complicating doctors’ ability trust accept them without comprehending rationale. By integrating LIME, achieves elevated alongside transparency, facilitating environments improving care neuro-oncology.
Language: Английский
Citations
0Internet of Things, Journal Year: 2024, Volume and Issue: unknown, P. 101469 - 101469
Published: Dec. 1, 2024
Language: Английский
Citations
0