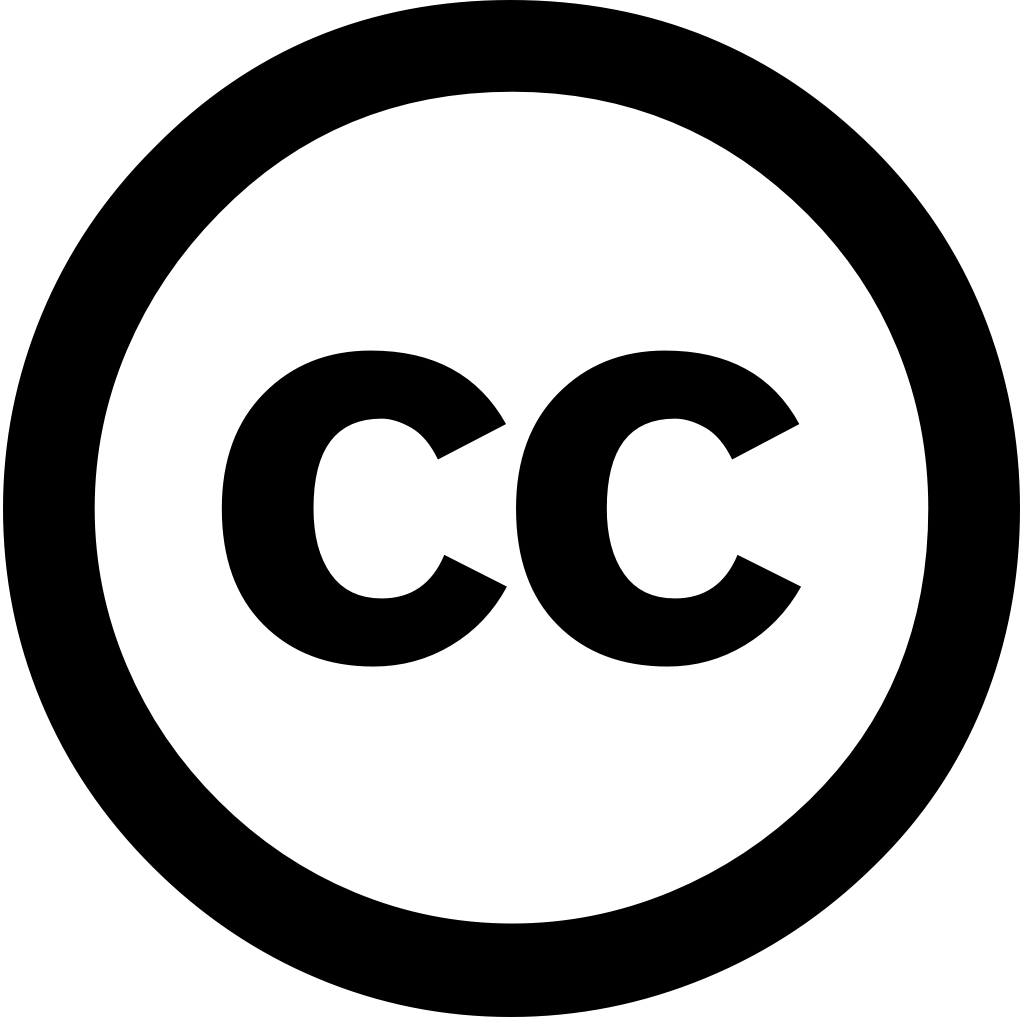
Sensors, Journal Year: 2025, Volume and Issue: 25(3), P. 782 - 782
Published: Jan. 28, 2025
Accurately estimating soil moisture at multiple depths is essential for sustainable farming practices, as it supports efficient irrigation management, optimizes crop yields, and conserves water resources. This study integrates a drone-mounted hyperspectral sensor with machine learning techniques to enhance estimation 10 cm 30 in cornfield. The primary aim was understand the relationship between root zone content canopy reflectance, pinpoint where this most significant, identify informative wavelengths, train model using those wavelengths estimate moisture. Our results demonstrate that PCA effectively detected critical variables estimation, ANN outperforming other algorithms, including Random Forest (RF), Support Vector Regression (SVR), Gradient Boosting (XGBoost). Model comparisons irrigated non-irrigated treatments showed plots could be estimated greater accuracy across various dates. finding indicates plants experiencing high stress exhibit more significant spectral variability their canopy, enhancing correlation zone. Moreover, over growing season, when corn exhibits chlorophyll increased resilience environmental stressors, spectrum weakens. Error analysis revealed lowest relative errors depth, aligning periods of elevated shallower levels, which drove deeper growth strengthened reflectance relationship. corresponded lower RMSE values, highlighting improved accuracy.
Language: Английский