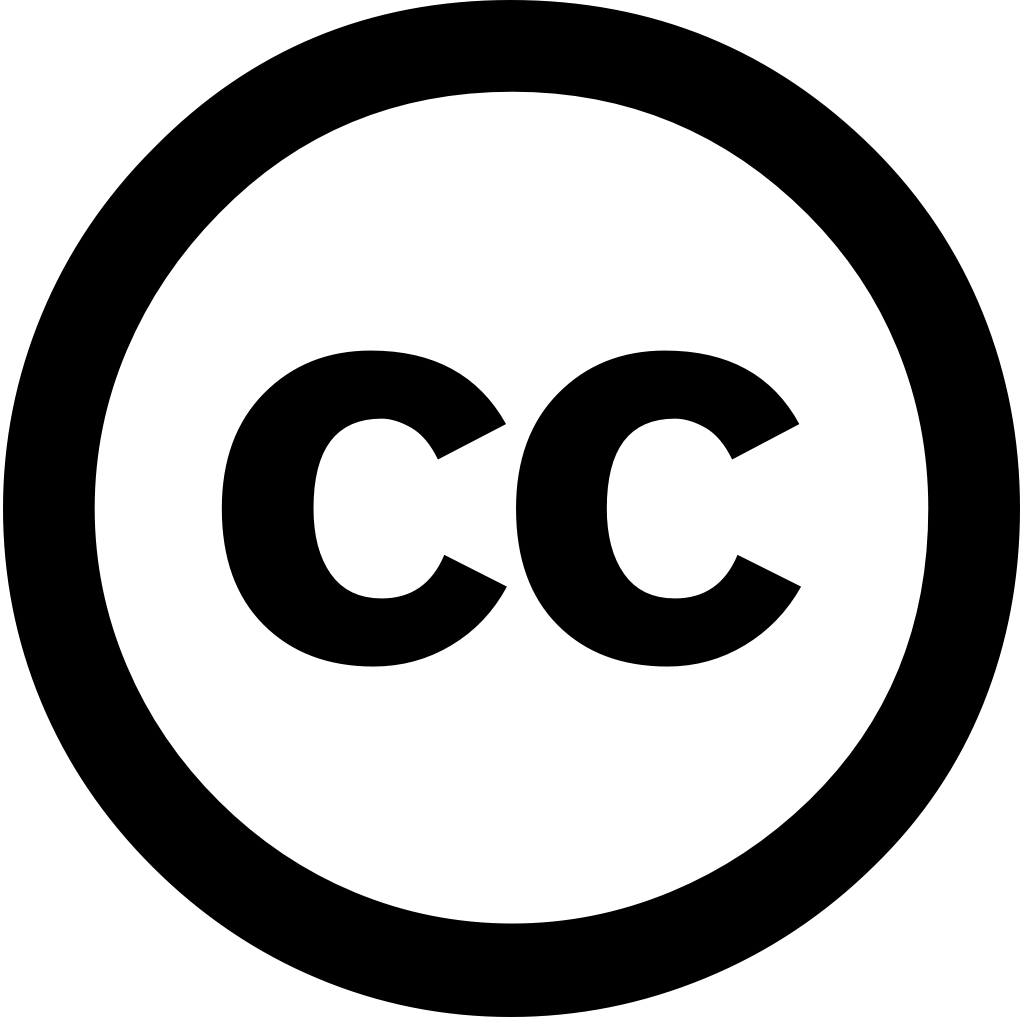
Applied Artificial Intelligence, Journal Year: 2024, Volume and Issue: 38(1)
Published: Jan. 10, 2024
Severe wind shear events near airport runways pose serious safety risks and are a growing concern in civil aviation. Identification of severe risk factors may enhance aviation safety. Although rare, impacts by affecting the airspeed, lift, maneuverability aircraft. This study presents TabNet, novel deep learning technique coupled with Bayesian optimization (BO) to predict severity runway vicinity using Doppler LiDAR data from Hong Kong International Airport. To address imbalanced data, it was first processed resampling techniques then used as input TabNet. The analysis demonstrated that Bayesian-tuned TabNet (BO-TabNet) SVM-SMOTE-processed led better performance compared other strategies. architecture employs attention mechanism enable model-specific interpretability. Analysis showed most important contributing factor summer season, followed encounter location (1 nautical miles at departure end). Additionally, more comprehensive model-agnostic LIME method elucidate model local perspective. By predicting assessing factors, stakeholders can proactively manage mitigate associated risks, leading safer efficient operations.
Language: Английский