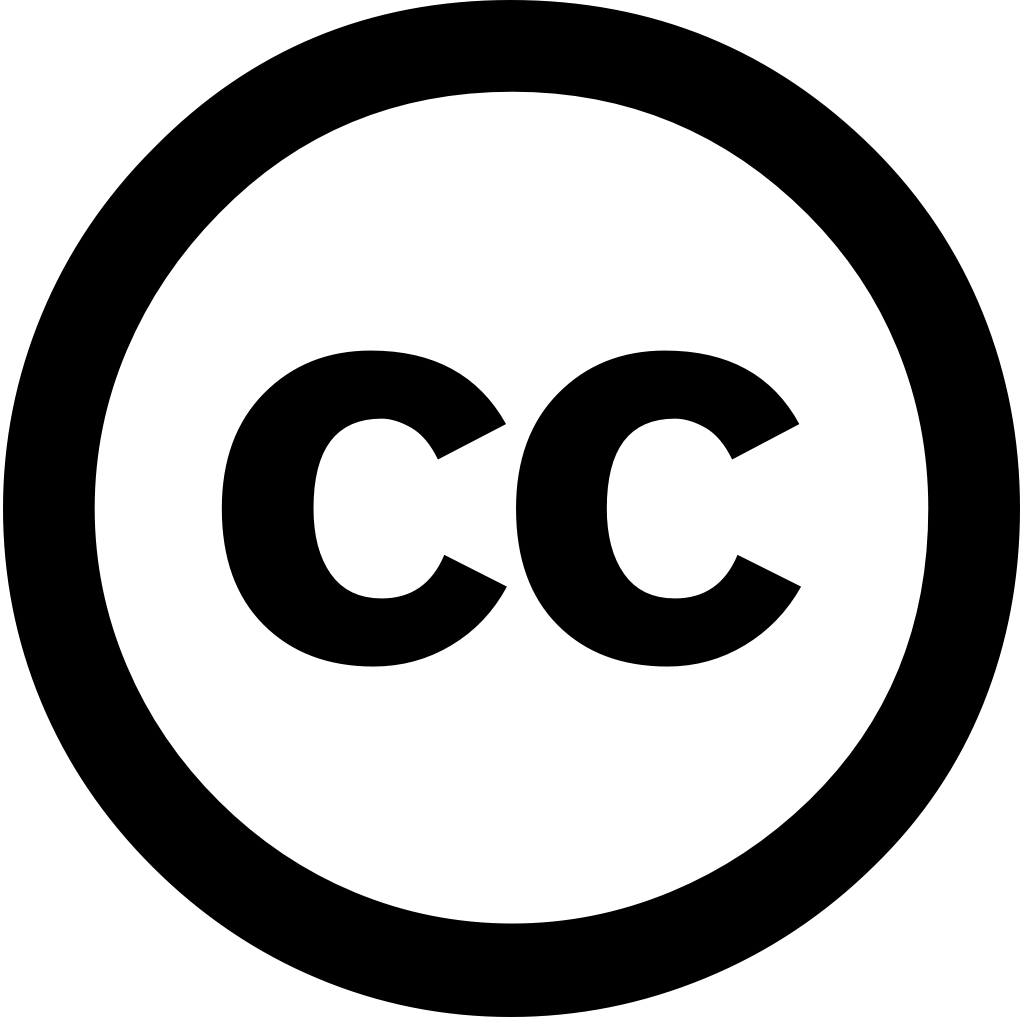
Research, Journal Year: 2024, Volume and Issue: 7
Published: Jan. 1, 2024
Problem: Chest radiography is a crucial tool for diagnosing thoracic disorders, but interpretation errors and lack of qualified practitioners can cause delays in treatment. Aim: This study aimed to develop reliable multi-classification artificial intelligence (AI) improve the accuracy efficiency chest radiograph diagnosis. Methods: We developed convolutional neural network (CNN) capable distinguishing among 26 diagnoses. The model was trained externally validated using 795,055 radiographs from 13 datasets across 4 countries. Results: CNN achieved an average area under curve (AUC) 0.961 all diagnoses testing set. COVID-19 detection perfect (AUC 1.000, [95% confidence interval {CI}, 1.000 1.000]), while effusion or pleural showed lowest 0.8453, CI, 0.8417 0.8489]). In external validation, demonstrated strong reproducibility generalizability within local dataset, achieving AUC 0.9634 lung opacity (95% 0.9423 0.9702). outperformed both radiologists nonradiological physicians, particularly trans-device image recognition. Even diseases not specifically on, such as aortic dissection, AI considerable scalability enhanced diagnostic physicians varying experience levels (all P < 0.05). Additionally, our exhibited no gender bias ( > Conclusion: algorithm, now available professional web-based software, substantively improves interpretation. research advances medical imaging offers substantial support clinical settings.
Language: Английский