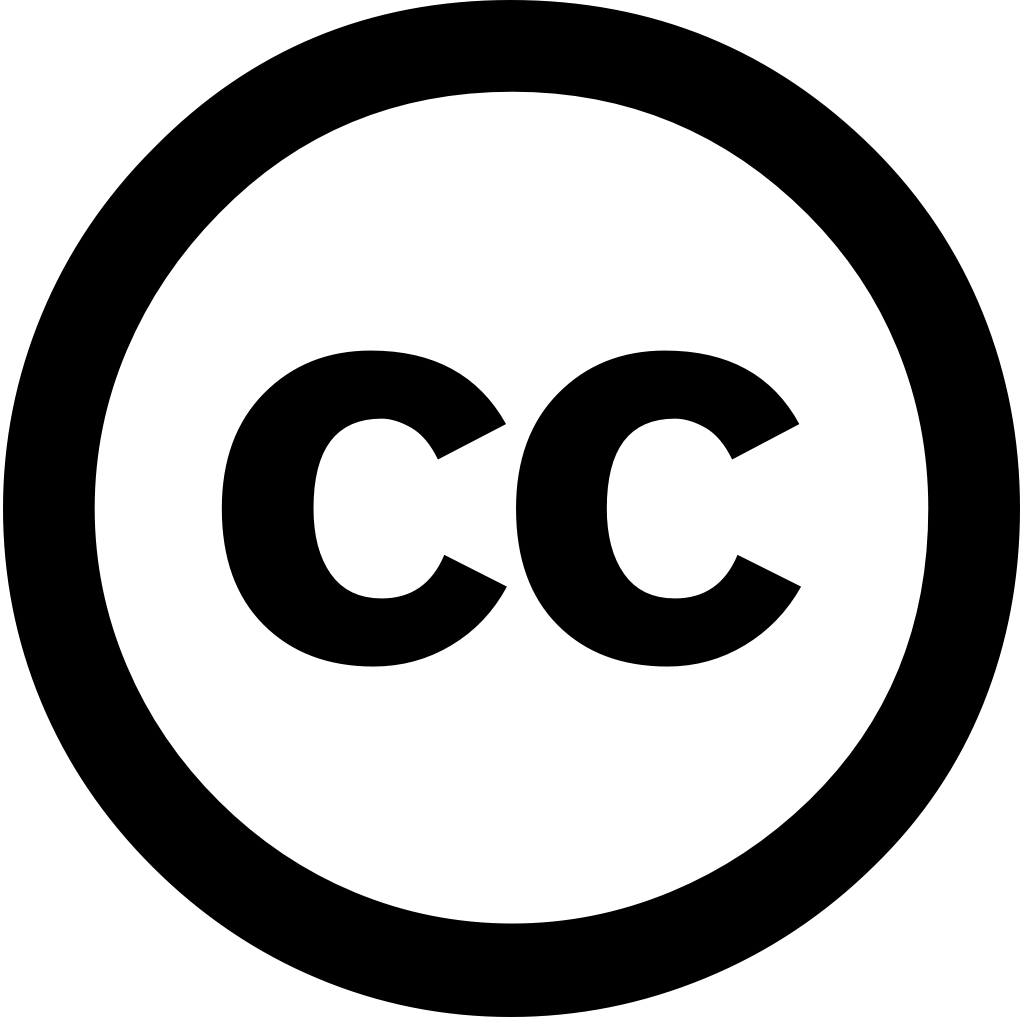
Published: Jan. 1, 2024
Language: Английский
Published: Jan. 1, 2024
Language: Английский
2021 IEEE/CVF International Conference on Computer Vision (ICCV), Journal Year: 2023, Volume and Issue: unknown, P. 6532 - 6542
Published: Oct. 1, 2023
Spiking neural networks (SNNs) are brain-inspired energy-efficient models that encode information in spatiotemporal dynamics. Recently, deep SNNs trained directly have shown great success achieving high performance on classification tasks with very few time steps. However, how to design a directly-trained SNN for the regression task of object detection still remains challenging problem. To address this problem, we propose EMS-YOLO, novel framework detection, which is first trial train surrogate gradients rather than ANN-SNN conversion strategies. Specifically, full-spike residual block, EMS-ResNet, can effectively extend depth low power consumption. Furthermore, theoretically analyze and prove EMS-ResNet could avoid gradient vanishing or exploding. The results demonstrate our approach outperforms state-of-the-art methods (at least 500 steps) extremely fewer steps (only 4 steps). It model achieve comparable ANN same architecture while consuming 5.83× less energy frame-based COCO Dataset event-based Gen1 Dataset. Our code available https://github.com/BICLab/EMS-YOLO.
Language: Английский
Citations
24APL Materials, Journal Year: 2024, Volume and Issue: 12(10)
Published: Oct. 1, 2024
Language: Английский
Citations
10ACS Applied Electronic Materials, Journal Year: 2025, Volume and Issue: unknown
Published: Feb. 14, 2025
A brain-inspired computing paradigm known as "neuromorphic computing" seeks to replicate the information processing processes of biological neural systems in order create that are effective, low-power, and adaptable. Spiking networks (SNNs) based on a single device at forefront computing, which aims mimic powers human brain. Neuromorphic devices, enable hardware implementation artificial (ANNs), heart neuromorphic computing. These devices dynamics functions neurons synapses. This mini-review assesses latest advancements with an emphasis small, energy-efficient synapses neurons. Key like spike-timing-dependent plasticity, multistate storage, dynamic filtering demonstrated by variety single-device models, such memristors, transistors, magnetic ferroelectric devices. The integrate-and-fire (IF) neuron is key model these because it allows for mathematical analysis while successfully capturing aspects processing. review examines potential SNNs scalable, low-power applications, highlighting both benefits constraints implementing them architectures. highlights increasing importance creation flexible cognitive
Language: Английский
Citations
1IEEE Transactions on Cognitive and Developmental Systems, Journal Year: 2023, Volume and Issue: 16(2), P. 544 - 558
Published: May 26, 2023
Spiking neural networks (SNNs) have demonstrated excellent capabilities in various intelligent scenarios. Most existing methods for training SNNs are based on the concept of synaptic plasticity; however, learning realistic brain also utilizes intrinsic non-synaptic mechanisms neurons. The spike threshold biological neurons is a critical neuronal feature that exhibits rich dynamics millisecond timescale and has been proposed as an underlying mechanism facilitates information processing. In this study, we develop novel synergistic approach involves simultaneously weights thresholds SNNs. trained with synapse-threshold (STL-SNNs) achieve significantly superior performance static neuromorphic datasets than two degenerated single-learning models. During training, optimizes thresholds, providing network stable signal transmission via appropriate firing rates. Further analysis indicates STL-SNNs robust to noisy data exhibit low energy consumption deep structures. Additionally, STL-SNN can be further improved by introducing generalized joint decision framework. Overall, our findings indicate biologically plausible synergies between may provide promising developing highly efficient SNN methods.
Language: Английский
Citations
122021 IEEE/CVF International Conference on Computer Vision (ICCV), Journal Year: 2023, Volume and Issue: unknown, P. 16878 - 16888
Published: Oct. 1, 2023
Spiking Neural Networks (SNNs) are well known as a promising energy-efficient alternative to conventional artificial neural networks. Subject the preconceived impression that SNNs sparse firing, analysis and optimization of inherent redundancy in have been largely overlooked, thus potential advantages spike-based neuromorphic computing accuracy energy efficiency interfered. In this work, we pose focus on three key questions regarding SNNs. We argue is induced by spatio-temporal invariance SNNs, which enhances parameter utilization but also invites lots noise spikes. Further, analyze effect dynamics spike firing Then, motivated these analyses, propose an Advance Spatial Attention (ASA) module harness SNNs' redundancy, can adaptively optimize their membrane distribution pair individual spatial attention sub-modules. way, features accurately regulated. Experimental results demonstrate proposed method significantly drop with better performance than state-of-the-art SNN baselines. Our code available https://github.com/BICLab/ASA-SNN.
Language: Английский
Citations
11Frontiers in Neuroscience, Journal Year: 2025, Volume and Issue: 19
Published: March 12, 2025
Introduction Spiking Neural Networks (SNNs) offer a biologically inspired alternative to conventional artificial neural networks, with potential advantages in power efficiency due their event-driven computation. Despite promise, SNNs have yet achieve competitive performance on complex visual tasks, such as image classification. Methods This study introduces novel SNN architecture called SpikeAtConv, designed enhance computational efficacy and task accuracy. The features optimized spiking modules that facilitate the processing of spatio-temporal patterns data, aiming reconcile demands high-level vision tasks energy-efficient SNNs. Results Extensive experiments show proposed SpikeAtConv outperforms or is comparable state-of-the-art datasets. Notably, we achieved top-1 accuracy 81.23% ImageNet-1K using directly trained Large which result field SNN. Discussion Our evaluations standard classification benchmarks indicate narrows gap traditional providing insights into design more efficient capable neuromorphic computing systems.
Language: Английский
Citations
0Neural Networks, Journal Year: 2025, Volume and Issue: unknown, P. 107395 - 107395
Published: March 1, 2025
Language: Английский
Citations
0Neural Networks, Journal Year: 2024, Volume and Issue: 176, P. 106330 - 106330
Published: April 21, 2024
Language: Английский
Citations
1Neural Networks, Journal Year: 2024, Volume and Issue: 184, P. 107043 - 107043
Published: Dec. 16, 2024
Language: Английский
Citations
1Neural Networks, Journal Year: 2024, Volume and Issue: 180, P. 106630 - 106630
Published: Aug. 22, 2024
Language: Английский
Citations
0