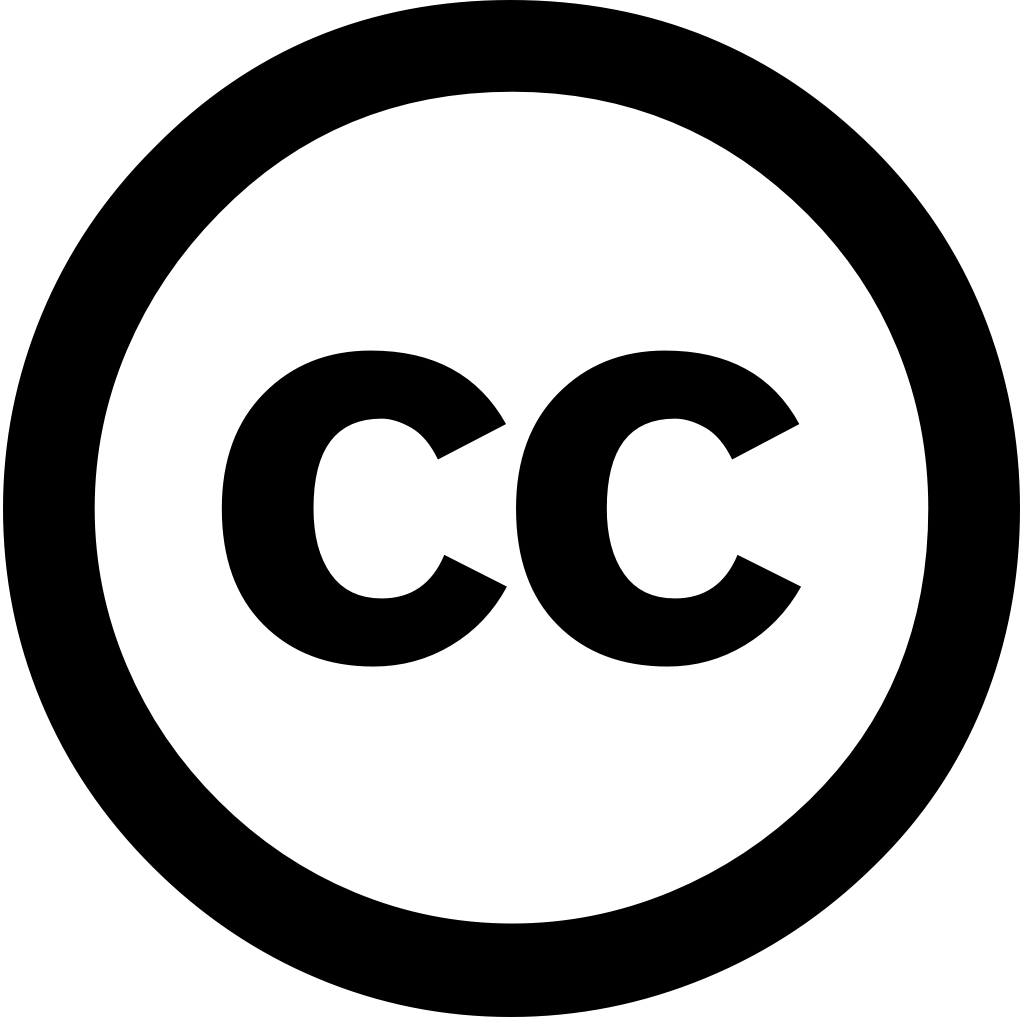
Research Square (Research Square), Journal Year: 2024, Volume and Issue: unknown
Published: April 17, 2024
Language: Английский
Research Square (Research Square), Journal Year: 2024, Volume and Issue: unknown
Published: April 17, 2024
Language: Английский
Electronics, Journal Year: 2022, Volume and Issue: 11(23), P. 3915 - 3915
Published: Nov. 26, 2022
Electromyography is a diagnostic medical procedure used to assess the state of muscle and its related nerves. signals are monitored detect neuromuscular abnormalities diseases but can also prove useful in decoding movement-related signals. This information vital controlling prosthetics more natural way. To this end, novel analog integrated voting classifier proposed as hand gesture recognition system. The classifiers utilize 3 separate centroid-based classifiers, each one attached different electromyographic electrode circuit. main building blocks architecture bump winner-take-all circuits. confirm proper operation classifier, post-layout classification results (91.2% accuracy) compared software-based implementation (93.8% same classifier. A TSMC 90 nm CMOS process Cadence IC Suite was design simulate following circuits architectures.
Language: Английский
Citations
13IEEE Access, Journal Year: 2023, Volume and Issue: 11, P. 96797 - 96807
Published: Jan. 1, 2023
The use of hand gestures for human-computer interaction (HCI) has gained popularity due to its ability provide natural and intuitive communication in human dialogues. Hand gesture recognition (HGR) using surface electromyography (sEMG) signals is more reliable user-friendly than traditional computer vision-based methods. This study proposes a deep network named DeepTPA-Net that utilizes multi-channel sEMG recognize gestures. employs ResNet50 as an automated feature extractor novel triple attention (3Attn) block connects spatial, temporal, channel modules parallel signify important features HGR. We evaluated the performance five publicly available benchmark datasets, including CapgMyo DB-a, Csl-hdemg, NinaPro DB1, DB2, SeNic. effectiveness proposed 3Attn HGR demonstrated through comparison with other mechanisms. compared various baseline models, variations existing results show significantly outperforms models all indicating superiority sEMG-based
Language: Английский
Citations
82022 IEEE International Symposium on Circuits and Systems (ISCAS), Journal Year: 2023, Volume and Issue: unknown, P. 1 - 5
Published: May 21, 2023
End-to-end event-based computation has the poten-tial to push envelope in latency and energy efficiency for edge AI applications. Unfortunately, sensors (e.g., DVS cameras) neuromorphic spike-based processors Loihi) have been designed a decoupled fashion, thereby missing major streamlining opportunities. This paper presents ColibriES, first-ever hardware embedded system plat-form with dedicated event-sensor interfaces full processing pipelines. ColibriES includes event frame data processing, aiming at efficient long-life systems scenarios. is based on Kraken system-on-chip contains heterogeneous parallel ultra-low power (PULP) processor, frame-based camera interfaces, two accelerators of both spiking neural networks ternary convolutional networks. explores accurately evaluates performance example gesture recognition as first step full-system evaluation. In our experiments, we demonstrate chip consumption 7.7 mJ 164.5 ms each inference Gesture set an closed-loop showcasing potential battery-powered applications such wearable devices UAVs that require low-latency control.
Language: Английский
Citations
7Journal of Neural Engineering, Journal Year: 2024, Volume and Issue: 21(3), P. 036031 - 036031
Published: May 16, 2024
Upper limb loss can profoundly impact an individual's quality of life, posing challenges to both physical capabilities and emotional well-being. To restore function by decoding electromyography (EMG) signals, in this paper, we present a novel deep prototype learning method for accurate generalizable EMG-based gesture classification. Existing methods suffer from limitations generalization across subjects due the diverse nature individual muscle responses, impeding seamless applicability broader populations.
Language: Английский
Citations
2Lecture notes in computer science, Journal Year: 2024, Volume and Issue: unknown, P. 15 - 26
Published: Jan. 1, 2024
Language: Английский
Citations
2IEEE Access, Journal Year: 2024, Volume and Issue: 12, P. 40439 - 40449
Published: Jan. 1, 2024
Motion intent recognition for controlling prosthetic systems has long relied on machine learning algorithms. Artificial neural networks have shown great promise solving such nonlinear classification tasks, making them a viable method this purpose. To bring these advanced methods and algorithms beyond the confines of laboratory into daily lives users, self-contained embedded are essential. However, face constraints in size, computational power, memory footprint, power consumption, as they must be non-intrusive discreetly integrated commercial components. One promising approach to tackle challenges is use network quantization, which allows complying with limitations without significant loss accuracy. Here, we compare quantization performance using TensorFlow Lite recently developed QKeras platform. Due internal libraries, led 8 times higher flash usage than that unquantized reference network, disadvantageous systems. In response, offer open-source code solutions leverage platform, effectively reducing requirements by 24 compared Tensorflow Lite. Additionally, conducted comprehensive comparison state-of-the-art microcontrollers. Our results reveal adoption new architectures offers substantial reductions inference time consumption. These improvements pave way real-time decoding motor more life usage, possibly enabling reliable precise control users.
Language: Английский
Citations
1Published: June 20, 2024
Language: Английский
Citations
1Published: July 11, 2024
Language: Английский
Citations
1IEEE Transactions on Biomedical Circuits and Systems, Journal Year: 2024, Volume and Issue: 19(1), P. 68 - 81
Published: Sept. 9, 2024
The accurate modeling of hand movement based on the analysis surface electromyographic (sEMG) signals offers exciting opportunities for development complex prosthetic devices and human-machine interfaces, moving from discrete gesture recognition, towards continuous tracking. In this study, we present two solutions real-time sEMG processing, lightweight Spiking Neural Networks (SNNs) efficiently implemented a Lattice iCE40-UltraPlus FPGA, especially suitable low-power applications. We first assess performance in finger recognition task, considering as reference NinaPro DB5 dataset, demonstrating an accuracy 83.17% classification twelve different gestures. also consider more challenging problem force modeling, referencing Hyser dataset tracking during independent extension contraction exercises. assessment reveals correlation up to 0.875 with ground-truth forces. Our systems take advantage SNNs' inherent efficiency and, dissipating 11.31 mW active mode, consume 44.6 µJ 1.19 inference. Considering dynamic power-consumption management introduction idle periods, average power drops 1.84 3.69 these respective tasks.
Language: Английский
Citations
1Scientific Reports, Journal Year: 2024, Volume and Issue: 14(1)
Published: Dec. 28, 2024
Surface electromyography (sEMG) data has been extensively utilized in deep learning algorithms for hand movement classification. This paper aims to introduce a novel method gesture classification using sEMG data, addressing accuracy challenges seen previous studies. We propose U-Net architecture incorporating MobileNetV2 encoder, enhanced by Bidirectional Long Short-Term Memory (BiLSTM) and metaheuristic optimization spatial feature extraction motion recognition. Bayesian is employed as the approach optimize BiLSTM model's architecture. To address non-stationarity of signals, we employ windowing strategy signal augmentation within architectures. The encoder extract relevant features from spectrogram images. Edge computing integration leveraged further enhance innovation enabling real-time processing decision-making closer source. Six standard databases were utilized, achieving an average 90.23% with our proposed model, showcasing 3–4% improvement 10% variance reduction. Notably, Mendeley Data, BioPatRec DB3, DB1 surpassed advanced models their respective domains accuracies 88.71%, 90.2%, 88.6%, respectively. Experimental results underscore significant enhancement generalizability recognition robustness. offers fresh perspective on prosthetic management human-machine interaction, emphasizing its efficacy improving reducing control interaction machines through edge integration.
Language: Английский
Citations
1