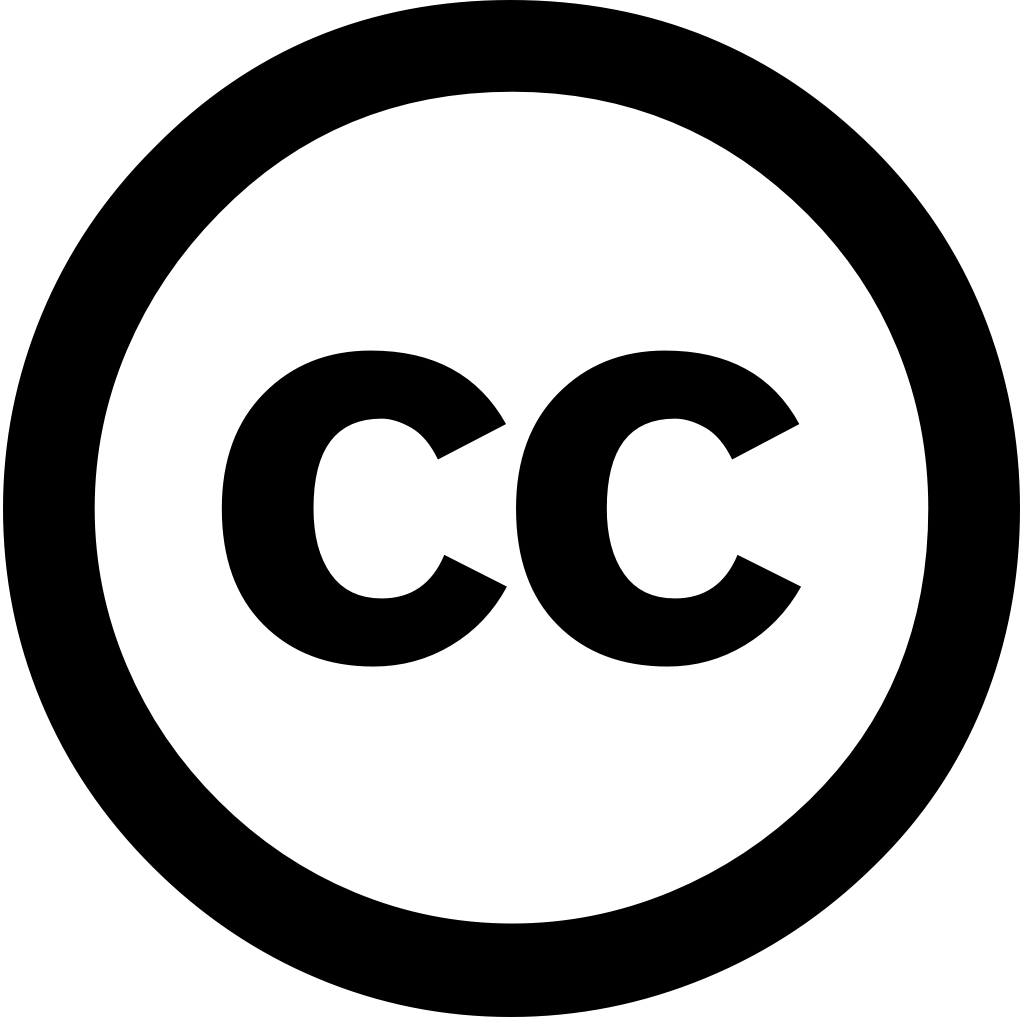
Research Square (Research Square), Journal Year: 2024, Volume and Issue: unknown
Published: April 17, 2024
Language: Английский
Research Square (Research Square), Journal Year: 2024, Volume and Issue: unknown
Published: April 17, 2024
Language: Английский
2022 IEEE Biomedical Circuits and Systems Conference (BioCAS), Journal Year: 2023, Volume and Issue: 30, P. 1 - 6
Published: Oct. 19, 2023
Fast and accurate online processing is essential for smooth prosthetic hand control with Surface Electromyography signals (sEMG). Although transformers are state-of-the-art deep learning models in signal processing, the self-attention mechanism at core of their operations requires accumulating data large time-windows. They therefore not suited processing. In this paper, we use an attention sliding windows that allows a transformer to process sequences element-by-element. Moreover, increase sparsity network using spiking neurons. We test model on NinaproDB8 finger position regression dataset. Our sets its new terms accuracy NinaproDB8, while requiring only very short time 3.5 ms each inference step, reducing number synaptic up factor ×5.3 thanks results hold great promises wearable sEMG systems control.
Language: Английский
Citations
3Published: Oct. 3, 2023
Energy efficiency and low latency are crucial requirements for designing wearable AI-empowered human activity recognition systems, due to the hard constraints of battery operations closed-loop feedback. While neural network models have been extensively compressed match stringent edge requirements, spiking networks event-based sensing recently emerging as promising solutions further improve performance their inherent energy capacity process spatiotemporal data in very latency. This work aims evaluate effectiveness on neuromorphic processors applications. The case workout with wrist-worn motion sensors is used a study. A multi-threshold delta modulation approach utilized encoding input sensor into spike trains move pipeline approach. spikes then fed direct-event training, trained model deployed research platform from Intel, Loihi, efficiency. Test results show that spike-based workouts system can achieve comparable accuracy (87.5\%) popular milliwatt RISC-V bases multi-core processor GAP8 traditional ( 88.1\%) while achieving two times better energy-delay product (0.66 \si{\micro\joule\second} vs. 1.32 \si{\micro\joule\second}).
Language: Английский
Citations
22022 IEEE Biomedical Circuits and Systems Conference (BioCAS), Journal Year: 2023, Volume and Issue: unknown, P. 1 - 5
Published: Oct. 19, 2023
Neuromorphic processors that implement Spiking Neural Networks (SNNs) using mixed-signal analog/digital circuits represent a promising technology for closed-loop real-time processing of biosignals. As in biology, to minimize power consumption, the silicon neurons' are configured fire with limited dynamic range and maximum firing rates restricted few tens or hundreds Herz. However, biosignals can have very large range, so encoding them into spikes without saturating neuron outputs represents an open challenge. In this work, we present biologically-inspired strategy compressing high-dynamic SNN architectures, three adaptation mechanisms ubiquitous brain: spike-frequency at single level, feed-forward inhibitory connections from neurons belonging input layer, Excitatory-Inhibitory (E-I) balance via recurrent inhibition among output layer. We apply encoded both asynchronous delta modulation method energy-based pulse-frequency method. validate approach silico, simulating simple network applied gesture classification task surface EMG recordings.
Language: Английский
Citations
2IEEE Sensors Journal, Journal Year: 2024, Volume and Issue: 24(8), P. 13640 - 13655
Published: March 6, 2024
Using surface electromyography (sEMG) signals for gesture recognition can significantly improve the effects of recognition. Therefore, this article proposed a CNN-SE-LSTM-TCN feature fusion network (FFCSLT) traffic police based on characteristics sEMG signals. First, an acquisition system with six-channel sensors was developed acquiring during human movement, and dataset hand gestures (TPG) constructed, which contains total 36000 sets data. Then, squeeze-and-excitation (SE) block adaptive channel weighting added top depthwise separable convolutional (DSCN) to enhance spatial features between each in FFCSLT network. Meanwhile, temporal (TCN) integrated into long short-term memory (LSTM) extract additional Finally, comparing experiments other methods were taken two datasets: self-collected TPG dataset, widely used Sensor Data Ninapro DB1. The experimental results show that our model has accuracy 98.89% 96.52% DB1 is 2.22% 0.75% higher than suboptimal methods, respectively. To further validate network, we also performed variety ablation studies.
Language: Английский
Citations
0Research Square (Research Square), Journal Year: 2024, Volume and Issue: unknown
Published: April 17, 2024
Language: Английский
Citations
0