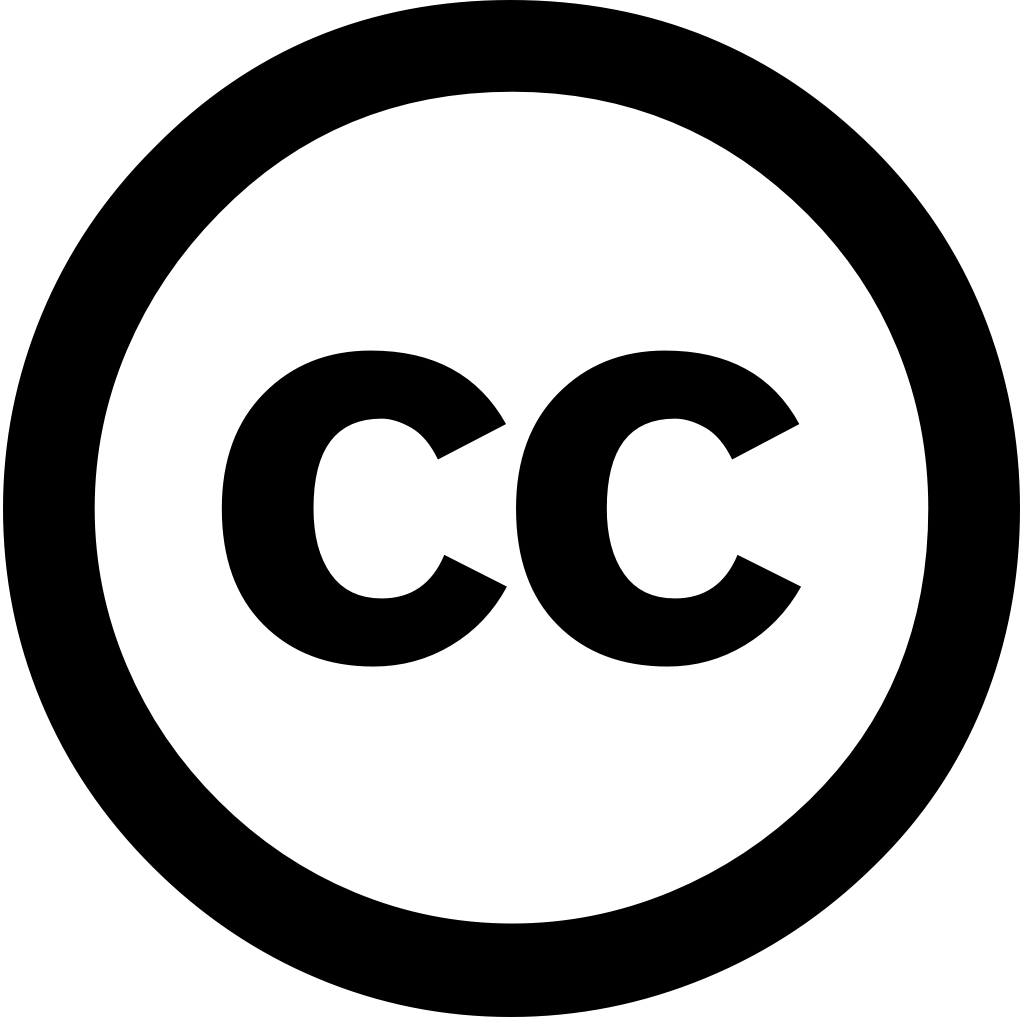
PLoS ONE, Journal Year: 2024, Volume and Issue: 19(11), P. e0310035 - e0310035
Published: Nov. 4, 2024
This study aims to develop a cost-effective and reliable motion monitoring device capable of comprehensive fatigue analysis. It achieves this objective by integrating surface electromyography (sEMG) accelerometer (ACC) signals through feature fusion strategy. The introduces multi-level attention mechanism for classification, leveraging convolutional neural networks (CNNs). preprocessing phase involves local that enhances waveform features using the amplitude envelope. A dual-scale mechanism, operating at both channel neuron levels, is employed enhance model’s learning from high-dimensional fused data, improving extraction generalization. significantly improves classification accuracy convergence, as demonstrated in ablation experiments. model, optimized with mechanisms, excels generalization, particularly handling data pseudo-artifacts. Computational analysis indicates proposed optimization algorithm has minimal impact on CNN’s training testing times. recognition accuracies 92.52%, 92.38%, 92.30%, well F1-scores 91.92%, 92.13%, 92.29% three states, affirming its reliability. research provides technical support development affordable dependable wearable devices.
Language: Английский