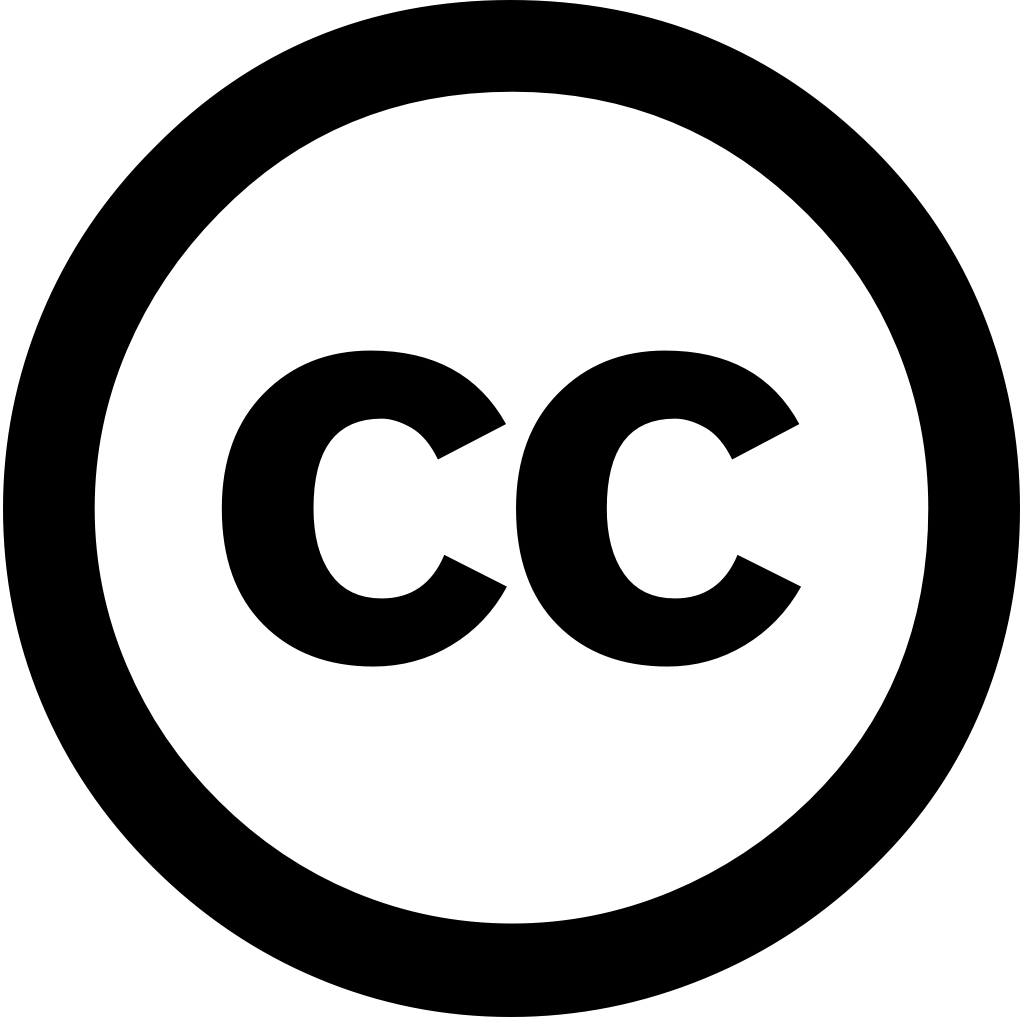
Egyptian Informatics Journal, Journal Year: 2024, Volume and Issue: 27, P. 100509 - 100509
Published: July 30, 2024
Language: Английский
Egyptian Informatics Journal, Journal Year: 2024, Volume and Issue: 27, P. 100509 - 100509
Published: July 30, 2024
Language: Английский
Swarm and Evolutionary Computation, Journal Year: 2025, Volume and Issue: 93, P. 101849 - 101849
Published: Jan. 23, 2025
Language: Английский
Citations
0Scientific Reports, Journal Year: 2025, Volume and Issue: 15(1)
Published: March 20, 2025
The hybrid flowshop scheduling problem (HFSP), a typical NP-hard problem, has gained significant interest from researchers focusing on the development of solution methods. We focus variable speed problem. assume that machines operate at when processing workpieces, making more reflective real-world scenarios. Aiming this optimization strategy for encoding and decoding is proposed. Meanwhile, we design constructive-destructive search driven artificial bee colony algorithm to solve variable-speed green flow shop minimize makespan total energy consumption. A neighbor method designed update population in employed phase. process redesigned with three operators named technique order preferences similarity ideal solutions, binary tournament selection, global strategies onlooker In scout phase, individual evaluation replacement are designed. Extensive experimental evaluations testify CDSABC outperforms other algorithms regarding best, worst, average, standard deviation IGD index 80% test cases.
Language: Английский
Citations
0Knowledge-Based Systems, Journal Year: 2025, Volume and Issue: unknown, P. 113424 - 113424
Published: March 1, 2025
Language: Английский
Citations
0Robotics and Computer-Integrated Manufacturing, Journal Year: 2025, Volume and Issue: 95, P. 103029 - 103029
Published: April 21, 2025
Language: Английский
Citations
0European Journal of Operational Research, Journal Year: 2025, Volume and Issue: unknown
Published: May 1, 2025
Language: Английский
Citations
0IET Collaborative Intelligent Manufacturing, Journal Year: 2024, Volume and Issue: 6(1)
Published: March 1, 2024
Abstract A distributed heterogeneous permutation flowshop scheduling problem with sequence‐dependent setup times (DHPFSP‐SDST) is addressed, which well reflects real‐world scenarios in factories. The objective to minimise the maximum completion time (makespan) by assigning jobs factories, and sequencing them within each factory. First, a mathematical model describe DHPFSP‐SDST established. Second, four meta‐heuristics, including genetic algorithms, differential evolution, artificial bee colony, iterated greedy (IG) algorithms are improved optimally solve concerned compared other existing optimisers literature. Nawaz‐Enscore‐Ham (NEH) heuristic employed for generating an initial solution. Then, five local search operators designed based on characteristics enhance algorithms' performance. To choose appropriately during iterations, Q‐learning‐based strategy adopted. Finally, extensive numerical experiments conducted 72 instances using 5 optimisers. obtained optimisation results comparisons prove that IG algorithm along Q‐learning selection shows better performance respect its peers. proposed exhibits higher efficiency problems.
Language: Английский
Citations
3Engineering Optimization, Journal Year: 2024, Volume and Issue: unknown, P. 1 - 28
Published: April 26, 2024
Nowadays, manufacturing enterprises must have fast response and flexible production capabilities to meet personalized diversified market demands. Mixed-model distributed become the preferred methods for enterprises. This article studies a heterogeneous hybrid flow shop scheduling problem with mixed-model assembly line (DHHFSP-MMAL), which consists of stages. The DHHFSP-MMAL is modelled by mixed integer linear programming (MILP) model. Three constructive heuristics parallel deep adaptive large neighbourhood search (PDALNS) are presented. A heuristic group strategy employed obtain an initial solution. Several destroy-and-repair operators proposed where problem-specific greedy local applied. PDALNS assigns weights guide selection operators. computing technique introduced increase efficiency training. experiments demonstrate that algorithm efficient effective solving problem.
Language: Английский
Citations
3Swarm and Evolutionary Computation, Journal Year: 2024, Volume and Issue: 91, P. 101771 - 101771
Published: Nov. 14, 2024
Language: Английский
Citations
3IEEE Transactions on Evolutionary Computation, Journal Year: 2023, Volume and Issue: 28(5), P. 1427 - 1441
Published: Sept. 7, 2023
Handling energy resource management (ERM) in today's systems is complex and challenging due to uncertainties arising from the high penetration of distributed resources. Such introduces various uncertain factors, such as renewable energy, storage, electric vehicles, making it difficult for traditional mathematical methods find effective solutions. However, Evolutionary Algorithms (EAs) have shown good performance solving this problem. Therefore, paper, a self-adaptive collaborative differential evolution algorithm (SADEA) proposed solve ERM problem under uncertainty. In SADEA, three-stage adaptive collaboration strategy, includes boundary randomization stage, knowledge-assisted range restructuration used generate The solutions generated above stages will jointly participate perturbation DE strategies explore promising addition, different are selected according count values random factors. At end algorithm, control, elite selection retention ensure legitimacy robustness SADEA compared several state-of-the-art algorithms on real-world distribution network located Salamanca, Spain. results show that superior its competitors terms objective function, ranking index, convergence. summary, handle
Language: Английский
Citations
8Swarm and Evolutionary Computation, Journal Year: 2024, Volume and Issue: 86, P. 101545 - 101545
Published: March 22, 2024
Language: Английский
Citations
2