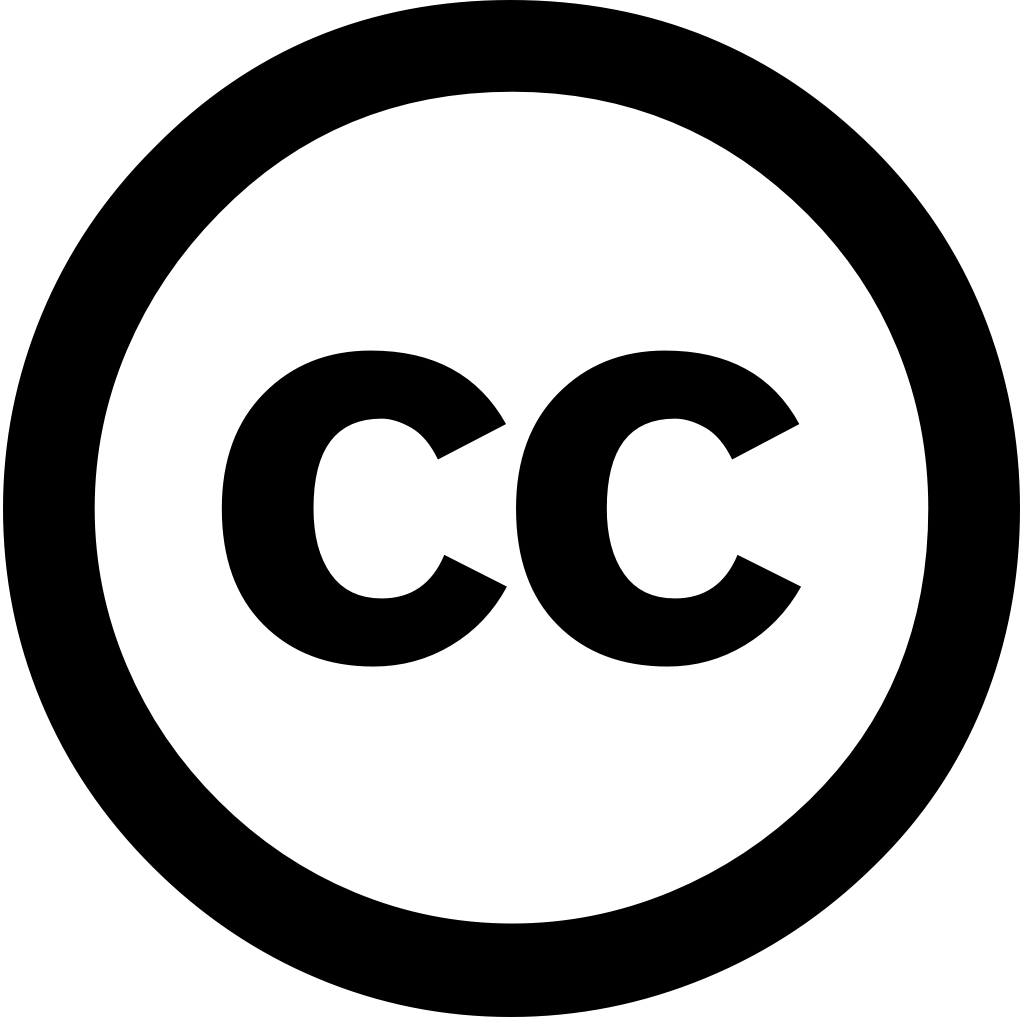
Advanced Intelligent Systems, Journal Year: 2025, Volume and Issue: unknown
Published: April 6, 2025
At the intersection of cutting‐edge technologies and privacy concerns, federated learning (FL) with its distributed architecture, stands at forefront in a bid to facilitate collaborative model training across multiple clients while preserving data privacy. However, applicability FL systems is hindered by fairness concerns arising from numerous sources heterogeneity that can result biases undermine system's effectiveness, skewed predictions, reduced accuracy, inefficient convergence. This survey thus explores diverse bias, including but not limited to, data, client, biases, thoroughly discusses strengths limitations inherited within array state‐of‐the‐art techniques utilized literature mitigate such disparities process. A comprehensive overview several notions, theoretical underpinnings, technical aspects associated their adoption FL‐based multidisciplinary environments are delineated. Furthermore, salient evaluation metrics leveraged measure quantitatively examined. Finally, exciting open research directions have potential drive future advancements achieving fairer frameworks, turn, offering strong foundation for this pivotal area envisaged.
Language: Английский