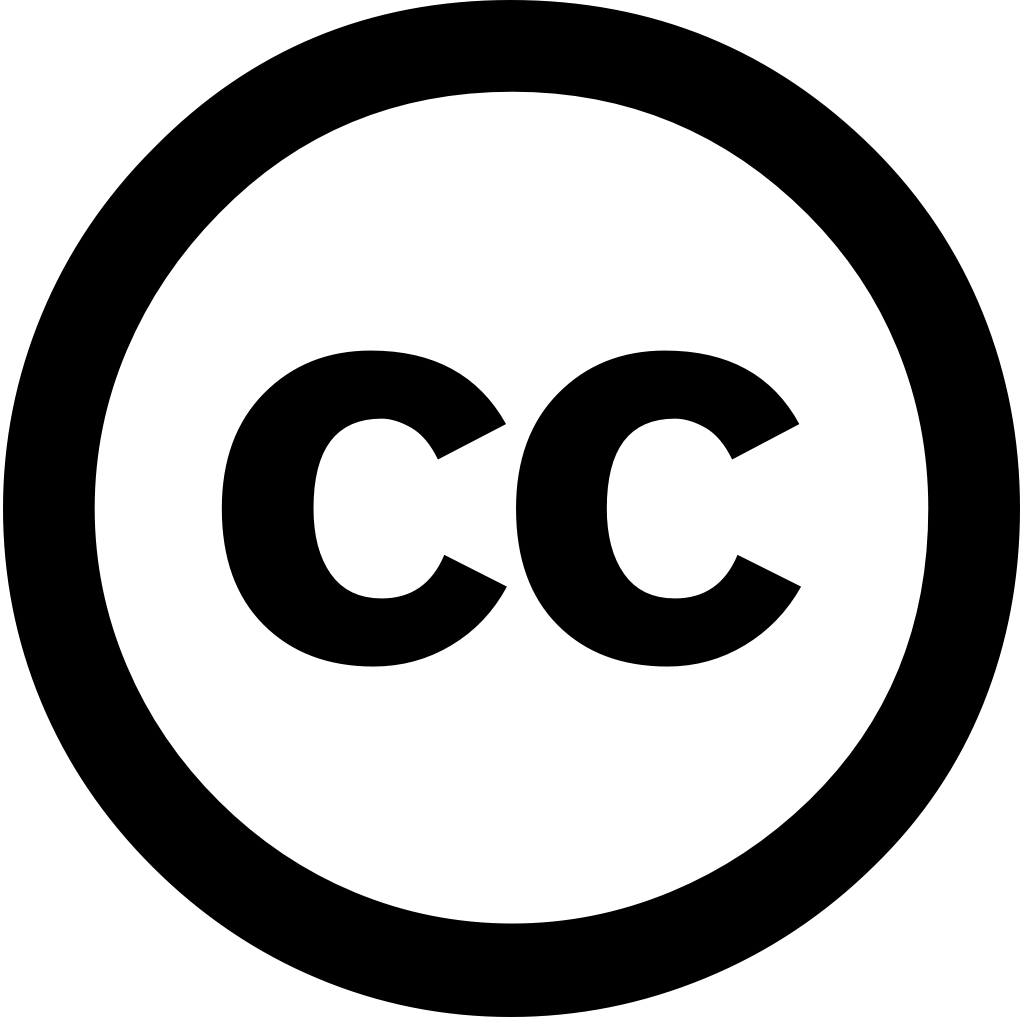
Complex & Intelligent Systems, Journal Year: 2025, Volume and Issue: 11(5)
Published: March 17, 2025
Language: Английский
Complex & Intelligent Systems, Journal Year: 2025, Volume and Issue: 11(5)
Published: March 17, 2025
Language: Английский
Computers & Industrial Engineering, Journal Year: 2024, Volume and Issue: 196, P. 110484 - 110484
Published: Aug. 18, 2024
Language: Английский
Citations
28Computers & Industrial Engineering, Journal Year: 2024, Volume and Issue: 189, P. 109917 - 109917
Published: Feb. 2, 2024
Language: Английский
Citations
26Applied Soft Computing, Journal Year: 2025, Volume and Issue: unknown, P. 112780 - 112780
Published: Jan. 1, 2025
Language: Английский
Citations
2Swarm and Evolutionary Computation, Journal Year: 2024, Volume and Issue: 87, P. 101550 - 101550
Published: April 1, 2024
Language: Английский
Citations
16Computers & Electrical Engineering, Journal Year: 2024, Volume and Issue: 120, P. 109780 - 109780
Published: Oct. 18, 2024
Language: Английский
Citations
15Engineering Applications of Artificial Intelligence, Journal Year: 2024, Volume and Issue: 135, P. 108775 - 108775
Published: June 12, 2024
Language: Английский
Citations
9Computers & Industrial Engineering, Journal Year: 2025, Volume and Issue: unknown, P. 110829 - 110829
Published: Jan. 1, 2025
Language: Английский
Citations
1Computers & Industrial Engineering, Journal Year: 2025, Volume and Issue: unknown, P. 110856 - 110856
Published: Jan. 1, 2025
Language: Английский
Citations
1Computers & Industrial Engineering, Journal Year: 2025, Volume and Issue: unknown, P. 110990 - 110990
Published: Feb. 1, 2025
Language: Английский
Citations
1IEEE Transactions on Automation Science and Engineering, Journal Year: 2023, Volume and Issue: 21(4), P. 6550 - 6562
Published: Nov. 1, 2023
Distributed
manufacturing
involving
heterogeneous
factories
presents
significant
challenges
to
enterprises.
Furthermore,
the
need
prioritize
various
jobs
based
on
order
urgency
and
customer
importance
further
complicates
scheduling
process.
Consequently,
this
study
addresses
practical
issue
by
tackling
distributed
hybrid
flow
shop
problem
with
multiple
priorities
of
(DHHFSP-MPJ).
The
primary
objective
is
simultaneously
minimize
total
weighted
tardiness
energy
consumption.
To
solve
DHHFSP-MPJ,
a
double
deep
Q-network-based
co-evolution
(D2QCE)
developed
four
features:
i)
global
local
searches
are
allocated
into
two
populations
balance
computational
resources;
ii)
A
heuristic
strategy
proposed
obtain
an
initialized
population
great
convergence
diversity;
iii)
Four
knowledge-based
neighborhood
structures
accelerate
converging.
Next,
Q-Network
applied
learn
operator
selection;
iv)
An
energy-efficient
presented
save
energy.
verify
effectiveness
D2QCE,
five
state-of-the-art
algorithms
compared
20
instances
real-world
case.
results
numerical
experiments
indicate
that:
D2QN
can
fast
only
consuming
few
computation
resources
select
best
operator.
Combining
vastly
improve
performance
evolutionary
for
solving
scheduling.
D2QCE
has
better
than
state-of-the-arts
DHHFSP-MPJ
Language: Английский
Citations
19