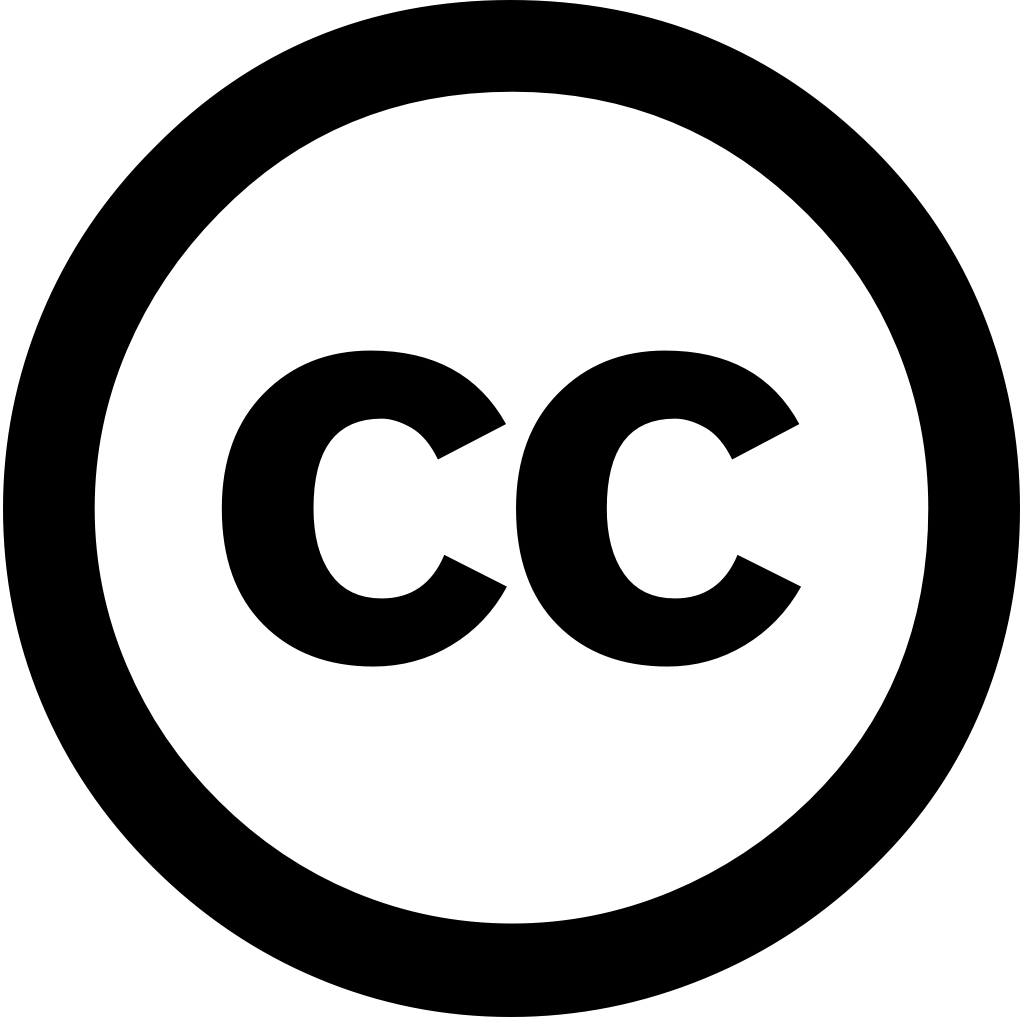
Frontiers in Medicine, Journal Year: 2025, Volume and Issue: 12
Published: March 27, 2025
Background Deep learning has shown considerable promise in the differential diagnosis of lung lesions. However, majority previous studies have focused primarily on X-ray, computed tomography (CT), and magnetic resonance imaging (MRI), with relatively few investigations exploring predictive value ultrasound imaging. Objective This study aims to develop a deep model based differentiate between benign malignant peripheral tumors. Methods A retrospective analysis was conducted cohort 371 patients who underwent ultrasound-guided percutaneous tumor procedures across two centers. The dataset divided into training set ( n = 296) test 75) an 8:2 ratio for further evaluation. Five distinct models were developed using ResNet152, ResNet101, ResNet50, ResNet34, ResNet18 algorithms. Receiver Operating Characteristic (ROC) curves generated, Area Under Curve (AUC) calculated assess diagnostic performance each model. DeLong’s employed compare differences groups. Results Among five models, one algorithm demonstrated highest performance. It exhibited statistically significant advantages accuracy p < 0.05) compared ResNet34 Specifically, showed superior discriminatory power. Quantitative evaluation through Net Reclassification Improvement (NRI) revealed that NRI values model, when 0.180, 0.240, 0.186, 0.221, respectively. All corresponding -values less than 0.05 comparison), confirming significantly outperformed other four reclassification ability. Moreover, its outcomes led marked improvements risk stratification classification accuracy. Conclusion ResNet18-based distinguishing tumors, providing effective non-invasive tool early detection cancer.
Language: Английский