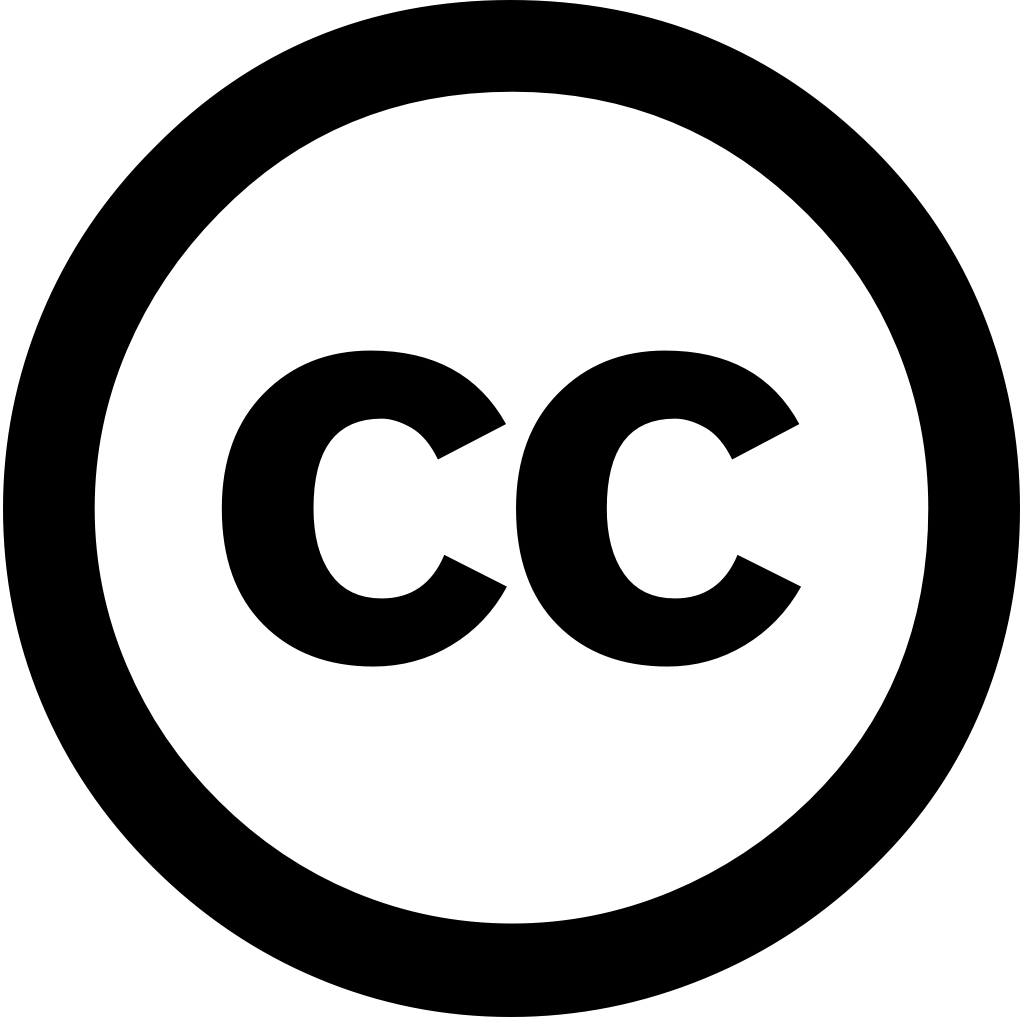
Published: May 9, 2024
Language: Английский
Published: May 9, 2024
Language: Английский
BMC Gastroenterology, Journal Year: 2025, Volume and Issue: 25(1)
Published: April 29, 2025
The red blood cell distribution width-to-albumin ratio (RAR) is a novel biomarker that concurrently reflects nutritional status and inflammation. Unlike traditional cancer risk markers focus on either inflammation or nutrition independently, RAR provides more integrated assessment of these interrelated processes, making it promising tool for prediction. This study aims to investigate the relationship between digestive tract tumors (DTT), with particular emphasis colorectal (CC) gastric (GC). explored DTT using data from 32,953 participants in 2005-2018 National Health Nutrition Examination Survey (NHANES). Although weighted multivariate logistic regression models were used adjust potential confounders, residual confounding selection bias may still affect accuracy generalizability findings, potentially influencing causal inferences. Additionally, subgroup analyses, interaction tests, restricted cubic splines performed further examine associations. A two-sample Mendelian randomization analysis was also conducted DTT. Among participants, 234 diagnosed DTT, including 215 cases CC 19 GC. Higher levels significantly associated an increased (OR = 1.48, 95% CI 1.04-2.11, P < 0.027), but not GC 1.33, 0.45-3.94, 0.60). non-linear association observed. indicated albumin negatively 0.84, 0.73-0.97), while erythrocyte width (RDW) showed no significant association. reveals risk, indicating serve as valuable stratification. For individuals abnormal values, integration supplementary screening tools-such fecal occult testing, colonoscopy, additional biomarkers-could enhance early detection rates CC. strategy would allow healthcare providers effectively identify high-risk tailor personalized prevention strategies.
Language: Английский
Citations
0Array, Journal Year: 2024, Volume and Issue: 22, P. 100345 - 100345
Published: April 26, 2024
Nowadays, artificial intelligence (AI) has been utilized in several domains of the healthcare sector. Despite its effectiveness settings, massive adoption remains limited due to transparency issue, which is considered a significant obstacle. To achieve trust end users, it necessary explain AI models' output. Therefore, explainable (XAI) become apparent as potential solution by providing transparent explanations In this review paper, primary aim articles that are mainly related machine learning (ML) or deep (DL) based human disease diagnoses, and model's decision-making process explained XAI techniques. do that, two journal databases (Scopus IEEE Xplore Digital Library) were thoroughly searched using few predetermined relevant keywords. The PRISMA guidelines have followed determine papers for final analysis, where studies did not meet requirements eliminated. Finally, 90 Q1 selected in-depth covering Then, summarization findings presented, appropriate responses proposed research questions outlined. addition, challenges case diagnosis future directions sector presented.
Language: Английский
Citations
3Medicine & Science in Sports & Exercise, Journal Year: 2024, Volume and Issue: 56(5), P. 868 - 875
Published: Feb. 2, 2024
We develop blood test-based aging clocks and examine how these reflect high-volume sports activity.
Language: Английский
Citations
1medRxiv (Cold Spring Harbor Laboratory), Journal Year: 2024, Volume and Issue: unknown
Published: Jan. 12, 2024
Abstract Background The red cell distribution width to albumin ratio (RAR) has emerged as a reliable prognostic marker for mortality in various diseases. However, whether RAR is associated with remains unknown the general population. Objective Explore all-cause and cause-specific mortality, elucidate dose-response relationship between them. Methods This study included 50622 participants aged 18+ years from US National Health Nutrition Examination Survey (NHANES), 418950 37+ UK Biobank. potential association risk of was evaluated by Cox proportional hazard models. Restricted cubic spline regressions were applied estimate possible nonlinear relationships. Results NHANES documented 7590 deaths over median follow-up 9.4 years, Biobank 36793 14.5 years. In multivariable analysis, elevated significantly higher (NHANES: [HR]: 1.86, 95% confidence interval [CI]: 1.81-1.93; Biobank: HR: 2.01, CI: 1.96-2.06), well due malignant neoplasms, heart disease, cerebrovascular diseases, respiratory diabetes mellitus, others both two cohorts (all P-value < 0.05). Conclusions Higher baseline strongly independently increased promising indicator that simply, reliably, inexpensively accessible identifying high-risk clinical practice.
Language: Английский
Citations
0Published: May 9, 2024
Language: Английский
Citations
0