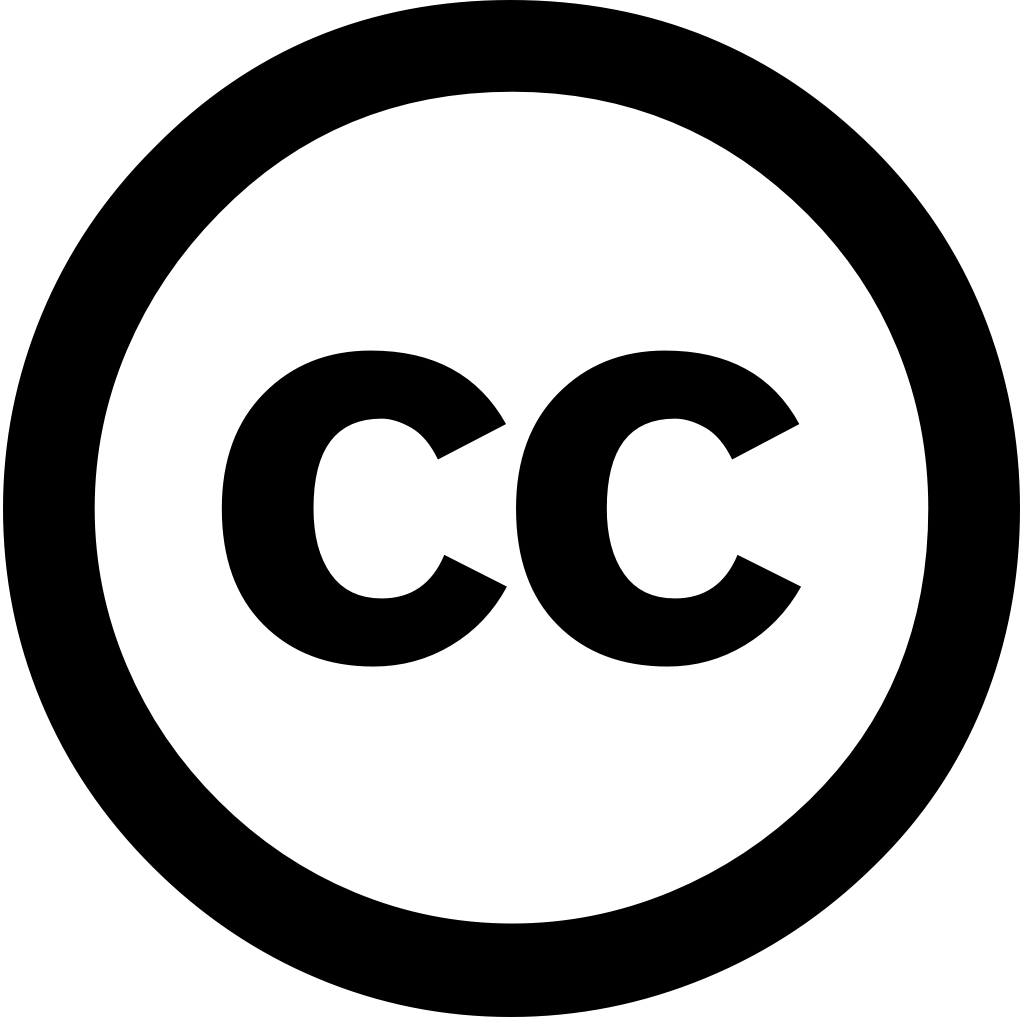
bioRxiv (Cold Spring Harbor Laboratory), Journal Year: 2025, Volume and Issue: unknown
Published: Jan. 29, 2025
A bstract The unpredictability of seizures is one the most compromising features reported by people with epilepsy. Non-stigmatizing and easy-to-use wearable devices may provide information to predict based on physiological data. We propose a patient-agnostic seizure prediction method that identifies group-level patterns across data from multiple patients. employ supervised long-short-term networks (LSTMs) add unsupervised deep canonically correlated autoencoders (DCCAE) 24-hour using time-of-day information. fuse these three techniques growing neural network, allowing incremental learning. Our all improves accuracy over baseline LSTM 7.3%, 74.4% 81.7%, averaged patients, outperforms in 84% Compared all-at-once fusion, network 9.5%. analyze impact preictal duration, quality, clinical variables performance.
Language: Английский