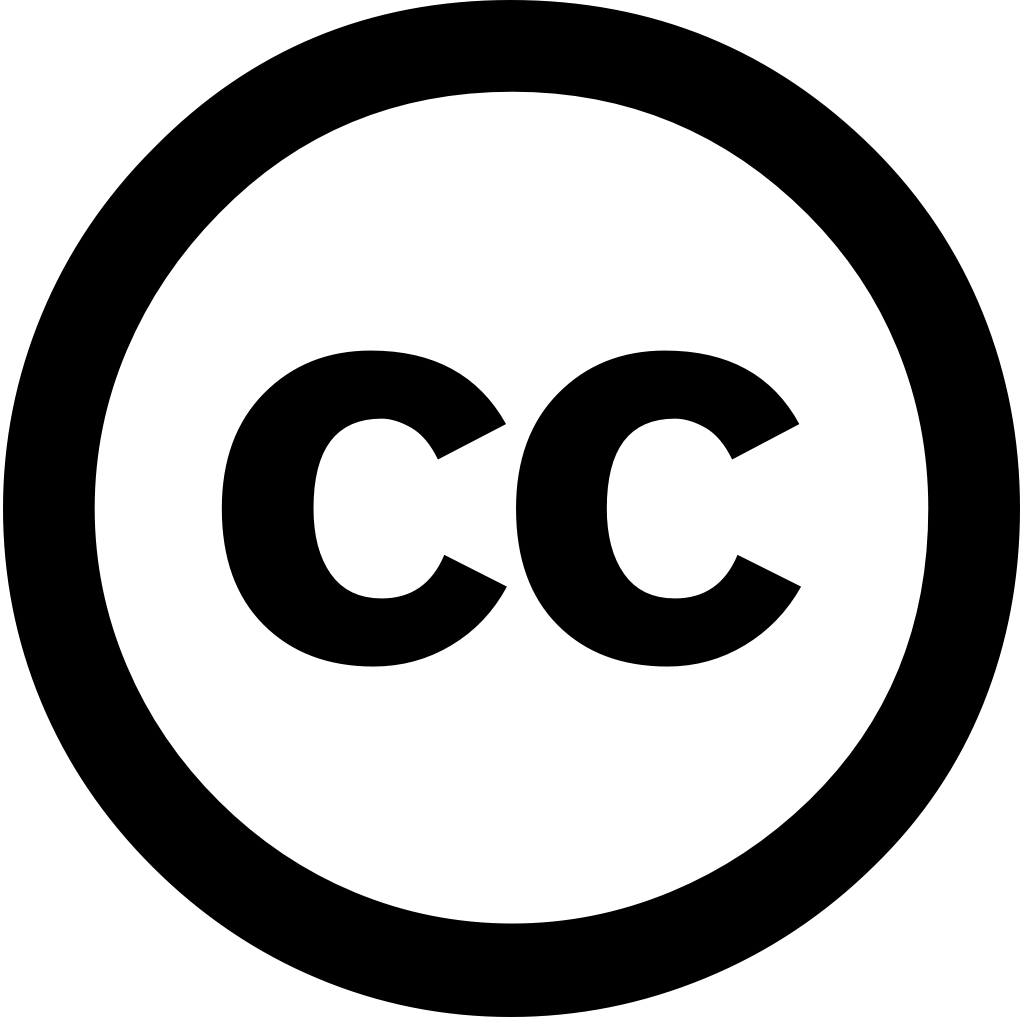
bioRxiv (Cold Spring Harbor Laboratory), Journal Year: 2024, Volume and Issue: unknown
Published: March 17, 2024
Abstract The proliferation of high-dimensional data in ecology and evolutionary biology raise the promise statistical machine learning models that are highly predictive interpretable. However, commonly burdened with an inherent trade-off: in-sample prediction outcomes will improve as additional predictors included model, but this may come at cost poor accuracy limited generalizability for future or unsampled observations (out-of-sample prediction). To confront problem overfitting, sparse can focus on key by correctly placing low weight unimportant variables. We competed nine methods to quantify their performance variable selection using simulated different sample sizes, numbers predictors, strengths effects. Overfitting was typical many simulation scenarios. Despite this, out-of-sample converged true target simulations more observations, larger causal effects, fewer predictors. Accurate support process-based understanding be unattainable realistic sampling schemes evolution. use our analyses characterize attributes which is possible, illustrate how some achieve while mitigating extent overfitting.
Language: Английский