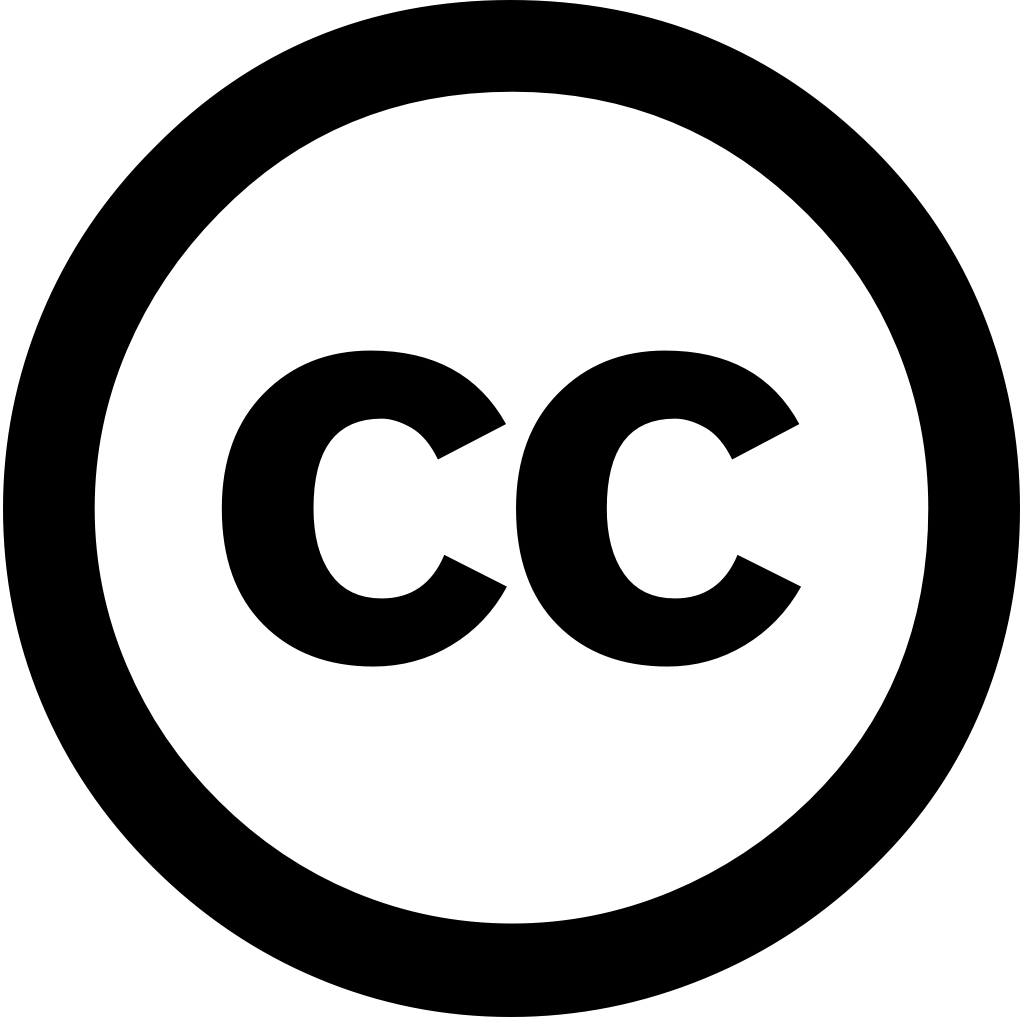
bioRxiv (Cold Spring Harbor Laboratory), Journal Year: 2024, Volume and Issue: unknown
Published: Oct. 18, 2024
Abstract 1. Species distribution models (SDMs) are crucial tools for understanding and predicting biodiversity patterns, yet they often struggle with limited data, biased sampling, complex species-environment relationships. Here I present NicheFlow, a novel foundation model SDMs that leverages generative AI to address these challenges advance our ability predict species distributions across taxa environments. 2. NicheFlow employs two-stage approach, combining embeddings two chained models, one generate in environmental space, second geographic space. This architecture allows the sharing of information captures complex, non-linear relationships trained on comprehensive dataset reptile evaluated its performance using both standard SDM metrics zero-shot prediction tasks. 3. demonstrates good predictive performance, particularly rare data-deficient species. The successfully generated plausible not seen during training, showcasing potential prediction. learned captured meaningful ecological information, revealing patterns niche structure taxa, latitude range sizes. 4. As proof-of-principle model, represents significant modeling, offering powerful tool addressing pressing questions ecology, evolution, conservation biology. Its joint hypothetical niches opens new avenues exploring evolutionary questions, including ancestral reconstruction community assembly processes. approach has transform improve capacity manage face global change.
Language: Английский