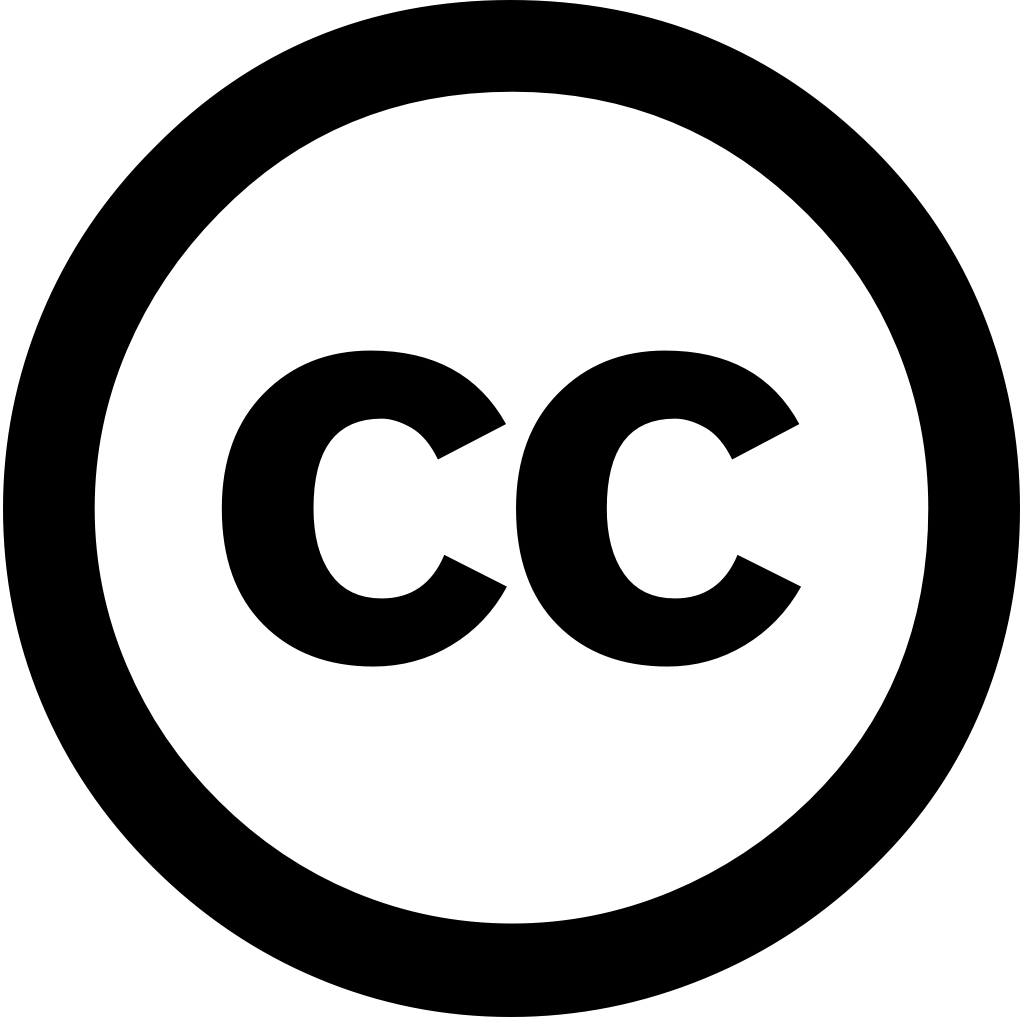
Frontiers in Digital Health, Journal Year: 2025, Volume and Issue: 7
Published: March 28, 2025
Hypernasality, a hallmark of velopharyngeal insufficiency (VPI), is speech disorder with significant psychosocial and functional implications. Conventional diagnostic methods rely heavily on specialized expertise equipment, posing challenges in resource-limited settings. This study explores the application OpenAI's Whisper model for automated hypernasality detection, offering scalable efficient alternative to traditional approaches. The was adapted binary classification by replacing its sequence-to-sequence decoder custom head. A dataset 184 audio recordings, including 96 hypernasal (cases) 88 non-hypernasal samples (controls), used training evaluation. model's performance compared machine learning approaches, support vector machines (SVM) random forest (RF) classifiers. Whisper-based effectively detected speech, achieving test accuracy 97% an F1-score 0.97. It significantly outperformed SVM RF classifiers, which achieved accuracies 88.1% 85.7%, respectively. demonstrated robust across diverse recording conditions required minimal data, showcasing scalability efficiency detection. demonstrates effectiveness By providing reliable pretest probability, can serve as triaging mechanism prioritize patients further evaluation, reducing delays optimizing resource allocation.
Language: Английский