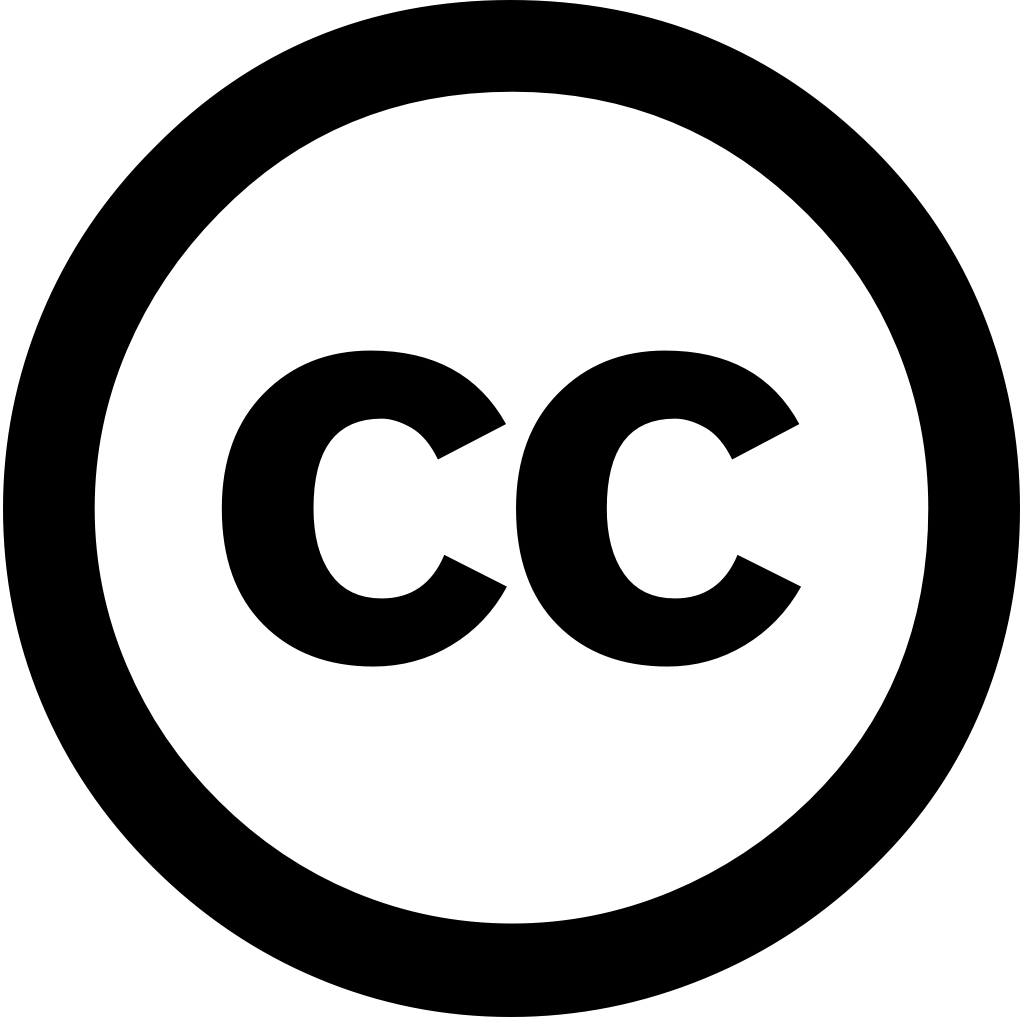
Computer-Aided Civil and Infrastructure Engineering, Journal Year: 2025, Volume and Issue: unknown
Published: April 23, 2025
Abstract High‐resolution flood probability maps are instrumental for assessing risk but often limited by the availability of historical data. Additionally, producing simulated data needed creating probabilistic using physics‐based models involves significant computation and time effort, which inhibit its feasibility. To address this gap, study introduces Precipitation‐Flood Depth Generative Pipeline, a novel methodology that leverages generative machine learning to generate large‐scale synthetic inundation produce maps. With focus on Harris County, Texas, Pipeline begins with training cell‐wise depth estimator number precipitation‐flood events model model. This estimator, emphasizes precipitation‐based features, outperforms universal models. Subsequently, conditional adversarial network (CTGAN) is used conditionally precipitation point cloud, filtered strategic thresholds align realistic patterns. Hence, feature pool constructed each cell, enabling sampling generation events. After generating 10,000 events, created various depths. Validation similarity correlation metrics confirms accuracy distributions. The provides scalable solution high‐resolution maps, can enhance mitigation planning.
Language: Английский