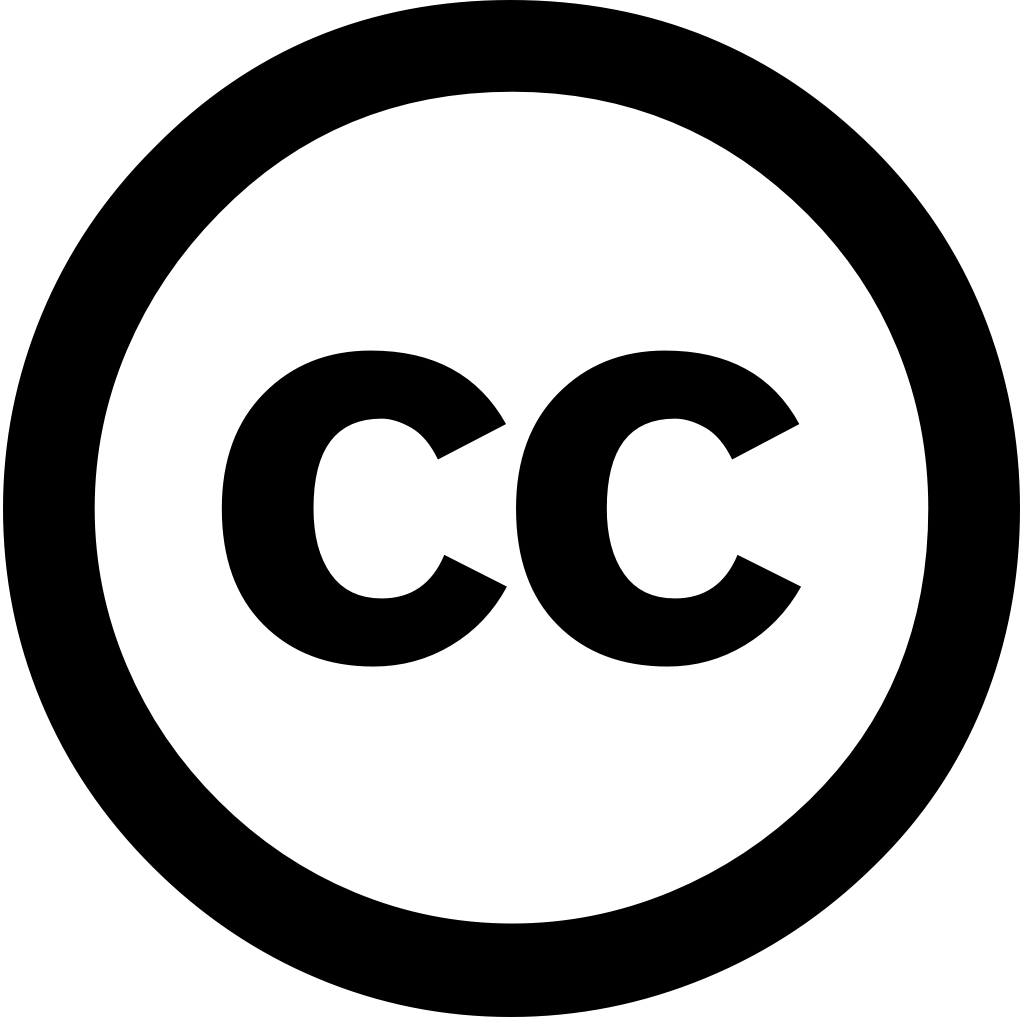
Results in Engineering, Journal Year: 2025, Volume and Issue: unknown, P. 105156 - 105156
Published: May 1, 2025
Language: Английский
Results in Engineering, Journal Year: 2025, Volume and Issue: unknown, P. 105156 - 105156
Published: May 1, 2025
Language: Английский
ACM Computing Surveys, Journal Year: 2024, Volume and Issue: 56(10), P. 1 - 42
Published: May 11, 2024
Over the past decade, dominance of deep learning has prevailed across various domains artificial intelligence, including natural language processing, computer vision, and biomedical signal processing. While there have been remarkable improvements in model accuracy, deploying these models on lightweight devices, such as mobile phones microcontrollers, is constrained by limited resources. In this survey, we provide comprehensive design guidance tailored for detailing meticulous models, compression methods, hardware acceleration strategies. The principal goal work to explore methods concepts getting around constraints without compromising model’s accuracy. Additionally, two notable paths future: deployment techniques TinyML Large Language Models. Although undoubtedly potential, they also present significant challenges, encouraging research into unexplored areas.
Language: Английский
Citations
22Nature Communications, Journal Year: 2024, Volume and Issue: 15(1)
Published: Sept. 16, 2024
Spiking neural networks and neuromorphic hardware platforms that simulate neuronal dynamics are getting wide attention being applied to many relevant problems using Machine Learning. Despite a well-established mathematical foundation for dynamics, there exists numerous software solutions stacks whose variability makes it difficult reproduce findings. Here, we establish common reference frame computations in digital systems, titled Neuromorphic Intermediate Representation (NIR). NIR defines set of computational composable model primitives as hybrid systems combining continuous-time discrete events. By abstracting away assumptions around discretization constraints, faithfully captures the model, while bridging differences between evaluated implementation underlying formalism. supports an unprecedented number which demonstrate by reproducing three spiking network models different complexity across 7 simulators 4 platforms. decouples development software, enabling interoperability improving accessibility multiple technologies. We believe is key next step brain-inspired hardware-software co-evolution, research towards energy efficient principles nervous systems. available at neuroir.org.
Language: Английский
Citations
14Electronics, Journal Year: 2024, Volume and Issue: 13(9), P. 1624 - 1624
Published: April 24, 2024
Binarization is an extreme quantization technique that attracting research in the Internet of Things (IoT) field, as it radically reduces memory footprint deep neural networks without a correspondingly significant accuracy drop. To support effective deployment Binarized Neural Networks (BNNs), we propose CBin-NN, library layer operators allows building simple yet flexible convolutional (CNNs) with binary weights and activations. CBin-NN platform-independent thus portable to virtually any software-programmable device. Experimental analysis on CIFAR-10 dataset shows our library, compared set state-of-the-art inference engines, speeds up by 3.6 times required store model activations 7.5 28 times, respectively, at cost slightly lower (2.5%). An ablation study stresses importance Quantized Input Kernel Convolution improve reduce latency slight increase size.
Language: Английский
Citations
13Sensors, Journal Year: 2025, Volume and Issue: 25(2), P. 531 - 531
Published: Jan. 17, 2025
The integration of deep learning (DL) into image processing has driven transformative advancements, enabling capabilities far beyond the reach traditional methodologies. This survey offers an in-depth exploration DL approaches that have redefined processing, tracing their evolution from early innovations to latest state-of-the-art developments. It also analyzes progression architectural designs and paradigms significantly enhanced ability process interpret complex visual data. Key such as techniques improving model efficiency, generalization, robustness, are examined, showcasing DL's address increasingly sophisticated image-processing tasks across diverse domains. Metrics used for rigorous evaluation discussed, underscoring importance performance assessment in varied application contexts. impact is highlighted through its tackle challenges generate actionable insights. Finally, this identifies potential future directions, including emerging technologies like quantum computing neuromorphic architectures efficiency federated privacy-preserving training. Additionally, it highlights combining with edge explainable artificial intelligence (AI) scalability interpretability challenges. These advancements positioned further extend applications DL, driving innovation processing.
Language: Английский
Citations
1Future Internet, Journal Year: 2025, Volume and Issue: 17(2), P. 89 - 89
Published: Feb. 14, 2025
The rapid advancement of edge computing and Tiny Machine Learning (TinyML) has created new opportunities for deploying intelligence in resource-constrained environments. With the growing demand intelligent Internet Things (IoT) devices that can efficiently process complex data real-time, there is an urgent need innovative optimisation techniques overcome limitations IoT enable accurate efficient computations. This study investigates a novel approach to optimising Convolutional Neural Network (CNN) models Hand Gesture Recognition (HGR) based on Electrical Impedance Tomography (EIT), which requires signal processing, energy efficiency, real-time by simultaneously reducing input complexity using advanced model compression techniques. By systematically halving 1D CNN from 40 20 Boundary Voltages (BVs) applying method, we achieved remarkable size reductions 91.75% 97.49% BVs EIT inputs, respectively. Additionally, Floating-Point operations (FLOPs) are significantly reduced, more than 99% both cases. These have been with minimal loss accuracy, maintaining performance 97.22% 94.44% most significant result compressed model. In fact, at only 8.73 kB our demonstrates potential design strategies creating ultra-lightweight, high-performance CNN-based solutions near-full capabilities specifically case HGR inputs.
Language: Английский
Citations
1IEEE Access, Journal Year: 2024, Volume and Issue: 12, P. 133245 - 133314
Published: Jan. 1, 2024
The advent of the sixth generation mobile communications (6G) ushers in an era heightened demand for advanced network intelligence to tackle challenges expanding landscape and increasing service demands. Deep Learning (DL), as a crucial technique instilling into 6G, has demonstrated powerful promising development. This paper provides comprehensive overview pivotal role DL exploring myriad opportunities that arise. Firstly, we present detailed vision emphasizing areas such adaptive resource allocation, intelligent management, robust signal processing, ubiquitous edge intelligence, endogenous security. Secondly, this reviews how models leverage their unique learning capabilities solve complex demands 6G. discussed include Convolutional Neural Networks (CNN), Generative Adversarial (GAN), Graph (GNN), Reinforcement (DRL), Transformer, Federated (FL), Meta Learning. Additionally, examine specific each model faces within 6G context. Moreover, delve rapidly evolving field Artificial Intelligence Generated Content (AIGC), examining its development impact framework. Finally, culminates discussion ten critical open problems integrating with setting stage future research field.
Language: Английский
Citations
7Plant Phenomics, Journal Year: 2024, Volume and Issue: 6
Published: Jan. 1, 2024
Wheat stripe rust poses a marked threat to global wheat production. Accurate and effective disease severity assessments are crucial for resistance breeding timely management of field diseases. In this study, we propose practical solution using mobile-based deep learning model-assisted labeling. StripeRust-Pocket, user-friendly mobile application developed based on models, accurately quantifies in leaf images, even under complex backgrounds. Additionally, StripeRust-Pocket facilitates image acquisition, result storage, organization, sharing. The underlying model employed by called StripeRustNet, is balanced lightweight 2-stage model. first stage utilizes MobileNetV2-DeepLabV3+ segmentation, followed ResNet50-DeepLabV3+ the second lesion segmentation. Disease estimated calculating ratio pixel area area. StripeRustNet achieves 98.65% mean intersection over union (MIoU) segmentation 86.08% MIoU Validation an additional 100 images demonstrated correlation 0.964 with 3 expert visual scores. To address challenges manual labeling, introduce labeling pipeline that combines correction, spatial complementarity. We apply our self-collected dataset, reducing annotation time from 20 min per image. Our method provides efficient assessments, empowering breeders pathologists implement management. It also demonstrates how “last mile” challenge applying computer vision technology plant phenomics.
Language: Английский
Citations
5ACM Transactions on Intelligent Systems and Technology, Journal Year: 2024, Volume and Issue: 15(6), P. 1 - 16
Published: July 29, 2024
Vision-and-language pre-training models have achieved impressive performance for image captioning. But most of them are trained with millions paired image-text data and require huge memory computing overhead. To alleviate this, we try to stand on the shoulders large-scale pre-trained language (PLM) vision (PVM) efficiently connect There two major challenges: one is that modalities different semantic granularity (e.g., a noun may cover many pixels), other gap still exists between models. this end, design lightweight efficient connector glue PVM PLM, which holds criterion selection-then-transformation . Specifically, in selection phase, treat each as set patches instead pixels. We select salient cluster into visual regions align text. Then, effectively reduce gap, propose map selected text space through spatial channel transformations. With training captioning datasets, learns bridge via backpropagation, preparing PLM generate descriptions. Experimental results MSCOCO Flickr30k datasets demonstrate our method yields comparable existing works. By solely small connector, achieve CIDEr 132.2% Karpathy test split. Moreover, findings reveal fine-tuning can further enhance potential, resulting score 140.6%. Code available at https://github.com/YuanEZhou/PrefixCap
Language: Английский
Citations
5Sensors, Journal Year: 2025, Volume and Issue: 25(3), P. 970 - 970
Published: Feb. 6, 2025
Ensuring the safety and quality of poultry products requires efficient detection removal foreign materials during processing. Hyperspectral imaging (HSI) offers a non-invasive mechanism to capture detailed spatial spectral information, enabling discrimination different types contaminants from muscle non-muscle external tissues. When integrated with advanced deep learning (DL) models, HSI systems can achieve high accuracy in detecting materials. However, dimensionality data, computational complexity DL high-paced nature processing environments pose challenges for real-time implementation industrial settings, where speed decision-making is critical. In this study, we address these by optimizing inference HSI-based material through combination post-training quantization hardware acceleration techniques. We leveraged utilizing TensorRT module NVIDIA GPU enhance speed. Additionally, applied half-precision (called FP16) reduce precision model parameters, decreasing memory usage requirements without any loss accuracy. conducted simulations using two hypothetical hyperspectral line-scan cameras evaluate feasibility conditions. The simulation results demonstrated that our optimized models could times compatible line speeds lines between 140 250 birds per minute, indicating potential deployment. Specifically, proposed method, compression, achieved reductions time up five compared unoptimized, traditional GPU-based inference. addition, it resulted 50% decrease size while maintaining was also comparable original model. Our findings suggest integration an effective strategy overcoming bottlenecks associated on data.
Language: Английский
Citations
0Lecture notes in computer science, Journal Year: 2025, Volume and Issue: unknown, P. 131 - 144
Published: Jan. 1, 2025
Language: Английский
Citations
0