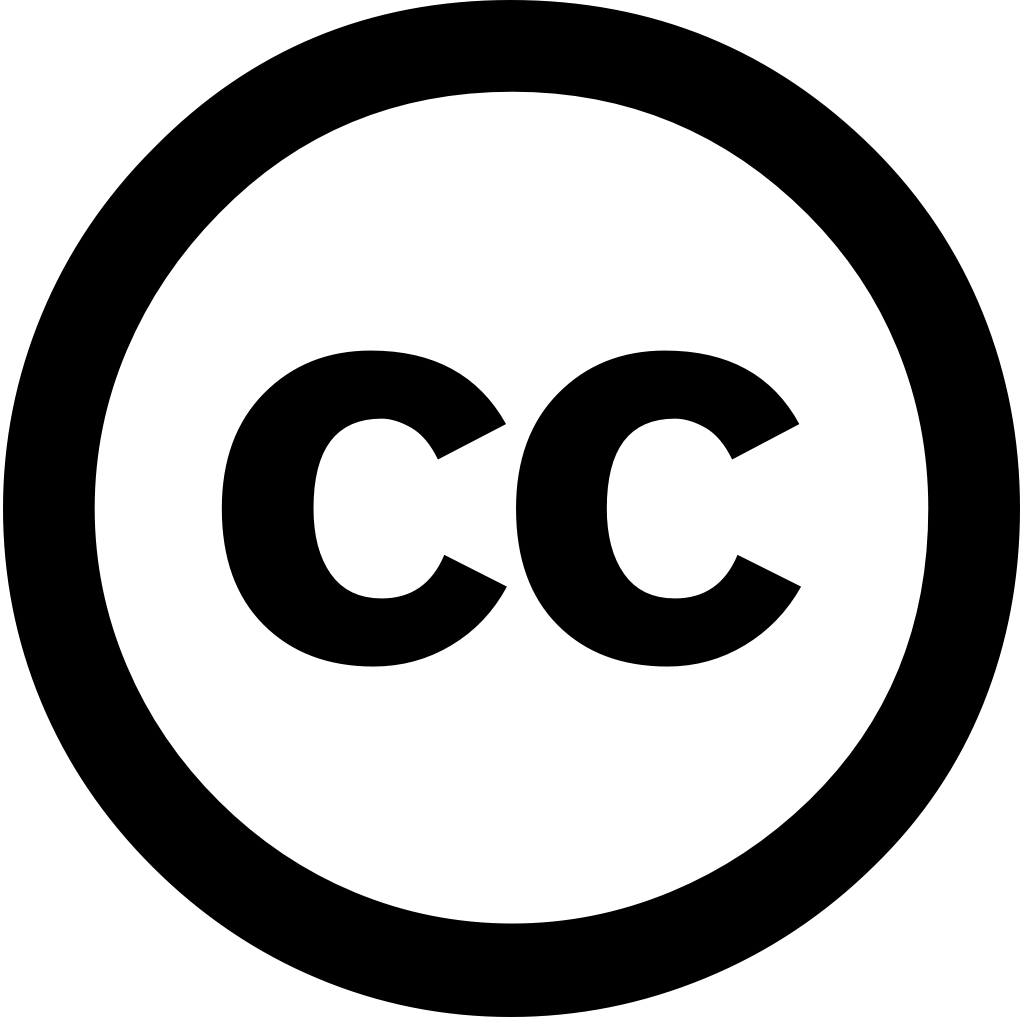
Results in Engineering, Journal Year: 2025, Volume and Issue: unknown, P. 105156 - 105156
Published: May 1, 2025
Language: Английский
Results in Engineering, Journal Year: 2025, Volume and Issue: unknown, P. 105156 - 105156
Published: May 1, 2025
Language: Английский
Neural Networks, Journal Year: 2025, Volume and Issue: unknown, P. 107384 - 107384
Published: March 1, 2025
Language: Английский
Citations
0Water Research, Journal Year: 2025, Volume and Issue: unknown, P. 123760 - 123760
Published: May 1, 2025
Language: Английский
Citations
0Knowledge-Based Systems, Journal Year: 2025, Volume and Issue: 310, P. 113002 - 113002
Published: Jan. 22, 2025
Language: Английский
Citations
0Computers & Security, Journal Year: 2025, Volume and Issue: unknown, P. 104323 - 104323
Published: Feb. 1, 2025
Language: Английский
Citations
0Artificial Life and Robotics, Journal Year: 2025, Volume and Issue: unknown
Published: Feb. 12, 2025
Language: Английский
Citations
0Cogent Food & Agriculture, Journal Year: 2025, Volume and Issue: 11(1)
Published: Feb. 12, 2025
Image classification poses a significant challenge in agriculture. However, the utilization of popular algorithms such as vision transformers and convolutional neural networks has often fallen short numerous agricultural tasks owing to scarcity extensively labelled data reliance on pretrained models trained generic datasets. To address this, our study details pretraining ViTs using 224,228 images, employing masked image modeling for preprocessing. The model was then fine-tuned three independent datasets performed better than state-of-the-art methods. For example, method achieved highest accuracy rates 76.18%, 98.49%, 88.56% IP102, DeepWeeds, Tsinghua Dogs datasets, respectively. This enhancement can be attributed robust strategy we have developed through extensive experimentation with MIM model. Our encompasses advanced models, leveraging histogram oriented gradient features reconstruction target, selecting an appropriate mask ratio. We hope that this research will prompt application self-supervised learning techniques, represented by model, wide range image-related future.
Language: Английский
Citations
0IntechOpen eBooks, Journal Year: 2025, Volume and Issue: unknown
Published: Feb. 24, 2025
Ensuring the reliability, safety, and efficiency of electric vehicles (EVs) necessitates precise diagnostics battery life, as degradation batteries directly influences both performance sustainability. The transformative role artificial intelligence (AI) in advancing EV is explored herein, with an emphasis placed on complexities predicting managing health. Initially, we provide overview challenges associated lifetime diagnostics, such issues accuracy, generalization, model training. following sections delve into advanced AI methodologies that enhance diagnostic capabilities. These methods include extensive time-series AI, which improves predictive accuracy; end-to-end simplifies system complexity; multi-model ensures generalization across varied operating conditions; adaptable strategies for dynamic environments. In addition, explore use federated learning decentralized, privacy-preserving discuss automated machine streamlining development AI-based models. By integrating these sophisticated techniques, present a comprehensive roadmap future AI-driven prognostics health management. This underscores critical importance scalability, sustainability fostering advancement. Our interdisciplinary framework offers valuable insights can accelerate electrification transportation advance evolution energy storage systems, tackling key at intersection technology AI.
Language: Английский
Citations
0Mathematics, Journal Year: 2025, Volume and Issue: 13(5), P. 887 - 887
Published: March 6, 2025
DeepFake detection models play a crucial role in ambient intelligence and smart environments, where systems rely on authentic information for accurate decisions. These integrating interconnected IoT devices AI-driven systems, face significant threats from DeepFakes, potentially leading to compromised trust, erroneous decisions, security breaches. To mitigate these risks, neural-network-based have been developed. However, their substantial computational requirements long training times hinder deployment resource-constrained edge devices. This paper investigates compression transfer learning techniques reduce the demands of deploying models, while preserving performance. Pruning, knowledge distillation, quantization, adapter modules are explored enable efficient real-time detection. An evaluation was conducted four benchmark datasets: “SynthBuster”, “140k Real Fake Faces”, “DeepFake Images”, “ForenSynths”. It compared compressed with uncompressed baselines using widely recognized metrics such as accuracy, precision, recall, F1-score, model size, time. The results showed that at 10% original size retained only 56% baseline but fine-tuning similar scenarios increased this nearly 98%. In some cases, accuracy even surpassed original’s performance by up 12%. findings highlight feasibility computing scenarios.
Language: Английский
Citations
0The Visual Computer, Journal Year: 2025, Volume and Issue: unknown
Published: March 19, 2025
Language: Английский
Citations
0EURASIP Journal on Advances in Signal Processing, Journal Year: 2025, Volume and Issue: 2025(1)
Published: March 20, 2025
Language: Английский
Citations
0