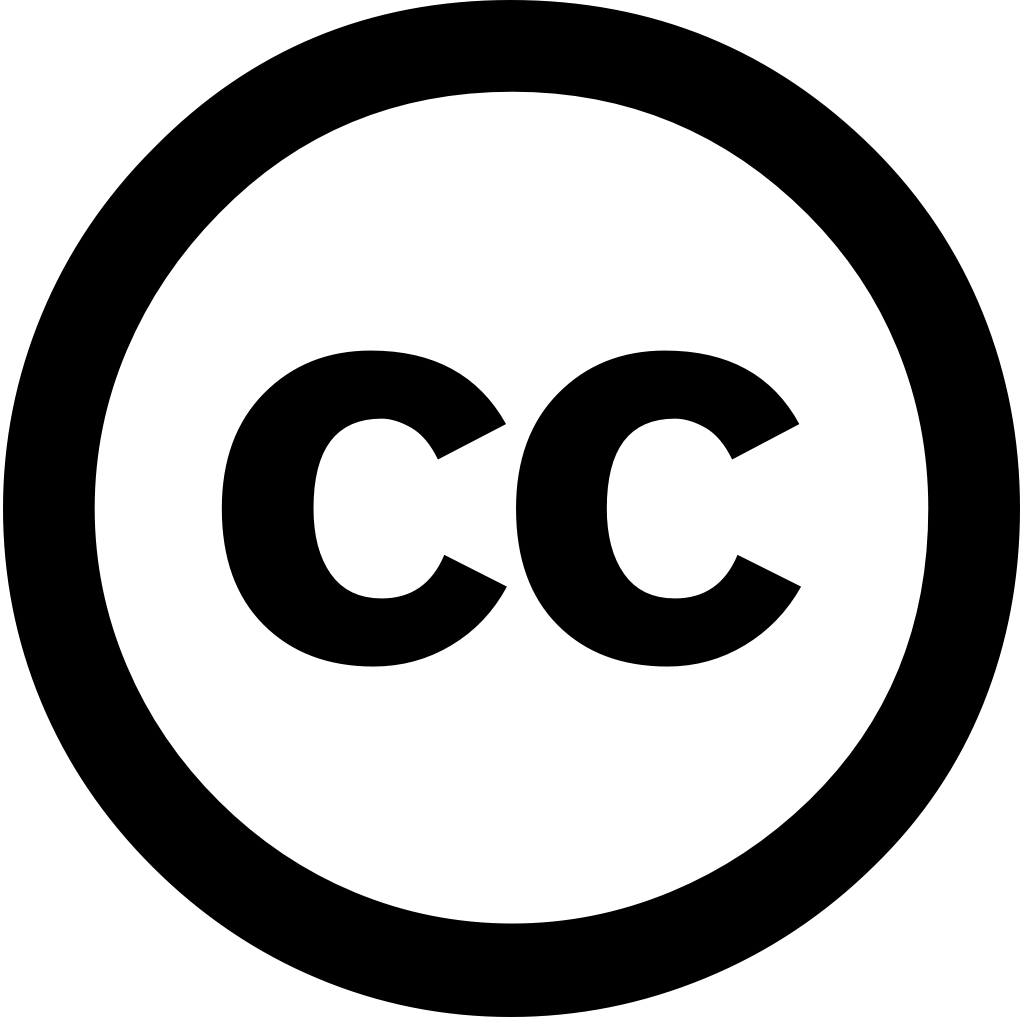
bioRxiv (Cold Spring Harbor Laboratory), Journal Year: 2023, Volume and Issue: unknown
Published: Oct. 10, 2023
Abstract Training recurrent neural networks (RNNs) has become a go-to approach for generating and evaluating mechanistic hypotheses cognition. The ease efficiency of training RNNs with backpropagation through time the availability robustly supported deep learning libraries made RNN modeling more approachable accessible to neuroscience. Yet, major technical hindrance remains. Cognitive processes such as working memory decision making involve population dynamics over long period within behavioral trial across trials. It is difficult train accomplish tasks where representations have temporal dependencies without gating mechanisms LSTMs or GRUs which currently lack experimental support prohibit direct comparison between biological circuits. We tackled this problem based on idea specialized skip-connections emergence task-relevant dynamics, subsequently reinstitute plausibility by reverting original architecture. show that enables successfully learn cognitive prove impractical if not impossible using conventional methods. Over numerous considered here, we achieve less steps shorter wall-clock times, particularly in require long-term via integration timescales maintaining past events hidden-states. Our methods expand range biologically plausible models can learn, thereby supporting development theory emergent computations involving dependencies.
Language: Английский