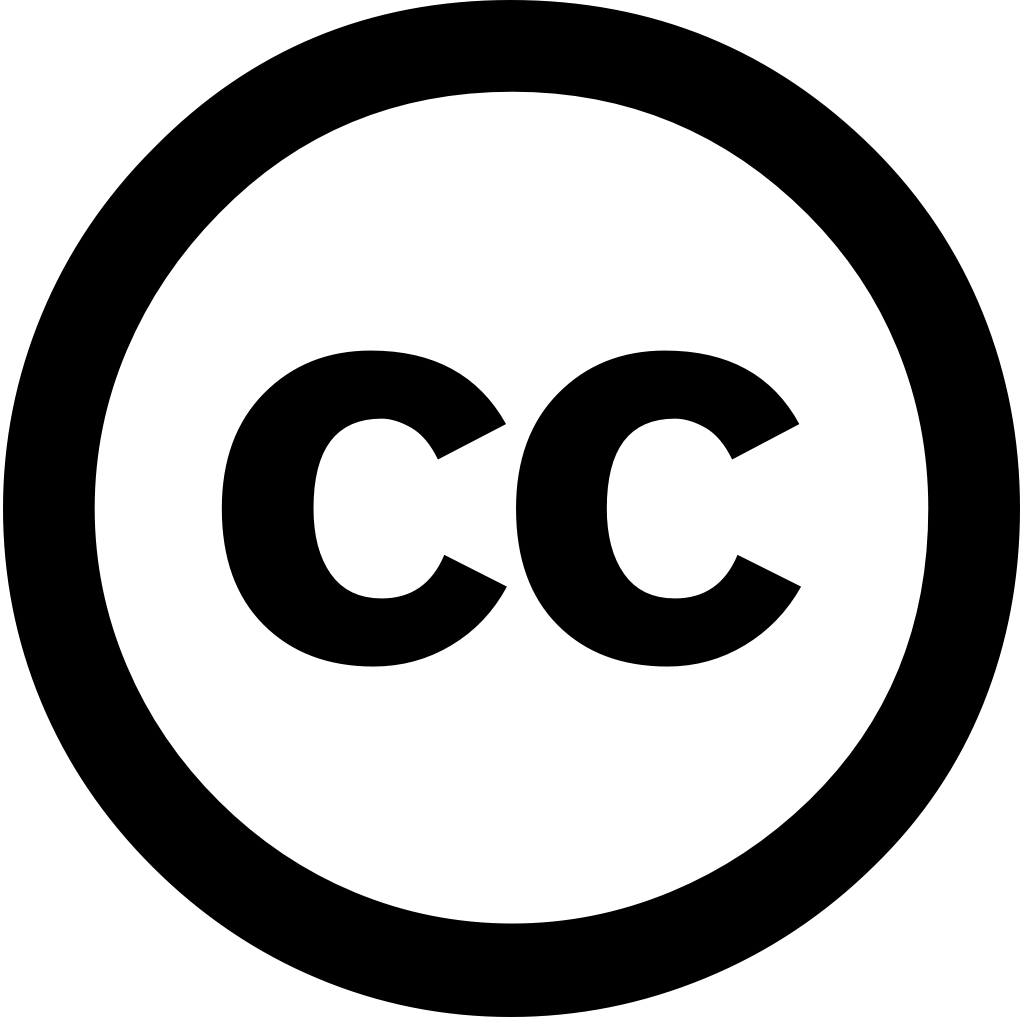
IEEE Access, Journal Year: 2024, Volume and Issue: 13, P. 32944 - 32970
Published: Nov. 18, 2024
Language: Английский
IEEE Access, Journal Year: 2024, Volume and Issue: 13, P. 32944 - 32970
Published: Nov. 18, 2024
Language: Английский
Natural Language Processing Journal, Journal Year: 2023, Volume and Issue: 6, P. 100048 - 100048
Published: Dec. 19, 2023
Large language models (LLMs) are a special class of pretrained (PLMs) obtained by scaling model size, pretraining corpus and computation. LLMs, because their large size on volumes text data, exhibit abilities which allow them to achieve remarkable performances without any task-specific training in many the natural processing tasks. The era LLMs started with OpenAI's GPT-3 model, popularity has increased exponentially after introduction like ChatGPT GPT4. We refer its successor OpenAI models, including GPT4, as family (GLLMs). With ever-rising GLLMs, especially research community, there is strong need for comprehensive survey summarizes recent progress multiple dimensions can guide community insightful future directions. start paper foundation concepts transformers, transfer learning, self-supervised models. then present brief overview GLLMs discuss various downstream tasks, specific domains languages. also data labelling augmentation robustness effectiveness evaluators, finally, conclude To summarize, this will serve good resource both academic industry people stay updated latest related GLLMs.
Language: Английский
Citations
122IEEE Transactions on Pattern Analysis and Machine Intelligence, Journal Year: 2024, Volume and Issue: 46(12), P. 10558 - 10578
Published: Aug. 21, 2024
Modern deep neural networks, particularly recent large language models, come with massive model sizes that require significant computational and storage resources. To enable the deployment of modern models on resource-constrained environments to accelerate inference time, researchers have increasingly explored pruning techniques as a popular research direction in network compression. More than three thousand papers been published from 2020 2024. However, there is dearth up-to-date comprehensive review pruning. address this issue, survey, we provide existing works taxonomy 1) universal/specific speedup, 2) when prune, 3) how 4) fusion other compression techniques. We then thorough comparative analysis eight pairs contrast settings for (e.g., unstructured/structured, one-shot/iterative, data-free/data-driven, initialized/pre-trained weights, etc.) explore several emerging topics, including vision transformers, diffusion multimodal post-training pruning, different levels supervision shed light commonalities differences methods lay foundation further method development. Finally, some valuable recommendations selecting prospect promising directions facilitate future summarize broad applications adversarial robustness, natural understanding, build curated collection datasets, evaluations applications. maintain repository https://github.com/hrcheng1066/awesome-pruning serves resource corresponding open-source codes. will keep updating include latest advancements field.
Language: Английский
Citations
48Computers and Electronics in Agriculture, Journal Year: 2024, Volume and Issue: 222, P. 109032 - 109032
Published: May 29, 2024
Language: Английский
Citations
17Industrial & Engineering Chemistry Research, Journal Year: 2025, Volume and Issue: 64(9), P. 4637 - 4668
Published: Feb. 24, 2025
This review discusses the transformative impact of convergence artificial intelligence (AI) and laboratory automation on discovery synthesis metal–organic frameworks (MOFs). MOFs, known for their tunable structures extensive applications in fields such as energy storage, drug delivery, environmental remediation, pose significant challenges due to complex processes high structural diversity. Laboratory has streamlined repetitive tasks, enabled high-throughput screening reaction conditions, accelerated optimization protocols. The integration AI, particularly Transformers large language models (LLMs), further revolutionized MOF research by analyzing massive data sets, predicting material properties, guiding experimental design. emergence self-driving laboratories (SDLs), where AI-driven decision-making is coupled with automated experimentation, represents next frontier research. While remain fully realizing potential this synergistic approach, AI heralds a new era efficiency innovation engineering materials.
Language: Английский
Citations
1IEEE Internet Computing, Journal Year: 2024, Volume and Issue: 28(5), P. 57 - 64
Published: Aug. 16, 2024
Language: Английский
Citations
8IEEE Open Journal of Vehicular Technology, Journal Year: 2024, Volume and Issue: 5, P. 1166 - 1192
Published: Jan. 1, 2024
Language: Английский
Citations
5Computers and Electronics in Agriculture, Journal Year: 2025, Volume and Issue: 231, P. 109908 - 109908
Published: Jan. 22, 2025
Language: Английский
Citations
0Neural Processing Letters, Journal Year: 2025, Volume and Issue: 57(1)
Published: Feb. 10, 2025
Language: Английский
Citations
0Nutrients, Journal Year: 2025, Volume and Issue: 17(5), P. 818 - 818
Published: Feb. 27, 2025
Background/Objectives: The increasing use of social media for sharing health and diet experiences presents new opportunities nutritional research dietary assessment. Large language models (LLMs) artificial intelligence (AI) offer innovative approaches to analyzing self-reported data from online communities. This study explores weight loss associated with the ketogenic (KD) using user-generated content Reddit, aiming identify trends potential biases in outcomes. Methods: A dataset 35,079 Reddit posts related KD was collected processed. Posts mentioning loss, duration, additional factors (age, gender, physical activity, conditions) were identified, yielding 2416 complete cases. Descriptive statistics summarized distributions adherence patterns, while linear regression examined loss. Results: median reported 10.9 kg (IQR: 4.4-22.7 kg). Diet varied 36.3% users following up 30 days 7.8% more than a year. Metabolic (27%) cardiovascular disorders (17%) most frequently conditions. Adherence beyond one year an average 28.2 (95% CI: 25.5-30.9) compared days. Male gender 5.2 3.8-6.6) females. Conclusions: Findings suggest may lead substantial based on data. highlights value research, uncovering hidden patterns that could inform public strategies personalized nutrition plans.
Language: Английский
Citations
0Applied Sciences, Journal Year: 2025, Volume and Issue: 15(3), P. 1577 - 1577
Published: Feb. 4, 2025
Restricted and Repetitive Behaviors (RRBs) are hallmark features of children with autism spectrum disorder (ASD) also one the diagnostic criteria for condition. Traditional methods RRBs assessment through manual observation limited by low efficiency uncertainty in outcomes. As a result, AI-assisted screening has emerged as promising research direction. In this study, we explore synergy visual foundation models multimodal large language (MLLMs), proposing Multi-Model Synergistic Behavior Recognition method (MS-RRBR). Based on method, developed an interpretable multi-model autonomous question-answering system. To evaluate effectiveness our approach, collected annotated Autism Dataset (ARRBD), which includes 10 ASD-related behaviors easily observable from various perspectives. Experimental results ARRBD dataset demonstrate that collaboration outperforms single-model approaches, achieving highest recognition accuracy 94.94%. The MS-RRBR leverages extensive linguistic knowledge GPT-4o to enhance zero-shot capabilities MLLM, while providing clear explanations system decisions. This approach holds promise timely, reliable, accurate technical support clinical diagnosis educational rehabilitation ASD.
Language: Английский
Citations
0