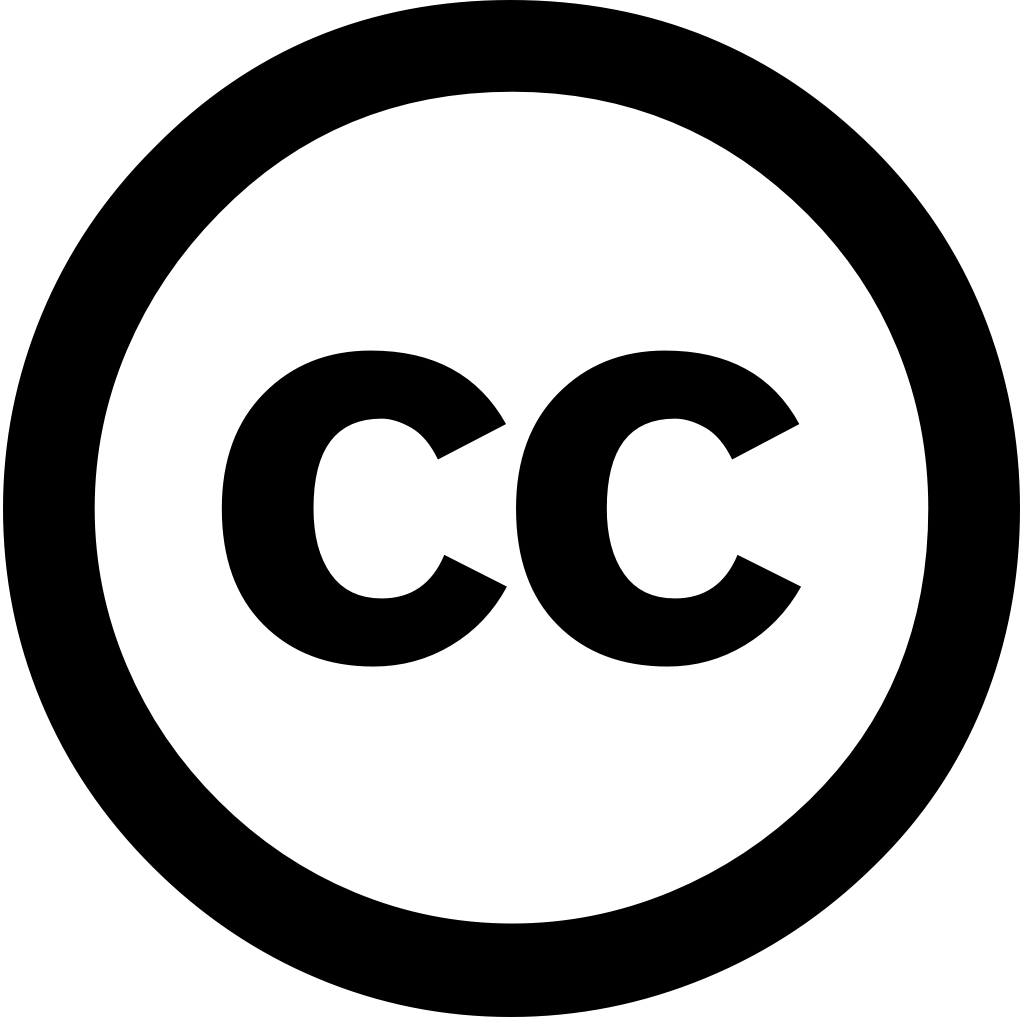
Machines, Journal Year: 2025, Volume and Issue: 13(5), P. 400 - 400
Published: May 11, 2025
Ensuring the reliability of induction motors is essential for industrial applications, as motor failures can lead to unplanned downtime and significant financial losses. Motor current signature analysis (MCSA) has emerged an effective non-intrusive technique diagnosing health, particularly monitoring bearing conditions, which account a percentage failures. However, MCSA only assess status bearings: whether they are healthy or unhealthy. Regular maintenance activities necessary avoid due failure. Furthermore, this cannot help proactively replace bearings before fail. Therefore, research develops predictive framework by integrating with machine learning techniques estimate remaining useful life (RUL) bearings. The methodology involves analyzing historical data using Isd−Isq trajectory fast Fourier transform (FFT) extract relevant health indicators. identifies deviations in behavior, whereas FFT detects harmonics that indicate potential faults. A model employed classify their RUL based on extracted signal features. This approach effectively differentiates from faulty bearings, enabling proactive reduce boost efficiency.
Language: Английский