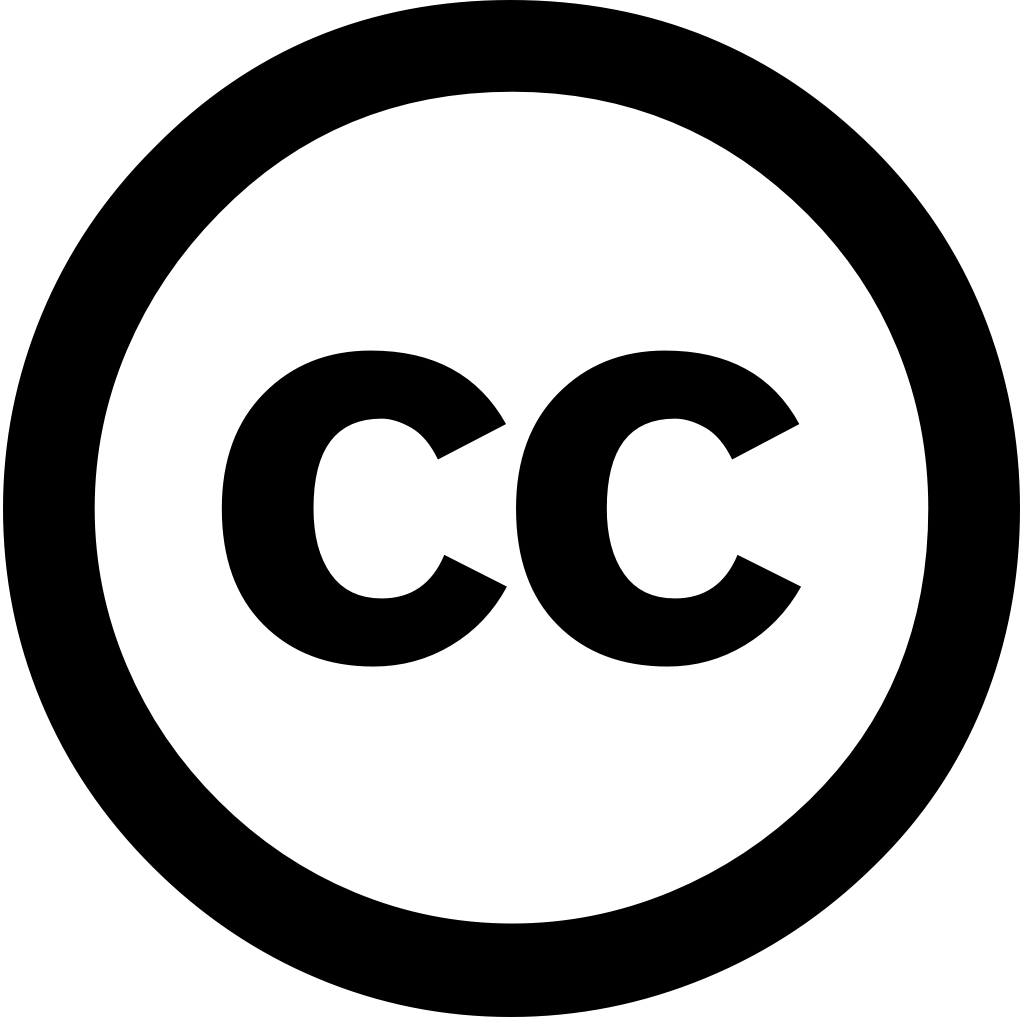
BMC Medical Imaging, Journal Year: 2025, Volume and Issue: 25(1)
Published: May 7, 2025
Language: Английский
BMC Medical Imaging, Journal Year: 2025, Volume and Issue: 25(1)
Published: May 7, 2025
Language: Английский
American Journal of Transplantation, Journal Year: 2025, Volume and Issue: unknown
Published: Feb. 1, 2025
Primary graft dysfunction (PGD) is a common complication after lung transplantation associated with poor outcomes. Although risk factors have been identified, the complex interactions between clinical variables affecting PGD are not well understood, which can complicate decisions about donor acceptance. Previously, we developed machine learning (ML) model to predict grade 3 using and recipient electronic health record (EHR) data, but it lacked granular information from CT scans, routinely assessed during offer review. In this study, used gated approach determine optimal methods for analyzing scans among patients receiving first-time, bilateral transplants at single center over 10 years. We four computer vision approaches fused best EHR data three points in ML process. A total of 160 had donor-lung analysis. The imaging-only employed 3D ResNet model, yielding median (IQR) AUROC AUPRC 0.63 (0.49 - 0.72) 0.48 (0.35 0.6), respectively. Combining imaging late fusion provided highest performance, 0.74 (0.59 0.85) 0.61 (0.47 0.72),
Language: Английский
Citations
0Journal of Agriculture and Food Research, Journal Year: 2025, Volume and Issue: unknown, P. 101948 - 101948
Published: April 1, 2025
Language: Английский
Citations
0Holistic Integrative Oncology, Journal Year: 2025, Volume and Issue: 4(1)
Published: April 8, 2025
Language: Английский
Citations
0Frontiers in Physiology, Journal Year: 2025, Volume and Issue: 16
Published: April 22, 2025
Breast cancer (BC) is a malignant neoplasm that originates in the mammary gland's cellular structures and remains one of most prevalent cancers among women, ranking second cancer-related mortality after lung cancer. Early accurate diagnosis crucial due to heterogeneous nature breast its rapid progression. However, manual detection classification are often time-consuming prone errors, necessitating development automated reliable diagnostic approaches. Recent advancements deep learning have significantly improved medical image analysis, demonstrating superior predictive performance using ultrasound images. Despite these advancements, training models from scratch can be computationally expensive data-intensive. Transfer learning, leveraging pre-trained on large-scale datasets, offers an effective solution mitigate challenges. In this study, we investigate compare multiple deep-learning for transfer learning. The evaluated architectures include modified InceptionV3, GoogLeNet, ShuffleNet, AlexNet, VGG-16, SqueezeNet. Additionally, propose neural network model integrates features InceptionV3 further enhance performance. experimental results demonstrate achieves highest accuracy 99.10%, with recall 98.90%, precision 99.00%, F1-score 98.80%, outperforming all other given datasets. achieved findings underscore potential proposed approach enhancing confirm superiority tasks.
Language: Английский
Citations
0BMC Medical Imaging, Journal Year: 2025, Volume and Issue: 25(1)
Published: May 7, 2025
Language: Английский
Citations
0