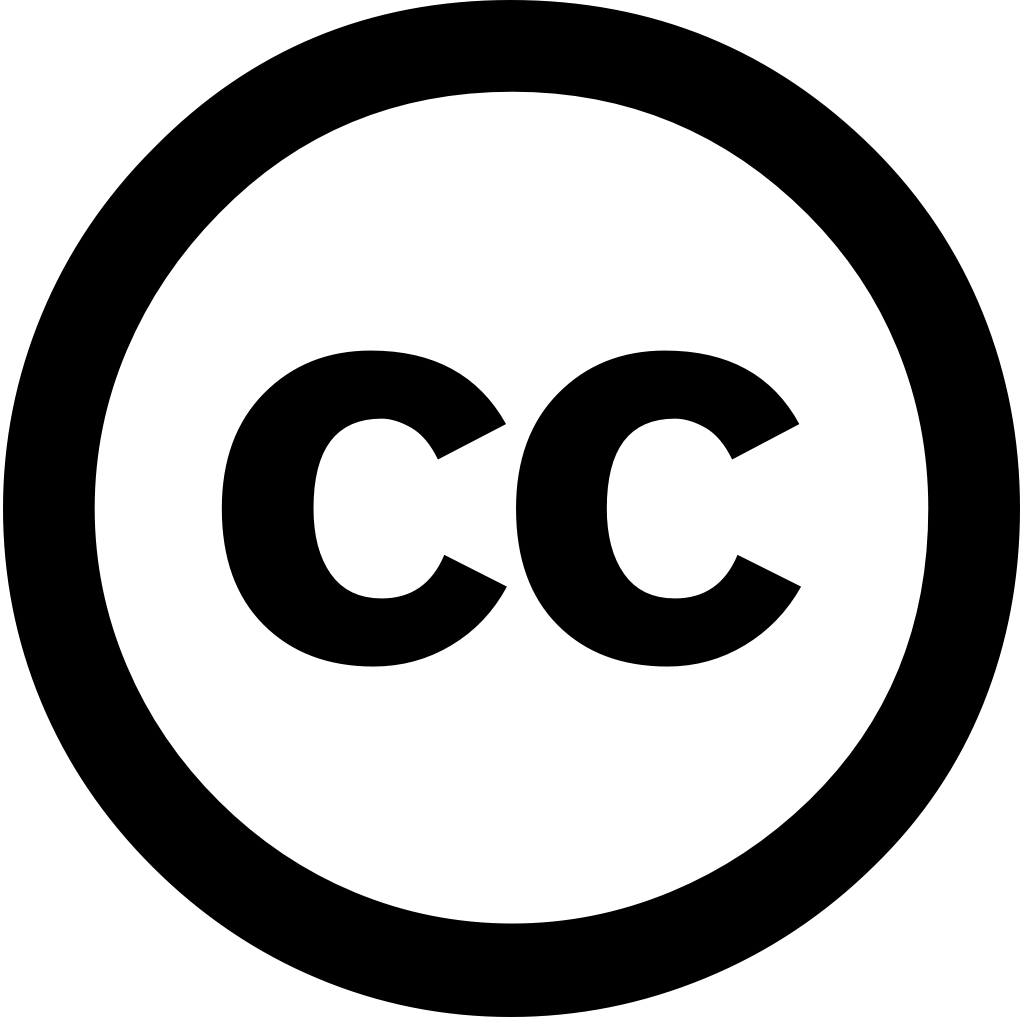
Life, Journal Year: 2025, Volume and Issue: 15(4), P. 594 - 594
Published: April 3, 2025
Objectives: Ovarian cancer continues to be one of the most prevalent gynecological cancers diagnosed. Early detection is highly critical for increasing survival chances. This research aims assess feature extraction process from various machine learning techniques better modelling ovarian and selection in analysis. By eliminating irrelevant features, this approach could guide clinicians towards more accurate results optimize diagnostic precision. Methods: study included both patients with without cancer, creating a dataset containing 50 independent variables/features. Eight algorithms: Random Forest, XGBoost, CatBoost, Decision Tree, K-Nearest Neighbors, Naive Bayes, Gradient Boosting, Support Vector Machine, were evaluated alongside four techniques: Boruta, PCA, RFE, MI. Metrics performance has been obtain best possible combination diagnosis. Results: These obtained using these methods significantly reduced number features. Forest CatBoost’s performances demonstrated significant differences contrast other algorithms (respectively, AUC 0.94% 0.95%). On hand, such as Boruta RFE consistently reflected higher accuracy scores than others. Conclusions: highlights importance choosing appropriate showed high accuracy. reducing features relevant ones, can make precise diagnoses, enhance patient outcomes, reduce unnecessary tests.
Language: Английский